This book is distributed under the terms of the Creative Commons Attribution-NonCommercial-NoDerivs License which permits noncommercial use and distribution provided the original author(s) and source are credited. (See https://creativecommons.org/licenses/by-nc-nd/4.0/
NCBI Bookshelf. A service of the National Library of Medicine, National Institutes of Health.
Structured Abstract
Background:
For patients with diabetes, hemoglobin A1c(HbA1c)treatment goals are based primarily on evaluating the outcomes and responses of the average patient. However, the American Diabetes Association and the American Association of Clinical Endocrinologists now recommend individualizing treatment regimens and HbA1c goals by including patient-centered and sociodemographic characteristics that might impact heterogeneity of treatment effect (HTE) for diabetes treatment.
Objectives:
We used pooled data from randomized controlled trials (RCTs) in diabetes and from Partners HealthCare electronic health records (EHRs) to estimate HTE and to provide performance benchmarks across clinical, sociodemographic, quality-of-life (QOL), and treatment satisfaction (SAT) patient subgroups to identify potential management strategies for improving the quality of diabetes care.
Methods:
Data from 19 previously conducted diabetes RCTs (6954 patients, 1002 clinics, 38 diabetes treatments) provided HbA1c best-practice, high benchmark probabilities (HBPs) for reaching HbA1c goals of <7.0% and <8.0%. EHRs, including those from 8107 diabetes medication-naive patients followed between 2000 and 2014, provided usual-care clinical performance probabilities (CPPs) for reaching the same HbA1c end points before any treatment modification. Linear, logistic, and survival regression models were used to estimate the effects of clinical and sociodemographic variables, such as age, sex, body mass index (BMI), baseline HbA1c, race/ethnicity, and diabetes treatments, on change in HbA1c, odds of achieving HbA1ctarget, and time to HbA1ctarget. Patient-reported QOL and SAT outcomes, HbA1c levels, and sociodemographic variables were analyzed simultaneously using latent variable structural equation modeling (SEM) to assess the impact of mediators and treatment effect modifiers on HTE. Using the estimates obtained from these statistical models, we deployed interactive web-based, online calculators on a social media learning platform and used them to facilitate the dissemination, implementation, and evaluation of the study findings.
Results
- Prototype RCT model. The results for 594 patients from 62 clinics showed that after 12 weeks on either diet and exercise (DE) plus a placebo (DE+P) or DE plus a sulfonylurea (DE+S), the overall unadjusted mean (SD) HBP estimate for reaching an HbA1c <8.0% for DE+P was 0.28 (0.29) (n = 184), and for DE+S, it was 0.67 (0.32) (n = 359). The adjusted HBP estimates for a subgroup of White men (age, 50 years; fasting plasma glucose [FPG], 150 mg/dL; diabetes duration, 1 year; BMI, 30; and baseline HbA1c, 9%) were 0.06 and 0.48 for HbA1c goals of <7.0% and <8.0%, respectively, with DE+P, compared with 0.51 and 0.94 with DE+S, respectively. For Black women of the same age and with the same FPG and duration of diabetes, but with a BMI of 36 and baseline HbA1c of 9.5%, the HBPs for HbA1c <7.0% and <8.0% were 0.04 and 0.18 with DE+P and 0.41 and 0.78 with DE+S, respectively. In the same study, mean (SD) clinic-specific HBPs for the DE+S group were 0.35 (0.18) and 0.67 (0.17) for HbA1c goals of <7.0% and <8.0%, respectively.
- Pooled RCT database. RCT models (2927 patients, 413 clinics, 18 arms) showed that improvements in QOL, SAT, and subscales of mental health and perceived health status were associated with increased odds of achieving HbA1c target goals of <7.0% and <8.0% (odds ratios [ORs] between 1.7 and 1.9 for QOL and SAT scales; all P < .05). For each SD unit improvement in overall QOL, there was a 10% increase in the odds of reaching an HbA1c goal of <8.0% (OR, 1.10; 95% CI, 1.03-1.18). Improved perceived effectiveness, symptom interference, mental health, health perceptions, cognitive performance, and functional health (all P < .02) facilitated achieving a more favorable HbA1c response. Latent variable SEM was undertaken for the full RCT sample of 5209 patients with type 1 and type 2 diabetes, 712 centers, and 32 regimens. Estimated path coefficients indicated that a 1-SD higher (better) score in baseline psychological distress corresponded to an 11% SD greater improvement in the diabetes Side Effects and Symptoms (SES) Distress Scale. In turn, a 1-SD improvement in this scale corresponded to a 15% SD decrease (better glycemic control) in HbA1c.
- Prototype EHR model (2477 patients). The CPPs of reaching HbA1ctargets of <7.0% and <8.0% before any medication change were 0.41 and 0.54 with sulfonylurea and 0.56 and 0.72 with metformin, respectively, for the Black female group described above. These CPPs could serve as benchmarks for a clinical practice to strive to meet.
- Full EHR database. EHR models for the 8107 medication-naive patients indicated that the largest variability in TEH was among the different classes of diabetes medications.
Conclusions:
Multiple RCT and EHR models confirmed the substantial variability in treatment response that is influenced by demographic, socioeconomic, and patient-reported QOL and SAT characteristics. Even in a well-controlled, highly resourced, and strict-protocol RCT setting, treatment response varies substantially. The probability of reaching HbA1c goals in benchmark practices is a measure of quality against which clinical practices can measure themselves. Moreover, potentially modifiable patient factors that drive success in achieving HbA1c goals, such as anxiety, depression, and symptom distress, can become targets for intervention to improve diabetes care.
Limitations:
Best-practice and usual-care benchmarks were based on clinical trials and the Partners HealthCare EHR data; neither is a nationally representative sample. Due to the fixed-visit-interval study design of the RCT in contrast to the EHR observational design, a direct head-to-head comparison of the 2 benchmark metrics cannot be made.
Background
Research Significance and Review of the Literature
Current HbA1c Treatment Target Goal Guidelines
In 2016, the American Diabetes Association (ADA) clinical guidelines emphasized tailoring HbA1c target goals to individual patient characteristics. Recommendations included promoting a patient-centered communication style that incorporates patient preferences, education, and cultural factors and making treatment decisions collaboratively with patients based on individual preferences, prognoses, and comorbidities.1 Several updates were subsequently made expanding on these issues, including (1) the need to reduce disparities in health outcomes2; (2) making patient-centered clinical outcomes, health status, and quality of life (QOL) key goals of diabetes self-management and education3; and (3) facilitating behavior change and well-being to improve health outcomes.4 Similarly, the glycemic control algorithm for type 2 diabetes endorsed by the American Association of Clinical Endocrinologists (AACE) and the American College of Endocrinologists (ACE) recommends that HbA1c targets be based on numerous patient-centered factors, such as age, life expectancy, comorbid conditions, duration of diabetes, risk of hypoglycemia and its associated adverse consequences, patient motivation, and adherence, in addition to other psychosocial determinants.5,6 In primary care, person-centered outcomes–driven treatment for type 2 diabetes is increasing, reflecting a paradigm shift away from focusing primarily on lowering HbA1c and more toward tailoring treatment to each patient’s comorbidities, characteristics, and preferences in addition to their level of glycemic control.7
The most recent ADA 2020 guideline recommends that an appropriate glycemic target for nonpregnant adults is <7.0% HbA1c (53 mmol/mol).8 However, based on provider judgment and patient preference, more stringent HbA1c goals, such as 6.5% (48 mmol/mol), for selected individuals at low risk for hypoglycemia and other adverse effects may be acceptable. A less stringent HbA1c goal of <8.0% (64 mmol/mol) also may be appropriate for patients with a history of severe hypoglycemia, limited life expectancy, advanced microvascular or macrovascular complications, extensive comorbid conditions, or difficult-to-treat longstanding diabetes. AACE and ACE generally recommend an HbA1c target of <6.5%, with similar caveats for more and less stringent targets depending on the individual’s risk factors and characteristics. The ADA and AACE/ACE consensus reports and algorithms are built on systematic and critical reviews of the literature reporting results from randomized controlled trials (RCTs), meta-analyses, prospective cohort studies, registries, and case-control studies. Information also is collected using expert panels, group consensus methods, and clinical experience. These consensus reports influence not only clinical decisions but also standards of quality of care and clinical performance in diabetes programs such as the Diabetes Recognition Program supported by the National Committee for Quality Assurance9 and Pay for Performance.10,11 The Centers for Medicare & Medicaid Services currently requires health plans to report performance using the Healthcare Effectiveness Data and Information Set (HEDIS) measures that allow comparisons between health care systems.12 However, the HbA1c HEDIS measures define poor control generally for all individuals with either type 1 or type 2 diabetes as HbA1c >9.0% and adequate control as HbA1c <8.0%, irrespective of any other patient characteristics.
Improving the Quality of Diabetes Care by Benchmarking Performance
The complexity of translating qualitative recommendations for personalizing HbA1c target goals into diabetes management practice contributes to clinical inertia among physicians for modifying treatment regimens based on customized profiles. This inertia results in using 1 generic HbA1c target for all individuals, except for those who fall clearly into the lowest- and highest-risk categories. Rodbard et al13 released an AACE/ACE algorithm for achieving glycemic control targets using decision paths to describe optimal treatment strategies based on categorizing HbA1c levels. However, quantitative models for HbA1c goals that incorporate more than beginning HbA1c levels do not exist. Furthermore, feedback to physicians and patients on how well they are doing relative to those patients with similar characteristics, and compared with physicians and systems representative of either “best practice” or “usual practice,” is also necessary to evaluate performance in a valid manner.
One method to improve and motivate clinical decision-making is through a quality control process known as “benchmarking.” External benchmarking involves measuring performance against best practice, whereas competitive or comparative benchmarking measures performance relative to how others with similar resources, skills, and abilities perform. One of the first applications of benchmarking to improve clinical performance was in the prevention of hyperbilirubinemia in newborns.14 Since that time, benchmarking for quality-of-care evaluation within hospitals and health care organizations has become more common. For example, a prospective RCT of type 2 diabetes demonstrated that benchmarking was a useful tool for increasing the achievement of critical quality indicators and possibly reducing patient cardiovascular residual-risk profile.15 Another study in Italy involving a network of 32 diabetes clinics in 4 different regions field tested benchmarking activities focusing on clinical and patient-centered indicators to reflect different levels of performance in terms of process and intermediate health outcome measures.16 The same benchmarking network also showed the association between diabetes-related distress and worse clinical and psychosocial outcomes, thus recommending that high levels of diabetes-related distress should be considered a critical patient-centered indicator.17 More recently, benchmarking has been used to explore variations in HbA1c across and within 8 high-income countries to best inform international benchmarking and policy recommendations18 and to describe the clinical status of people with diabetes attending specialist diabetes services in Australia.19 Another study demonstrated that the health of patients with diabetes was associated with sociodemographic factors such as age, sex, disease history, educational level, marital status, and region of birth, and that these were important factors to consider when benchmarking HbA1c to evaluate the level of health equity between organizations or between different administrative regions.20
Despite some recent advances in benchmarking research, quantitative benchmarking approaches to HbA1c target goals adjusted by personal characteristics is very uncommon. The application of quantitative methods in comparative benchmarking is also infrequent. Furthermore, in addition to baseline sociodemographic and clinical characteristics, the role of behavioral and psychological factors regarding heterogeneity of treatment effect (HTE) has not been adequately studied. Knowing whether individuals of comparable age, sex, education, duration of diabetes, and baseline HbA1c have more difficulty reaching their target goal if they have a diagnosis of depression or depressive symptoms could inform treatment decisions.
Although many treatment alternatives are available for individuals with type 2 diabetes, a substantial number of patients are still unable to achieve the ADA and AACE/ACE glycemic target goals. Benchmarking reference standards need to consider the diabetes therapy used when determining which patient characteristics should be included for an individual patient. A systematic review supported metformin as first-line therapy in type 2 diabetes, given its beneficial effects on HbA1c, weight, and cardiovascular mortality relative to sulfonylureas, as well as its overall safety profile.21,22 The review concluded that comparative outcomes of medication classes could “facilitate personalized treatment choices for clinicians and their patients” and that future research is needed to focus on diverse populations to investigate subgroup differences due to demographic and psychosocial factors. In addition to the inability of drug therapies to remain effective over time for specific subgroups of patients, issues with health care access, behavioral factors relating to clinical inertia, and patient characteristics also contribute to HTE.23-25 As such, they should be considered in setting benchmarking standards.
Role of Benchmarking in Personalized Medicine
Evidence regarding the HTE is necessary for calculating benchmarks as part of personalized or precision medicine. These terms refer to the “tailoring of medical treatment to the individual characteristics, needs, and preferences of each patient during all stages of care, including prevention, diagnosis, treatment, and follow-up.”26 Whereas personalized medicine has focused primarily on how genetic27 and genomics28,29 data impact treatment response, precision medicine also uses demographic, psychosocial, nutritional, physical activity,30 and socioeconomic data.31 The variability in the prevalence of diabetes across counties in the United States suggests that even regional differences, most likely reflecting patient socioeconomic, cultural, and behavioral factors, could play a role in the variability in treatment response.32 A review of 4 major clinical trials for patients with type 2 diabetes concluded that glycemic targets should be individualized not only according to the clinical context, but also to the psychological, sociological, and cultural characteristics specific to each patient.33 Using National Health and Nutrition Examination Survey 2007-2008 data to individualize glycemic targets, it was determined that approximately half of adults with diabetes in the United States would have recommended HbA1c targets of ≥7.0%. However, one-third of all adult patients with diabetes would still be inadequately controlled.34 The study recommended that performance measurement goals should be revised to encourage individualization of glycemic targets. The review also concluded that although evidence regarding the effects of health status, personality, and behavior on HTE in diabetes is needed for personalized care, scientific studies supplying the required evidence are rare.
Challenge of Gathering the Evidence for Benchmarking Analysis in Diabetes
In a 2010 systematic review of the evidence explaining HTE in type 2 diabetes, only 43 articles were identified. Of these, 31 (72%) described sociodemographic factors, and 17 (40%) reported comorbidity or behavioral factors.35 Few studies included patient-reported outcome (PRO) measures. Some metrics have been developed to identify subgroups of patients with differential potential for response to treatment36,37 as well as to investigate racial and ethnic disparities.38 However, few clinical trials compare multiple diabetes medications for periods longer than 6 to 12 months, and even fewer report subgroup treatment responses.
One currently ongoing clinical trial, the Glycemia Reduction Approaches in Diabetes: A Comparative Effectiveness (GRADE) Study, is following 5047 patients with type 2 diabetes treated with metformin alone for 4 to 7 years. The trial compares the addition of either a sulfonylurea, dipeptidyl peptidase-4 (DPP-4) inhibitor, glucagon-like peptide-1 (GLP-1) analog, or insulin to a metformin regimen. However, the study results will not be available until after the end of the study period in April 2021.39,40 In this study, patient-reported QOL and treatment satisfaction (SAT) are measured using the Short Form-36 (SF-36) and Quality of Well Being (QWB) questionnaires administered at baseline and the end of each year. The Diabetes Treatment Satisfaction Questionnaire (DTSQ) is administered at baseline, 6 months, and 1 year. However, the SF-36 is a generic, discriminant assessment instrument,41 and the QWB questionnaire is a preference-weighted utility measure.42 Neither instrument is designed to detect the more immediate, diabetes-specific psychological concerns, diabetes-related distress, and behavioral issues that affect the ability of patients to reach their HbA1c goals. The DTSQ43 consists of 8 items and was not originally developed as an evaluative measure, although a “change” module was subsequently added to reduce the impact of the ceiling effects observed with the original version.44
Study Goals, Primary Research Question, and Specific Aims
Health outcome differences associated with diabetes and its complications are related to a complex set of issues that include demographic, social, psychological, and economic factors. Because lifestyle-related behaviors such as diet and exercise (DE) and adherence to diabetes medications are primarily under the patient’s control, research yielding information on the effectiveness of diabetes prevention and treatment in terms of patient-centered outcomes is warranted. Furthermore, studies that inform patients, caregivers, and clinicians concerning how these patient characteristics can influence and modify the effects of treatment on patient-centered and clinical outcomes have not been addressed using comparative effectiveness research (CER). Although there are extensive and comprehensive guidelines for the treatment of diabetes, there remain gaps in the translation of CER evidence into clinical implementation and practice. We designed this project to address some of these gaps. The goal, primary research question, and specific aims of our study are described below.
- Study goal. This study will examine the influence of PROs and sociodemographic factors that might influence the comparative effectiveness of various treatments for diabetes.
- Primary research question. How do functional, psychological, social, economic, and demographic factors influence and modify diabetes treatment effectiveness?
- Specific aims. Our specific aims describe (1) treatment-related differences in patient-centered QOL and satisfaction outcomes; (2) the extent to which these differences impact glycemic control; and (3) how the effects of these differences on glycemic control are modified by demographic, socioeconomic, and psychosocial characteristics. To achieve these aims, we constructed evidence-based explanatory equations using the estimated parameters from regression models to develop a best clinical practice benchmark to aid clinicians and patients in personalizing and targeting modifiable patient-centered mediators of treatment effectiveness. Although the data are largely from adults with type 2 diabetes, type 1 diabetes data are also included in some models.
In summary, our primary research objective is to provide quality-of-care evidence to compare clinical practice performance for achieving HbA1c goals in individuals with diabetes with a high quality-of-care benchmark that can be adjusted with regard to the demographic, socioeconomic, and patient-centered characteristics of the clinical population subgroups being treated. A secondary objective of our study is to provide evidence that identifies patient-reported and sociodemographic factors that could be used for adjusting glycemic targets within various therapeutic classes of treatment.
Participation of Patients and Other Stakeholders
Our research program was structured to foster engagement and communication to ensure that key stakeholders and partners were involved in the process and to identify potential facilitators and barriers to dissemination and translation in clinical practice. Translating, communicating, and disseminating results in educational programs and practice tool kits were emphasized.45,46 The results from our research from this project have been presented annually at the ADA Scientific Sessions. In 2014, we introduced the RCT initial prototype “high benchmark” model,47 and in 2015, we presented the EHR initial prototype “clinical practice benchmark” model.48 During the 2015 meeting, we also presented the results of a survey of internet use that might impact our diabetes social media data sources,49 and we gave an oral presentation describing the social media crowdsourcing method.50 In 2016, we reported on patient-centered outcomes and sociodemographic factors of diabetes treatment effectiveness using the EHR database.51 We also reported the results from an RCT database analysis identifying the impact of subclinical depression and anxiety on the intensity and frequency of treatment-related adverse effects and symptom distress that affect treatment effectiveness.52 During the same meeting, we presented 2 EHR studies that were related to earlier EHR work.53,54 Other presentations in 2017 included a study describing the benchmarking calculator tool and the engagement and dissemination method to foster implementation55 using a social media learning collaborative. The results of a benchmarking study of the impact of patient-centered factors on the comparative effectiveness of initial therapies in treatment-naive individuals with type 2 diabetes were reported in 2018.56 In 2019, we reported the results of an analysis that modeled pathways between psychological distress, emergent diabetes adverse effects, and symptom distress during treatment and end point HbA1c.57 During these 6 annual ADA Scientific Sessions, we received feedback from advisory committee members and from the general attendees during poster sessions and oral presentations, and by hosting an informational and educational booth in the exhibit hall. The reach was substantial, with >15 000 individuals attending the ADA meetings each year.
In our original PCORI proposal, we stated,
Our goal for dissemination of the products of this research (monographs, journal publications, web-based and predictive benchmarking tools) is to obtain input and feedback using face-to-face meetings, interview focus groups, video broadcasts, and social media technologies, including collecting crowdsourced data from the diabetes social networks.
Based on our prior research using social networking engagement methods in diabetes,50 we also relied on web-based technologies to foster engagement. Appendix 1 contains screenshots from our websites. We collected data from online diabetes communities and built a Web 1.0 project website (https://www.hsph.harvard.edu/comparative-effectiveness-of-diabetes-treatments/) which contains static information for our target audiences. We also developed an interactive, collaborative Web 2.0 website entitled “Diabetes Stakeholders and Partners Forum” at https://phasevtech.net/pcori/ to disseminate, evaluate, improve, and sustain our work product after the end of the project period. All project-related meeting abstracts and links to posters and presentations are accessible to individuals who request membership to the password-protected forum. We posted information about future spin-off projects and publications there. We designed the Web 2.0 website to allow users to interact and collaborate in a social media dialogue as creators of user-generated content in a virtual community, in contrast to the Web 1.0 website, where visitors are limited to passive viewing of content. The hsph.harvard.edu domain website served as a feeder to the materials available on the Web 2.0 website. Opinion leaders also communicated findings through meetings and video clips (see sample video at https://youtu.be/XlL6Cbgidk4 [McConnell58] and Appendix 1 exhibits).
During the project, we obtained input and feedback from our partners and stakeholders using face-to-face meetings, webinars, and social media technologies, including collecting crowdsourced data from online diabetes social networks. To accurately identify individuals who could facilitate dissemination and implementation, we used professional membership directories, health care institutions, and industry registries. Our 5-member steering committee consisting of the co–principal investigators, co-investigators, chairs of the Stakeholder and Partner Forum (S&PF), and the online S&PF (eS&PF) liaison provided the highest level of leadership and governance. The S&PF also included the advisory committee (12 members), subcommittee of the diabetes social networking eS&PF, and the broader membership constituency made up mainly of the eS&PF members. We discussed barriers and potential solutions among research team members and with other S&PF advisory members to identify strategies to facilitate the future dissemination and uptake of knowledge.
Given that our study used existing data, changing or modifying data collection methods or settings based on the feedback received was not possible. However, translation of the science into health care education and practice through the implementation of the benchmarking tools was guided by the direction of health care stakeholders and patients. Our primary goal for their feedback was to enhance dissemination efforts to foster rapid translation of results to clinical care and policy decisions. To this end, we used the fundamentals of implementation science to promote the integration of research findings and evidence into health care policy and practice.
Methods
Study Overview and Settings
We used patient-level data from prior RCTs and electronic health records (EHRs) to investigate the impact of patient characteristics on achieving HbA1c goals when either randomly assigning patients to RCTs or prescribing usual-care diabetes treatments (as demonstrated in the EHRs). Surveys and crowdsourced data were used to plan for and guide future dissemination and implementation of the results of the study. As depicted in Figure 1, three independent data sources allowed us to triangulate and cross-validate our findings, conclusions, and interpretations using structured quantitative data and unstructured qualitative data. As explained in more detail below, we used RCTs to obtain the high clinical performance benchmarks for achieving HbA1c treatment goals because by design, RCTs ensure rigorous adherence to a strict study protocol, fully funded resources, and uniform health care delivery and access regardless of ability to pay. Moreover, due to the high degree of homogeneity in health care delivery and access afforded by an RCT, the observed HTE within treatments should be attributed primarily to the following types of patient characteristics: physiological; clinical; demographic; socioeconomic; behavioral; and physical, psychological, cognitive, social, and role functioning. Taking these points into consideration, the pooled RCT data design provides a way to estimate a high quality-of-care benchmark that can be adjusted depending on individual patient characteristics in the clinical practice. This application of what is commonly referred to as “case-mix adjustment” permits the comparison of treatment outcomes between a reference practice benchmark such as high quality, average quality, or poor quality, and a practice with differing mixes of patients with regard to diagnoses, severity of illness, and other variables associated with the probability of improvement with treatment using statistical procedures. Furthermore, by pooling data from many RCTs, we can more precisely estimate the magnitude of differences among patients.

Figure 1
Triangulated, Pooled Data From RCTs and EHRs, and Qualitative Data From the SPF and a Social Media Patient Website.
The EHR observational study design allows us to assess whether the contributions of these patient-centered variables to differences in treatment response can be detected in real-world practice settings where there are many other external factors contributing to HTE, such as health care access, ability to pay, and physician practice differences. We hypothesized that although heterogeneity would be present in the EHR setting, attributing it to particular patient characteristics would be more difficult. The EHR data were also used to assess the availability of the patient data that would be needed to perform the adjustment necessary for a clinical practice to compare their performance with that of the high-quality benchmark.
We structured our model development and analysis to coincide with the building of the pooled RCT database and the EHR database. While the first phase of the pooled RCT database was being constructed, we undertook an analysis of a prototype benchmarking model using data from a single, double-blind, placebo-controlled clinical trial. This prototype RCT model (N = 594) was used to determine the potential degree of HTE among HbA1c changes in groups of patients undergoing treatment with DE plus a placebo (DE+P) and DE plus a sulfonylurea (DE+S). After construction of the pooled RCT database, a sample of 5686 individuals with type 1 and type 2 diabetes was available for analysis and allowed us to make an estimation of HTE across several different diabetes treatments.
In this study, a benchmark provides an estimated probability of reaching a prespecified target (eg, HbA1c <7.0%), accounting for those factors that contribute to the variability observed among HbA1c outcomes using retrospective data from either clinical trials or EHRs. Our study pooled data from 19 RCTs that represent best practices or high benchmarks because RCTs provide substantially more health care resources and detailed protocols than clinical practice does. The protocols are followed by clinical investigators and monitored rigorously to achieve the best possible HbA1c outcome. We used the EHR database to obtain a pragmatic comparative clinical performance benchmark as a reference to demonstrate how physicians might compare with usual, real-world conditions, and how patients might respond in terms of treatment effectiveness variability in a clinical practice setting.
Participants
Pooled RCT Data
To develop the best-practice or high benchmarks, we used individual-level, deidentified data from therapeutic clinical trials of individuals with type 1 or type 2 diabetes. We used RCTs for which we had served as the original investigators responsible for designing, collecting, managing, and analyzing the demographic, socioeconomic, and standardized QOL, SAT, and other clinical variables (eg, diabetes duration, treatment assignment, HbA1c levels, fasting plasma glucose [FPG]) in a comprehensive, uniform, and consistent manner. These uniform criteria allowed for valid data pooling, and the data were made available through a data use agreement.59 All clinical trials were comparative efficacy trials conducted between 1990 and 2010 as Phase 3, Phase 4, or postmarketing studies, according to the FDA’s guidance. The trials included 12 to 52 weeks of active diabetes treatment. The 19 RCT studies collected standardized, patient-reported questionnaires that previously demonstrated sensitivity for detecting between-group changes in HbA1c levels and changes in QOL and SAT of at least 0.3 responsiveness standard error of measurement units or higher. These performance standards had been demonstrated in the RCTs including DE vs sulfonylurea,60 insulin glargine plus insulin glulisine vs premix insulin,61 and human GLP-1 analogue liraglutide vs glimepiride monotherapy.62 In total, 19 clinical trials met these design eligibility criteria, as shown in Table 1a.

Table 1a
Diabetes Pooled RCTs: Interventions, Sample Sizes, and Published References.
Due to the heterogeneity of the database structure among studies, a template for the pooled database was developed initially and a set of guidelines followed to abstract data from the available RCT source files into a pooled database of common core variables. Because these 19 studies were the only ones that qualified for inclusion in the pooled RCT database, they comprised the population of all such studies and were internally valid to represent this population.
EHR Data
To develop the usual-care, clinical practice benchmark references, we obtained EHRs from Partners HealthCare, an integrated health care delivery network in eastern Massachusetts founded by Brigham and Women’s Hospital (BWH) and Massachusetts General Hospital (MGH). The network consists of specialty and community hospitals and affiliated outpatient practices. Most outpatient clinicians at Partners HealthCare used the Longitudinal Medical Record (LMR), an internally developed EHR certified by the Office of the National Coordinator–Authorized Testing and Certification Body (meaningful use).84,85 The LMR includes a fully functional medication module that allows structural entry of the medication name, route, dose, strength and form, frequency, as needed, the reason, amount to dispense, and the number of refills. The composition of practice setting and physician specialties of LMR users did not change significantly over the study period. There were no significant changes in LMR user training methodologies throughout the study period. We studied adult patients with diabetes followed by primary care physicians (PCPs) affiliated with BWH and MGH, between August 1, 2000, and June 30, 2014. Patients were included in the analysis if they fulfilled all the following criteria: (1) were at least 18 years old; (2) had documented evidence of diabetes (“diabetes” on the EHR problem list, HbA1c of at least 7.0%, or at least 3 ICD-9 codes of diabetes mellitus); and (3) were followed for their diabetes for at least 12 months during the study period.
The database construction involved 2 phases, an early and late phase. The EHR early prototype model, described later in the “Statistical Methods” section, was developed using the early phase of BWH EHR data abstraction (N = 80 677 patients with diabetes through 2010) completed during the first 12 months of the study. Subsequent EHR models were developed using the expanded Partners HealthCare EHR database (N = 233 627) of patients with diabetes through 2014 completed after 18 months into the project. We developed many more variables for the analysis for the second phase. Given that it took project development time to use natural language-processing algorithms to extract data from the clinical record, variables that appear in the second phase of the EHR database regression models, such as estimated glomerular filtration rate (eGFR) and hypertension, were not available for analysis during the first phase. From these larger databases, we selected patients who met the following inclusion criteria: (1) new diabetes medication users; (2) service providers from either BWH or MGH who had laboratory test results available; (3) new diabetes medication starting date on or after January 1, 2000; (4) medical record notes available within the study dates; (5) at least 2 notes in a primary care clinic at least 1 year apart; and (6) age ≥18 years and HbA1c A1c >7.0% before the start of first-time diabetes medication therapy. Consequently, at the start of the observation period, patients had not been treated with diabetes medication, and their HbA1c was >7.0%.
Social Media and Survey Data
To assess the interpretability of the RCT and EHR quantitative analyses, we evaluated crowdsourced data consisting of anonymous publicly available online posts. The purpose of the social media qualitative analysis was to determine whether spontaneous, unsolicited reports from patients paralleled the structured assessments in terms of content, coverage, and direction of effects. For example, if total symptom distress during treatment is shown to be more strongly associated with lower HbA1c effectiveness while a patient is taking 1 drug but not another, we sought to explore potential reasons based on information from direct quotes from patients. The posting authors were from various online diabetes websites and were not identifiable. In addition to the crowdsourced data, we used the publicly accessible, structured, Pew Health Tracking Survey databases86,87 toevaluate potential information bias of the social media crowdsourced data.
Interventions and Comparators
Pooled RCT Data
The primary goal of this study was to examine how patient-reported baseline characteristics and treatment-emergent patient-centered outcomes and sociodemographic factors might influence the comparative effectiveness of various treatments for diabetes and to determine which diabetes treatments were uniformly more effective. We compared HbA1c levels at the end of treatment among subgroups of patients defined by the levels of PROs at baseline and in response to treatment, sociodemographic factors at baseline, and different diabetes treatments. Table 1a summarizes the diabetes treatment interventions by drug class, sample sizes, and baseline HbA1c or FPG level within each of the 19 RCTs used for this study. Table 1b provides further detail on the type of diabetes, treatment arms (collapsed to 38 arms), completion rates, and clinical site locations. These comparative trials included different insulin formulations and combinations (eg, regular, neutral protamine Hagedorn (NPH), premix, ultralente) and delivery systems (eg, subcutaneous syringe injection, pen injection, continuous subcutaneous insulin infusion, pulmonary inhaler). For analytic purposes, we grouped insulins by delivery system because the different types, doses, and number of injections or inhalations were already fixed and nested within the study. Oral agent (OA) treatments included sulfonylureas, thiazolidinediones, biguanides, meglitinides, and α-glucosidase inhibitors, in addition to an injectable glucagon-like peptide-1 (GLP-1) and diabetes management programs, such as DE only (some with placebo), usual care, and staged diabetes management.88 Medications such as the sodium-glucose cotransporter-2 (SGLT2) inhibitors approved during 2013 and 2014 (eg, canagliflozin and dapagliflozin) were not available for analysis because there were no clinical trials that included the required patient-centered outcome variables at the time this study was designed in 2013. Since this study ended, we have added 2 large clinical trials to the RCT database, increasing the pooled total sample size by >1000 patients with type 2 diabetes and expanding the treatment categories to include combination therapy with SGLT2 (dapagliflozin) plus DPP-4 (saxagliptin) plus metformin without sulfonylurea (n = 324) vs insulin glargine plus metformin without sulfonylurea (n = 319),89 and SGLT2 plus DPP-4 plus metformin (n = 227) vs glimepiride plus metformin (n = 216).90 For data available at the time of this analysis, selected baseline clinical, demographic, socioeconomic, and PRO descriptive statistics are shown for the largest subgroup of adults with type 2 diabetes (Table 1c).

Table 1b
Health Outcomes Comparative Effectiveness Diabetes Pooled RCTs: Detailed Information.

Table 1c
Adults (N = 6151) With Type 2 Diabetes: Baseline Characteristics in Pooled RCT Database (Common Core Variables).
EHR and Social Media Data
The interventions and comparators for the EHR data were based on sociodemographic and diabetes treatment variables that were similar to those used in the RCTs. Because no structured PROs were available in the medical record, these variables were not available as part of the EHR database. Crowdsourced data were analyzed to gain further insight into the impact of diabetes therapies on patient QOL for assessing the interpretability of the RCT and EHR quantitative analyses. These analyses did not involve interventions or hypothesis testing.
Study Outcomes
To facilitate the development of the benchmarking analyses and algorithms, we defined the following terms and definitions for the RCT and EHR analyses:
- HbA1c target outcomes: HbA1c <7.0% and HbA1c <8.0%.
- High-benchmark probability (HBP): The probability that a patient meets the HbA1c target outcome within a level of clinical care and patient management that provides a highly intensive, resourced, and well-managed best-practice reference as typically described in clinical practice guidelines published by professional organizations. In this study, the pooled RCT database was used to estimate the high benchmark reference.
- Clinical performance probability (CPP): The probability that a patient meets the HbA1c target outcome in a defined clinical setting. In this study, the BWH and Partners HealthCare databases were used to provide clinical performance benchmarks.
- Patient-centered variables: Clinical, demographic, and socioeconomic patient characteristics in addition to the patient-reported QOL and SAT outcomes at the start of the exposure and during the exposure period as applicable and used to adjust HBP and CPP for personalizing patient target goals.
- PROs: Subjective information generated directly by a patient through interviews, self-completed questionnaires, or other capture tools, such as diaries about his or her life, health condition(s), and treatment as viewed from the patient’s perspective, including perceptions of health status, symptoms, adverse effects of treatment, QOL, and SAT.
Variables in the Pooled RCT Database
The pooled RCT database included a battery of evaluative scales consisting of more than 170 items measuring perceived health, functional health status, diabetes-specific symptom interference, general symptom interference, concerns and distress about weight and body image, distress related to symptoms and adverse effects, mental and emotional health, general health perceptions, perceived cognitive functioning, sexual satisfaction and dysfunction, health care use, work absences, and disability.91 In many trials, diabetes SAT evaluative scales also were employed. These scales consisted of a core module applicable to all therapies, including DE, OAs, and insulin, with 71 items and 13 scales (overall satisfaction scale with its subscales of efficacy, flexibility, adverse effects, convenience, burden, preference, advocacy, social, general satisfaction, pain, hassle, and interference). Additional insulin delivery (6-12 items) and comparative delivery (13 items) modules were included as applicable. For all the RCTs, 2 authors (M.A.T. and D.C.S.) were the original investigators responsible for the data collection, management, and analysis of the demographic, socioeconomic, and patient-reported assessments of QOL and SAT. As such, they had access to these databases. The clinical data on HbA1c were collected by the original RCT study sponsor and shared with the authors as part of the analysis of these trials.
A more detailed description of the demographic, patient-reported, and clinical outcomes can be found in Appendix 2. Briefly, demographic variables included age, sex, race, education, employment status and occupation, income, and disabilities. Self-administered questionnaire assessments were made longitudinally at baseline and at the end of the study, as well as at several study visits in between and at early withdrawal, with scheduled follow-up periods ranging from 12 to 52 weeks. The PRO measures were based on standardized and validated scales that had been found to change in response to the effects of pharmacologic interventions,92-94 the impact of adverse effects of medication, symptoms of diabetes,95,96 and changes in HbA1c.97-99 The HbA1c data were available for all studies, and several studies also included data on FPG, adverse events, hypoglycemia, laboratory assessments, blood pressure, heart rate, weight, and height. All study protocols were cross-referenced with publications, FDA New Drug Application approval documents, and summaries on ClinicalTrials.gov, as applicable. As shown in Table 1a, of the 6954 unique study participants randomly assigned to treatment and reported in the study records, 6896 (99.2%) had sufficient nonmissing records and could be fully reconciled in the RCT database.
Variables in the EHRs
To abstract information from the narrative medical note files, we used natural language processing to construct algorithms.100 Structured data were abstracted directly. A patient entered the study on the last of the following dates: (a) first primary care note; (b) 18th birthday; (c) first documented evidence of diabetes; (d) first record of a diabetes medication; or (e) January 1, 2000. Demographic variables of age, sex, and zip code for geocoding of residential neighborhood income levels, as well as clinical variables, including HbA1c and current medications, were abstracted and exported into relational databases for analysis. Patients were followed longitudinally to assess changes in HbA1c levels and diabetes therapeutic regimens. We observed the medication-naive patient for the entire time period they were being seen by their health care provider until they reached the end point or until they had a change in medication; if they did not reach an end point or have a change in medication, they were followed until they were no longer under the care of their health care provider. If a patient did not reach an end point, or if they switched medication or ended care/left their provider, we either used the last HbA1c before switching or adding to their medication or used ending care/leaving their provider as the HbA1c study end point.
There were 2 dichotomous HbA1c target end-point variables: HbA1c <7.0% and <8.0%. The dichotomous variables were coded such that 0 indicated a failure to reach the goal (eg, HbA1c ≥8.0%), and 1 indicated success in reaching the goal (eg, HbA1c<8.0%). For example, if the last HbA1c value before switching medication was 6.9%, we coded these dichotomous variables as HbA1c <7.0% = 1 and HbA1c <8.0% = 1. If their last value was 7.2% before switching, we coded the variables as HbA1c <7.0% = 0 and HbA1c <8.0% = 1.
Social Media and Online Survey Analyses
As described previously in the “Participation of Patients and Other Stakeholders” section, to gather feedback directly from patients with diabetes, we gathered publicly available unstructured data from online diabetes forums (eg, https://community.diabetes.org/discuss), with the patient “author” as the primary sampling unit. Qualitative text analyses were used to abstract sentiments, feelings, medication adverse effects, health status, HbA1c, weight, fasting blood glucose (FBG) at baseline and follow-up, medications and medication history, membership level (eg, responder, contributor, advisory), and type of diabetes. Pew Health Tracking Survey databases were used as previously referenced.
Sample Size Calculations and Power
Continuous HbA1c Outcome Variable (Both RCT and EHR Databases)
The total pooled RCT sample of 6518 patients with diabetes with nonmissing HbA1c treatment-related changes, demographic, socioeconomic, clinical, and PRO data yielded an overall mean (SD) baseline-to–end point change in HbA1c of 0.85% (1.4%). On average, 2-sample unadjusted treatment-related HbA1c changes of 0.5% (a small-to-medium standardized effect size of 0.36 SD) would be detectable given a 2-sided test, α of .05, and power of 0.9, with comparison sample sizes of 166 per group. Medium effect sizes of 0.5 SD (change) or higher would require comparison sample sizes per group of 86. In multiple regression, to detect an increase in R2 of 0.03 from 0.45, with a power of 0.8, the number of independent variables equal to 8, and the number of independent variables being tested equal to 8, a total sample size of 270 would be required. With a smaller increase of 0.01 in R2, with a power of 0.8 and the number of independent variables in the model and tested equal to 8, a total sample size of 820 would be required.
Dichotomous HbA1c Outcome Variable (Both RCT and EHR Databases)
Within the pooled RCT sample, approximately 66% and 31% of patients achieved HbA1c end points of <8.0% and <7.0%, respectively. Given an α of .05, power of 0.9, a balanced design with dichotomous event outcomes (eg, HbA1c ≥8.0% = 0 and HbA1c <8.0% = 1) with X = 1 in group 1 of 0.5 and in group 2 of 0.6, the required sample size for a simple logistic regression (1 covariate) is 225. For a multiple logistic regression (α = .05, power = 0.8), where p1 equals the probability that the response variable equals 1 when the predictor is at the mean, p2 is the probability that the response variable equals 1 when the predictor is 1 SD above the mean, and R2is the squared multiple correlation between the predictor variable and all other variables in the model, the required total sample size is 205. The available sample sizes for the EHR data were of comparable or higher number.
Survival, Time to Failure/Success Outcomes (EHR Database Only)
In a simple survival function with α of .05, power of 0.90, Δ of 0.737 (hazard ratio [HR]; eg, 0.5 probability of reaching the target goal vs 0.6), with 3 years duration and follow-up and 10% loss to follow-up (average follow-up in those lost to follow-up of 2 years), a total sample size of 545 in each group would be required.
As such, for all outcomes, we determined that the sample sizes available in the RCT and EHR databases would be sufficient to detect small-to-medium effect sizes with a power of 0.8 or higher.
Time Frame for the Study
This study used a prospective design for both the RCT and EHR data, as previously described, although all data had been previously collected during other studies. The RCT data were collected over a period from 1990 through 2010, whereas the EHR data were collected from 2000 through 2014.
Analytical and Statistical Approaches
Analytical HbA1c Benchmarking Models
For HbA1c effectiveness models, the target goal dichotomous outcome variable, π, represents the probability of achieving success for the glycemic control target of HbA1c <8.0% for one model and <7.0% for another model vs failure with HbA1c ≥8.0% or ≥7.0%. This outcome was assessed at the prescribed last visit of the RCT in the RCT database or at the end of the observation period in the EHR period as described in the “Variables in the EHRs” section. The statistical model estimated π after treatment. Logistic regression modeled the binomial success variable, Y, estimating the odds ratio (OR) for success, whereas the β’s shown below represent the coefficients corresponding to the planned treatment (X1) and personal markers X2 through Xn for the ith patient:
For the EHR data, the time to reaching π was also modeled, adjusting for treatment and patient-centered variables. Estimated HbA1c probabilities for subgroups of interest were calculated using the logistic regression covariate coefficients.
Statistical Methods
Generalized linear models and multivariable logistic regression were used to identify explanatory variables of treatment effectiveness when operationalized by the dichotomized HbA1c target thresholds of <7.0% or <8.0% using both the RCT and EHR databases. Cox regression was used to estimate the time until HbA1c targets were reached. A patient was considered censored if they changed or added a diabetes medication or left the health care system. A change in the medication regimen without reaching a target goal HbA1c indicated failure to achieve accepted glycemic control or indicated the intolerable adverse effects of medication in the observational EHR data. We used multivariable linear regression, analysis of variance (ANOVA), and latent variable structural equation modeling (SEM) to model associations between explanatory variables and outcomes in the pooled RCT database. Modeling was also used to evaluate the magnitude of influence that the demographic, socioeconomic and PRO data had on treatment response variability. Discriminant function and receiver operating characteristic (ROC) curve analyses were used to evaluate the discriminative ability of the factors selected for the case-mix adjustment of benchmarks. Principal-component and factor analyses were used to reduce the dimensionality of multiple outcomes. Last-observation-carried-forward (LOCF) and random-effects modeling were used to account for missing HbA1c end point observations in the RCT databases. It should be noted that the LOCF was the end point used by the sponsors in these FDA registration trials, and no other imputation end point was available to us for analysis. However, the amount of missing data was very small (see % completed EOS values in Table 1b). The LOCF in diabetes trials drives the estimate toward the null hypothesis given that HbA1c seldom increases over time with a study drug. Goodness-of-fit statistics were obtained, including deviance, Pearson χ2, Akaike information criterion (AIC), and the bayesian information criterion (BIC); regression diagnostics included residuals, Cook distance and leverage value, Nagelkerke R2, Hosmer-Lemeshow χ2 statistic, and the percentage of responses predicted correctly.
The regression and latent variable SEMs were based on a conceptual model that postulated that sociodemographic, QOL, and SAT factors influence the probability of reaching the HbA1c target. The between-participant variance in the estimated probabilities reflected HTE attributable to those factors. The QOL factors hypothesized to impact treatment effectiveness were baseline and treatment-related changes in mental health and distress due to adverse effects and symptoms. The SEM allowed for more complex relationships between and among the QOL and SAT scales and their potential influence on HbA1c response.
The prototype RCT benchmarking model used data from protocol 14 (see Tables 1a and 1b; N = 594) and included the outcome of HbA1c <8.0% and the prespecified variables of baseline age, sex, BMI, FPG, HbA1c, duration of diabetes, race, previous diabetes treatment, and planned diabetes treatment. The prototype model allowed us to evaluate the degree of heterogeneity in primarily medication-naive patients from 1 relatively large, multicenter clinical trial while the larger pooled RCT database was being built. Prespecified variables were retained in the model regardless of their statistical significance. The same model was used for the HbA1c <7.0% outcome. As pooling the RCTs progressed throughout the study, subsequent RCT regression and latent variable SEMs evaluated the relationships between HbA1c, QOL, and SAT measurements using the larger pooled RCT database and the sociodemographic, QOL, and SAT measures from the QOL surveys. RCT regressions modeled the PROs as the dependent variables, whereas the latent variable SEMs allow variables to serve as covariates, independent variables, or dependent variables, depending on the defined pathways between variables.
Similar to the rationale for building the RCT prototype model, the prototype EHR benchmarking model was developed using the smaller BWH database while the larger Partners HealthCare database was being constructed. The prototype model included the prespecified baseline independent variables of age, sex, BMI, HbA1c, median income, race, previous diabetes treatment, and planned diabetes treatment. Unlike the RCT prototype model, the FPG and duration of diabetes data were not included because they were not routinely available in the patient records. The EHR prototype model included an initial smaller pooled database of 80 677 patients with diabetes, which yielded 3235 eligible patients, compared with second-phase database, which had 233 627 patients with diabetes and yielded 8107 patients. As mentioned previously, EHR database licensing, access, and programming occurred in phases during the project. The prototype EHR model used only data from the BWH database between the years 2000 and 2010, whereas the subsequent models used data from the entire Partners HealthCare system between the years 2000 and 2014. Also, in the Partners database, we coded from the medical record notes additional dichotomous variables, including evidence of being a current smoker or ever having smoked, being diagnosed with hypertension or depression, taking antidepressants, being diagnosed with dementia, and the eGFR. EHR models from the second phase included hypertension and eGFR. Descriptive statistics and 95% CIs for means and ORs were employed to summarize the effects.
Qualitative and quantitative methods were used to analyze the social media data. Social media text postings were organized and restructured into a relational database as either author attributes or codable text. Text searches, tree diagrams, parsing, and visualization methods were used to construct classification nodes using NVivo, version 11, software. Software-aided qualitative methods were compared with cognitive theme classification without software assistance. Data were analyzed using graphs, charts, pictograms, and other visualization methods to identify predictive author attributes, and the information was synthesized using ranking and prioritizing of topics, domains, nodes, and themes.
Conduct of the Study
The RCT and social media analysis portions of this study received a designation of “Not Human Subjects Research” by the Harvard T.H. Chan School of Public Health IRB because we were using previously collected deidentified clinical trials data and social media data that were publicly available. This EHR analysis was restricted to the Partners HealthCare system and was approved by the Partners HealthCare IRB, and the requirement for written informed consent was waived. This IRB approval by Partners HealthCare was accepted by Harvard.
Results
Several benchmarking models were developed from the RCT and EHR databases. Due to space limitations for this final report, we describe findings for selected models that informed the major goals and objectives as described below.
Pooled RCT Databases
Baseline characteristics for the 6151 patients with type 2 diabetes are shown in Table 1c. A total of 2442 patients had both QOL and SAT measures.
Prototype RCT Benchmarking Model (N = 594)
The RCT prototype model was developed to examine HTE in a highly resourced setting using a strict treatment protocol as part of a placebo-controlled trial. The assumption of being “highly resourced” is based on the increased frequency of weekly clinical visits in addition to clinical support staff, medical supplies, and laboratory tests available in the RCTs. The prototype model clinical trial had previously enrolled patients with type 2 diabetes who were randomly assigned to either DE+P or DE+S (refer to protocol number 14 in Tables 1a and 1b; N = 594). At baseline, patients had mean (SD) characteristics as follows: age, 58.6 (11.5) years; HbA1c, 8.5% (1.4%); FPG, 222.6 (63.4) mg/dL; BMI, 30.6 (5.7); duration of diabetes, 5.4 (5.8) years; triglycerides, 211.6 (144.3) mg/dL; high-density lipoprotein (HDL) cholesterol, 39.4 (11.1) mg/dL; and low-density lipoprotein (LDL) cholesterol, 143.2 (38.4) mg/dL. They were 56.9% male, and their race or ethnicity was 70.2% White, 18% Black, 4.6% Hispanic (any race), and 5% Asian.
At the end of 12 weeks of treatment, 29.3% of patients on DE+P and 66.8% on DE+S reached the HbA1c goal of <8.0%. The corresponding percentages for the HbA1c goal of <7.0% were 6.8% and 35.0%, respectively. HBP model estimates are given in Tables 2a and 2b for HbA1c <8.0% and in Tables 3a and 3b for HbA1c <7.0%. The corresponding ROC curve for HbA1c <8.0% illustrated high sensitivity, specificity, and discrimination, with an area under the curve (AUC) of 0.91 (SE, 0.013), 95% CI 0.885-0.935 (Figure 2), and P < .0001. For HbA1c <7.0% outcomes, the ROC curve statistics were AUC of 0.902 (SE, 0.016), 95% CI 0.871-0.932, and P ≤ .0001.

Table 2a
RCT Prototype HBP Model (N = 594) and Logistic Regression Estimates for OR of HbA1c <8.0%.

Table 2b
Goodness-of-Fit Statistics for RCT Prototype Generalized Linear Model for HBP HbA1c <8.0%.

Table 3a
RCT Prototype HBP Model, Logistic Regression Estimates for OR of AHb1c <7.0%.

Table 3b
Goodness-of-Fit Statistics for RCT Prototype Generalized Linear Model for HBP HbA1c <7.0%.

Figure 2
ROC Curve Illustrating the Sensitivity and Specificity of the HBP Model for Outcome of HbA1c <8.0%.
The logistic regression model used to create the subgroup treatment response profiles for the estimated probabilities of an HbA1c goal of <8.0% and using the 10 independent variables (age, race, sex, BMI, duration of diabetes, baseline FPG and HbA1c, weight, use of a prior diabetes drug treatment, and current drug treatment) yielded the following goodness-of-fit statistics: Nagelkerke R2, 0.62; Hosmer-Lemeshow χ2 = 8.156, df = 8, and P = 0.418; and percentage of responses predicted correctly: 95.2% (HbA1c <8.0% not reached), 62.1% (HbA1c <8.0% reached), and 77.0% (total percentage correct). For the logistic regression model with HbA1c goal of <7.0% reached, the statistics are Nagelkerke R2, 0.547; Hosmer and Lemeshow χ2 = 25.328, df = 8, and P = 0.001; and percentage of responses predicted correctly 98.9% (HbA1c <7.0% not reached), 29.5% (HbA1c <7.0% reached), and 80.8% (total percentage correct).
Because the purpose of this study was to estimate the outcome for specific subgroups and not to predict or forecast outcomes for individual patients, we did not use a best-fitting, validation, or calibration approach. However, to assess fit, we undertook logistic regression estimated with conditional forward selection. The method selected 6 of the 10 variables (race, baseline FPG and HbA1c, duration of diabetes, use of a prior diabetes drug treatment, and current drug treatment) to predict reaching an HbA1c goal of <8.0% yielding the corresponding goodness-of-fit values: Nagelkerke R2, 0.62; Hosmer-Lemeshow χ2 = 15.9, df = 8, and P = 0.044; and percentage of responses predicted correctly: 78.4% (HbA1c <8.0% not reached), 88.6% (HbA1c <8.0% reached), and 84.0% (total percentage correct). For HbA1c goal of <7.0%, conditional forward selection from 5 of the original 10 variables (duration of diabetes, baseline FPG and HbA1c, use of a prior diabetes drug treatment, and current drug treatment), stepwise regression selected all but FPG, and the corresponding goodness-of-fit statistics were Nagelkerke R2, 0.52; Hosmer-Lemeshow χ2 = 19.0, df = 8, and P = 0.015; and percentage of responses predicted correctly: 92.1% (HbA1c <7.0% not reached), 64.4% (HbA1c <7.0% reached), and 84.8% (total percentage correct).
Figure 3 shows the histograms of the logistic model predicted probabilities of HbA1c <8.0% for the 10-variable model, within each treatment group. Each individual estimate is referred to here as an HBP. The extremely skewed distributions of the HBPs illustrate a relatively high level of HTE among patients within the 2 treatment arms, with 31% of patients having an estimated “personalized” HBP between 0.0 and 0.05 (first bar in the histogram) for DE+P. While skewed in the opposite direction indicating the effect of treatment, 25% of DE+S patients’ HBPs were between 0.95 and 1.0 (last bar of the histogram). As shown in Figure 3, the mean (SD) estimated HBP (HbA1c <8.0%) for the 184 patients in the DE+P arm was 0.28 (0.29), the median was 0.14, the IQR was 0.44, and skewness was 0.917. For the 359 patients in the DE+S arm, the mean (SD) HBP was 0.67 (0.32), the median was 0.78, the IQR was 0.48, and skewness was −0.805. Averaging the HBPs within each of the 56 clinics’, the mean (SD) clinic-specific HBPs for the DE+P arm was 0.27 (0.18). These HBPs ranged between 0.01 and 0.87, with a median of 0.26, IQR of 0.21, and skewness of 0.78. For the 58 clinics with DE+S patients, there was a mean (SD) of 0.67 (0.17), ranging between 0.31 and 0.96, median of 0.72, IQR of 0.26, and skewness of −0.251. These results indicate that within both the placebo and sulfonylurea treatment arms, there was substantial HTE among both patients and clinics independent of treatment. First, for the individual HBP distributions, the percentage coefficient of variation, a standard measure of volatility, is 104% in the DE+P arm and 48% in the DE+S arm. There is also strong skewness in both groups, and the IQRs are 0.44 and 0.48 on a probability scale that is restricted between 0.0 and 1.0. The clinic mean HBP distributions are more mound shaped due to the central limit theory, but the SDs of these sampling means are stochastically relatively large.

Figure 3
Prototype HBP Model Showing the Frequency Histograms for the Predicted Probability of Success at Reaching HbA1c Target of <8.0% Within Each of the 2 Treatment Arms.
Table 4 shows the estimated HBPs for 4 subgroups of individuals with various personal and clinical characteristics. As shown, one can refer to a performance benchmark obtained in a clinical setting where all resources were provided and protocols were rigorously monitored. Moreover, changing personal characteristics changed the outcomes even within the controlled health care treatment environment of an RCT. A benchmarking calculator was generated from this prototype model, as discussed under “A Social Media Learning Collaborative Platform for Dissemination of Diabetes Benchmarking Tools.” This calculator was developed solely to estimate the HTE by adjusting any 1 of the 10 prespecified characteristics and not to predict the probability of a future individual’s response with these characteristics.

Table 4
Estimated HBPs for 4 Patients Using Logistic Regression Model Estimates.
RCT Database Models (N = 6868)
When all 19 studies in the database were pooled, there was a total of 6868 patients. Of these, 182 (2.7%) were <18 years of age, all of whom had type 1 diabetes. There were 563 (8.2%) adult patients with type 1 diabetes. Baseline descriptive statistics are shown for adults with type 2 diabetes in Table 1c. For some of the patients, values were missing for several reasons, including patient withdrawal before the first visit and first drug dose, delay in completing a baseline QOL assessment until an unscheduled visit, and the variable not being available in the pooled data repository. Table 1c shows that HbA1c was suboptimal, QOL was between 70% and 77% of the maximum score, and SAT was approximately 65% of the maximum score. QOL and SAT data were not included in the prototype RCT model because this model was developed as a reference for the EHR clinical practice model discussed in further detail in the “EHR Databases” section below. To explore the relationships of patient-reported QOL and SAT on treatment response and heterogeneity, we analyzed subsets of the full RCT database described in Table 1a. The first analysis presented includes all patients from trials that contained the full battery of both QOL and SAT instruments. Subsequent analyses included patients with diabetes with QOL data but not SAT data.
Results for RCT Models Containing QOL and SAT Questionnaires (N = 2927)
Eight of the 19 pooled RCTs (total of 2927 patients, 413 clinics, 18 arms) included 12 different regimens of insulin and OAs (sulfonylureas, metformin, thiazolidinediones) as mono- or combination therapy and contained both the QOL and SAT questionnaires, allowing for a more detailed analysis (see protocol numbers 1-8 in Tables 1a and 1b). Table 5 shows the baseline HbA1c, age, and BMI for patients with type 1 and type 2 diabetes who participated in these trials. Mean QOL and SAT scores at baseline indicated that patients were comparable across treatment categories, with the greatest potential for improvement in the areas of mental health and SAT (Table 6). For those QOL and mental health bidirectional scales with a range of 100 to 600, scores of 100, 200, 300, 400, 500, and 600 approximately correspond to ratings of very poor, poor, fair, acceptable, very good, and excellent health states, respectively. For the unidirectional Side Effects and Symptoms (SES) Distress Scale, the intervals correspond to ratings of very high, high, moderate, some, a little, and no distress, respectively, with higher scores being more favorable (ie, less distress).

Table 5
Selected Mean (SD) Baseline Clinical Characteristics in Study Sample (N = 2927).

Table 6
Selected Baseline QOL and SAT Mean (SD) Scores in Study Sample (N = 2927).
For SAT, scored from 0 to 100, with a mean and median score of 65, and an SD of 15, the 25th percentile of 54 indicates that approximately 25% of this group is generally dissatisfied (ie, scored <54) with their medication, and that overall, there is a high degree of variability among patients. At the end of the treatment follow-up period, 29.4% of patients achieved an HbA1c < 7.0%, and 66.8% achieved an HbA1c <8.0%. It should be noted that some therapeutic assignments were chosen not to optimize glycemic control, but rather to serve as an active control for superiority and noninferiority trials.
Table 7 reports the results of the logistic regression for HbA1c <8.0% and HbA1c<7.0% with QOL scales as independent variables. QOL changes are expressed in standard error of measurement units defining the absolute change as a proportion of the SD of change during a stable (no-intervention) period. For example, for each standard error of measurement unit improvement in “overall QOL,” there was a 10% increase in the probability of HbA1c <8.0%, with an OR of 1.10. The ORs indicate that across these scales, reaching the HbA1c target goal is associated with overall improvement in QOL. Table 8 reports the adjusted ORs for the SAT scales as independent variables.

Table 7
Relationship Between Change in QOL Scales and ORs of Reaching Goal HbA1c.

Table 8
Relationship Between Change in PRO SAT Scales and OR of Reaching HbA1c Goal.
As shown in Table 8, for each standard error of measurement unit improvement in overall satisfaction, there was a corresponding 9% increase in the probability of HbA1c <8.0%, with an OR of 1.09. To assess which subscales independently explained the variability in treatment response, we performed a regression in which the satisfaction subscales were entered simultaneously. The multivariable regression indicated that changes in perceived effectiveness and the adverse effects of the therapeutic regimens independently impacted therapeutic effectiveness. Tables 9 to 11 show the ANOVA results modeling the HbA1c end point controlling for treatment regimen assignment, age, and baseline HbA1c. For the QOL scales as independent variables, we performed model reduction, leaving only the 2 most statistically significant SES Distress and Health Rating Scales in the model. As shown in Table 9, advancing age, lower baseline HbA1c, and improvements in health-related SES Distress and Health Rating Scales resulted in lower HbA1c. For the SAT scales, the reduced model included the perceived effectiveness and side effects scales. As shown in Table 10, although improved perceived effectiveness was associated with lower HbA1c, an increase in the adverse effect–related distress associated with the regimen was also associated with lower HbA1c due to treatment-related adverse effects. Table 11 summarizes the β coefficients for the various diabetes treatments and the covariates for the analyses shown in Tables 9 and 10. Figure 4 shows the magnitude of HTE in HbA1c end point by plotting a frequency distribution of the estimated values from this regression model. The distribution was approximately normally distributed, with a mean (SD) HbA1c of 7.6% (0.7%). Also, as shown in Table 9, after covariate adjustment, the pooled error variance mean square was 0.94, corresponding to an SD of 0.97, indicating a 95% predictive interval (defined as the interval in which a future observation will fall, with a certain probability, given what has already been observed) of nearly 4% units in HbA1c. This reflects a relatively large degree of HTE among patients.

Table 9
ANOVA Modeling HbA1c End Point by Age, Baseline HbA1c, and Assigned Treatment and Change in PRO QOL Scales of SES Distress and the Overall Analogue Health Rating Scale.

Table 10
ANOVA Modeling the HbA1c End Point by Age, Baseline HbA1c, and Assigned Treatment and PRO SAT Subscales of Effectiveness and Adverse Effects.

Table 11
β Coefficients for the 12 Treatment Groups in QOL ANOVA (Table 9) and SAT ANOVA (Table 10).

Figure 4
Distribution of Estimated Values of HbA1c End Point From Regression After Adjusting for Age, Baseline HbA1c, Assigned Treatment, SAT Perceived Effectiveness, and SES Distress Subscales.
Standard Error of Measurement for HbA1cTreatment Response, QOL, and SAT, and Correlations Between Mental Health, Adverse Effects, and Treatment Effectiveness
Given that mental health and distress associated with treatment-related adverse effects could potentially be related to treatment effectiveness, we first examined the correlations between subclinical depression, anxiety, and emerging symptoms and adverse effects (eg, hypoglycemia, gastrointestinal symptoms, and weight gain as well as disease-related symptoms associated with hyperglycemia, such as frequent urination, blurry vision, difficulty concentrating, and fatigue). The QOL scales used in the model were psychological distress and its subscales of anxiety, depression, and loss of behavioral and emotional control (3-subscale Cronbach α = .88) and the SES Distress Scale representing “diabetes distress.” The correlations between the 3 mental health scales and the symptom and side-effects distress scales are given in Tables 12 to 14. These correlations indicate that mental health is associated with patient-reported functional health status, diabetes-specific and general symptomatology, and baseline HbA1c (Table 12). The correlations shown in Table 13 indicate that mental health also is correlated with patient-reported treatment-related adverse effects and symptom distress (diabetes distress) as well as perceived health ratings. Correlations between cognitive functioning scales (performance, disorientation, and detachment and mental acuity) and mental health scales indicated that cognitive functioning worsened or improved as mental health worsened or improved, respectively (Table 14).

Table 12
Baseline Pearson Correlation Coefficients Between Mental Health, Symptom Life Interference Scale, and Baseline HbA1c.

Table 13
Baseline Pearson Correlation Coefficients Between Mental Health, SES Distress, Incidence, and the Perceived Health Rating Scales.

Table 14
Baseline Pearson Correlation Coefficients Between Mental Health and Cognitive Symptoms and Performance Scales.
To further explore these relationships, the baseline psychological distress score (scaled 100-600) was divided into n-tiles (where n = 5), as depicted in Figure 5. The mean and median scores in the lowest 5th-tile group were 390 and 398, respectively; 20% (n = 112) of the 561 individuals in this category scored <358, and 26 of those individuals scored <300, as shown in the box plots depicted in Figure 6. Overall, 20% of this population with diabetes had relatively moderate to severe anxiety, depression, and loss of behavioral and emotional control. The linear regression model of the adjusted mean change in the SES Distress Scale score during diabetes treatment as a function of the baseline psychological distress score is shown in Table 15. To depict this relationship more clearly, we plotted the mean of the SES Distress Scale score as a function of the baseline psychological distress ranked at quintile midpoint (Table 16 and Figure 7). The logarithmic trend indicated that the ordinal 5-level rank representing baseline psychological distress was predictive of the corresponding treatment-related changes in the PRO SES Distress Scale. This graph provides evidence that individuals experiencing higher anxiety, depression, and loss of emotional/behavioral control at baseline will report a higher frequency and degree of severity in the worsening of diabetes-related symptoms of hyperglycemia (eg, frequent urination, fatigue, blurry vision) and adverse effects of medication, including hypoglycemia.

Figure 5
Mean Baseline Psychological Distress Score (Scaled 100-600, With Higher Scores Being Better).

Figure 6
Box Plots of Baseline Psychological Distress Score (Scaled 100-600, With Higher Scores Being Better).

Table 15
ANOVA Table for the General Linear Model of Treatment-Related Changes in the SES Distress Scale Score.

Table 16
Adjusted Mean Change In SES Distress Scale by Ranked Quintiles of Baseline Psychological Distress.

Figure 7
Adjusted Mean Change in SES Distress Scale as a Function of the Ranked Quintile (N-tile Method) of Baseline Psychological Distress.
Latent variable SEM
To explore potential causal and mediation pathways and relationships between the covariates mediator and treatment effect modifiers influencing the effectiveness of diabetes medication to lower BG, we used latent variable SEM. We first applied a relatively simple model to the prototype benchmarking data described previously, as shown in Figure 8. In this model, the QOL latent variable comprised the treatment-related change score (end point – baseline) for the general health subscales of vitality, sleep, and general health, in addition to the cognitive functioning and SES Distress Scale. The glycemic control latent variable comprised HbA1c and FPG change characterizing a treatment-related glycemic response. As shown by the path coefficients, vitality made the largest contribution to the QOL latent variable, with a factor loading of 0.76, whereas cognitive function loaded with a weight of only 0.29, indicating that changes in cognition are most likely affected by treatment differently from the other QOL subscales. The estimated standardized path coefficients obtained from the analysis indicated that a 1.0-SD improvement in glycemic control is associated with a 0.26-SD improvement in QOL. This path analysis supported the relationship between improved glycemic control and treatment-related improvement in QOL.
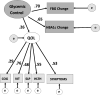
Figure 8
Latent Variable SEM Depicting the Relationship Between Changes in Glycemic Control and Changes in QOL for the Prototype Benchmarking Model.
We next developed a more complex latent variable SEM for the full set of the pooled RCT database patients (5209 evaluable patients, 712 centers, 32 regimens) with full data. The baseline characteristics for this group are shown in Table 17. For this model, we developed a 1-factor latent variable “psychological distress” component composed of the 3 subscales of depression, anxiety, and loss of emotional and behavioral control (Figure 9). The standardized path estimates indicated that the 3 subscales were relatively equally balanced in their contributions to the psychological distress latent variable construct. All path coefficients were significant at P < .0001. We designed the structural part of the model controlling for age, sex, baseline HbA1c, BMI, and baseline SES distress. As shown by the estimated standardized path coefficients, a 1-SD higher (better) score in baseline psychological distress corresponded to an 11% SD greater improvement in the treatment-related changes of the SES Distress Scale. This effect was in addition to the contributions of the baseline SES Distress Scale. Interpreting these results, a 1-SD improvement in the SES Distress Scale during treatment corresponded to a 15% SD decrease (better glycemic control) in HbA1c. Because both measures are changes, the path coefficient also implies that a 1-SD worsening in SES corresponds to a 15% SD increase (worse glycemic control) in HbA1c. Table 18 shows the standardized coefficients for the latent variable SEM depicted in Figure 9.

Table 17
SEM Pooled RCT Patient Population Baseline Characteristics.

Figure 9
Latent Variable SEM Depicting the Baseline Psychological Distress Score on the Emergence of SES Distress and the Unidirectional Impact of Treatment Response on HbA1c End Point.

Table 18
Standardized Coefficients for Latent Variable SEM Depicted in Figure 9.
We then modified this model by making bidirectional the unidirectional path between changes in treatment-related SES distress and HbA1c end point, as shown in Figure 9. Both directional path estimates were statistically significant and indicated that the potential gains in improved HbA1c, of 0.21 SD are offset by 0.08-SD losses due to the adverse effects of treatment. For illustration purposes, we added the psychological well-being latent variable to the model along with the bidirectional path between symptom distress and HbA1c end point (Figure 10). Here, we only show this added component because the remainder of the model was not changed. Adding the baseline positive well-being latent variable, consisting of the 3 scales of emotional ties, general positive affect, and life satisfaction, to the SEM for patients with type 2 diabetes only (vs patients with type 1 diabetes) did not significantly affect the change in the SES Distress Scale during treatment (standardized coefficient = 0.02, P = .511.) The influence of these mental health components indicated that with better baseline mental health, there is greater improvement or lesser negative impact on distress pertaining to diabetes-related symptoms and adverse effects. The bidirectionality of the 2 pathways of symptom distress → HbA1c end point and HbA1c end point → symptom distress suggests that the degree of lowering of HbA1c during treatment could affect changes in distress for individuals with higher distress and lower mental health at the start of therapy. These negative mental health influences could potentially influence adherence, causing reduced compliance and medication effectiveness.

Figure 10
Psychological Well-Being Latent Variable Pathway to the SES Distress Treatment Changes in the Model Shown in Figure 9 for Patients With Type 2 Diabetes Did Not Contribute Significantly to the Explanatory Ability of the Model.
EHR Databases
We used the EHR database to test the feasibility of using a real-world EHR database to estimate CPPs in usual daily practice. As was the case with the RCT models, we present only 2 models: (1) an EHR prototype model comparing the odds of reaching HbA1c <7.0% or HbA1c <8.0% in patients with type 2 diabetes taking metformin compared with those using a sulfonylurea to reach HbA1c A1c <7.0% (N = 2477 patients); and (2) the EHR model for treatment-naive patients treated with their first drug regimen and who were followed until they reached the HbA1c target, switched or added medication, or were no longer followed within the health care system (N = 13 746 records) second-phase database. As mentioned previously, building the EHR database took part in 2 phases, as described below.
Benchmarking Type 2 Diabetes Treatment Effectiveness in Clinical Practice
EHR prototype model (N = 2477)
After developing the HBP model using the RCT data as described previously, we developed a corresponding EHR usual-care clinical practice prototype model using the EHR primary care clinical practice. The benchmarking prototype CPP model was based on BWH and Partners HealthCare EHRs collected from 2000 to 2010. Screening the EHRS by using the eligibility criteria resulted in 3235 potentially eligible patients with diabetes for development of the prototype CPP model, as shown in the patient selection flow diagram in Figure 11a. The updated 2000-2014 data patient flow diagram that included additional providers within Partners HealthCare is shown in Figure 11b. Selected patients all had a diagnosis of type 2 diabetes and HbA1c >7.0%. The frequencies of the 6 selection criteria in these patients are shown in Table 19a. The distribution of baseline HbA1c values in the EHR prototype model database is shown in Table 19b. Patients were followed until they had a switch in their medication or until their last HbA1c was available before a change in medication. Out of the 1491 patients who entered with HbA1c >7.0%, 54.2% achieved HbA1c <7.0% on their starting therapy. An additional 16.2% achieved HbA1c between 7.0% and 7.99%, for a total of 70.4% with HbA1c <8.0%, before the need for a change in medication. Of the 3235 patients, we then selected only those patients who had started their initial therapy on either sulfonylurea or a biguanide (metformin), and the start of their observation period was the date of the visit when their first medication was prescribed (N = 2477). The characteristics of this subgroup are given in Table 20a, and the time to HbA1c end point summary statistics is given in Table 20b.

Figure 11a
Flow Diagram of Patient Selection for Building the Developmental Prototype for CPP Model.

Figure 11b
Flow Diagram of Patient Selection for Building the Production CPP Benchmarking Database for Patients Who Were Treatment Naive.

Table 19a
EHR Frequency of Patients Within the 6 Selection Criteria for the 2000-2010 and 2000-2014 Databases.

Table 19b
EHR Prototype Model (N = 3235): Frequency Distribution of Patients by Baseline and HbA1c End Point in Total Sample.

Table 20a
Characteristics of EHR Prototype Model Patients With Type 2 Diabetes Taking Either Metformin or a Sulfonylurea (N = 2477).

Table 20b
Percentiles and Mean Time to HbA1c End Point for Prototype Model Comparing Patients With Type 2 Diabetes Taking Either Metformin or a Sulfonylurea (N = 2477).
The patient-centered variables included in the prototype logistic regression model were age (years), sex, race/ethnicity (White [reference], Black, Hispanic, Asian, other non-White), income (geocoded from patient’s residence zip code), BMI, and HbA1c (%). The target outcome variable and the statistical estimation methods were conducted as previously described for the RCT prototype model. Two logistic regression models with multiple covariates were run to estimate the probability of achieving HbA1c <7.0% and <8.0% at end point. Due to space limitations, we present selected results here. The corresponding EHR model <8% ROC curve shows only fair discrimination (Figure 12), with an AUC of 0.73, compared with the RCT prototype model for the <8.0% target, where the AUC was 0.91. The area under the receiver operating characteristic curve (AUROC) increased by only 0.02 units when statistically significant polynomial terms were included for continuous covariates. Figure 13 shows the estimated probabilities for an HbA1c <7.0% by logistic regression for drug class set to sulfonylurea, median annual income of $51 389, baseline HbA1c 8.7%, and BMI of 33.4 for race and sex categories. The AUROC for the <7.0% HbA1c target was only 0.60, indicating that the discrimination of the model using these values for the covariates for this group was low given that an AUC of 0.5 indicates no discrimination.

Figure 12
ROC Curve for EHR Prototype (N = 2477) HbA1c <8.0% Logistic Regression Model.
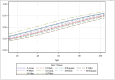
Figure 13
EHR Prototype Model Predicted Probabilities for HbA1c <7.0% by Logistic Regression for Drug Class Sulfonylurea, Median Annual Income of $51 389, Baseline HbA1c of 8.7%, and BMI of 33.4.
After controlling for covariates, metformin was 1.8 times (95% CI, 1.4-2.3) more likely to achieve HbA1c <7.0% and 2.2 times (95% CI, 1.7-2.8) more likely to achieve HbA1c <8.0% compared with sulfonylurea. Controlling for treatment, older age, lower baseline HbA1c, and male sex were associated with a greater probability of success for HbA1c <7.0% than younger age, higher baseline A1c and female sex. Sex was no longer significantly associated for HbA1c <8.0%. Table 21a displays the prototype CPP model in the format of a benchmarking calculator for HbA1c <8.0%. Using this calculator, the user can enter the patient subgroup characteristics and treatment to estimate the benchmark probability of reaching a goal in the usual-care setting.

Table 21a
EHR Prototype Model CPPs for Patient With Diabetes With Specific Characteristics (HbA1c <8.0%).
Table 21b displays the corresponding EHR prototype model logistic regression statistics. The coefficients in the table are the estimates from the EHR prototype model logistic regression. As shown here, if a Black woman, aged 50 years, with a BMI of 36, annual income of $50 000, and baseline HbA1c of 9.5% (similar to the prototype RCT case 1 from Table 4) were initially treated with a sulfonylurea, the estimated EHR CPP for reaching the HbA1c goal of <8.0% would be 0.54, and for a goal of <7.0%, it would be 0.41. In contrast, if initially treated with metformin, the EHR CPPs would be 0.72 and 0.56, respectively. Corresponding EHR CPP values for a White man with characteristics similar to the RCT prototype case 2 (age, 50 years; BMI, 30; annual income, $50 000; and baseline HbA1c, 9%) initially treated with a sulfonylurea would be 0.63 for HbA1c <8.0% and 0.51 for HbA1c <7.0%. If treated with metformin, the values would be 0.79 and 0.65, respectively.

Table 21b
EHR Prototype Model CPP Logistic Regression Summary Statistics for Patient With Diabetes With Specific Characteristics (HbA1c <8.0%).
For comparison purposes, Table 22 displays the RCT HBP model described previously for a Black female patient (case 1, Table 4). Although the covariate characteristics were not identical, the patients in Tables 21 and 22 did have the same, age, sex, BMI, race, and baseline HbA1c. The therapy common to both models is the sulfonylurea. The HBP model yielded a probability of reaching the target of HbA1c <8.0% (0.78) for this patient, which was 44% [(0.78-0.54)/0.54)] higher than what was achieved in clinical practice, even when follow-up was only 3 months for the high-benchmark RCT, and the median follow-up was 8 months for clinical practice. However, the success rate of 72% with metformin as initial therapy in clinical practice was only slightly lower (by 6%) than the 78% found for the sulfonylurea RCT high-benchmark reference. This means that under common clinical practice standards—not the highest possible standards (ie, care given to patients in an RCT of medication for diabetes) as was the case for the HBP—there is a 0.54 probability of achieving the HbA1c target goal of <8.0% with these patient characteristics using a sulfonylurea. This is substantially lower than the 0.78 probability under the RCT best-practice high benchmark. On the other hand, the common clinical practice probability of 0.72 for metformin was much closer to that of a sulfonylurea under the best-practice setting.

Table 22
RCT HBPs (HbA1C <8.0%) for Patient with Similar Characteristics to Patient in Table 21a.
EHR Medication-Naive Model (N = 8107)
To further characterize the magnitude of HTE within difference classes of treatments, we expanded the number of records available for developing the benchmarking models by including more Partners HealthCare providers through MGH in addition to patients from BWH. We also included an additional 4 years of observation from 2010 to 2014. This yielded 233 627 records for patients with diabetes, including 43 294 new diabetes medication records followed by PCPs between 2000 and 2014, as shown previously in Figure 11b. We then included only medication-naive patients with type 2 diabetes being treated with DE alone and who had never received diabetes medication. As shown in Figure 11b, this reduced the group to 14 019 medication-naive records (8257 patients).
Because there was not a structured type 1 or type 2 diabetes flag in the database, we further eliminated patients aged <45 years who received insulin as their very first diabetes medication at the time of diagnosis, because a new diagnosis of type 1 diabetes is rare in individuals aged ≥45 years (n = 146). We also eliminated patients with HbA1c end-point values that were obvious reporting errors (eg, an HbA1c end point of 1% [n = 4]), for a final total of 8107 medication-naive patients. The patient characteristics and initial monotherapy regimens for these patients are given in Table 23a. The initial monotherapy regimens among these patients are given in Table 23b. Of these 8107 patients, 80.0% reached the HbA1c target <7.0%, and 87.2% reached the HbA1c target <8.0% without needing to change diabetes medication. For those achieving their HbA1c target <7.0% (n = 6488), the median baseline HbA1c was 7.9%, and the median last HbA1c value after or at the point of reaching their target was 6.6%. The median time to target was 187.5 days for this group. For the 1619 patients failing to reach the HbA1c target <7.0% before a medication switch or for those who were withdrawn from observation without reaching their target, the corresponding median statistics were HbA1c of 8.5% at baseline and 8.3% before medication switch or withdrawal. The follow-up time for this group was 1289 days. As shown in Table 23b, this medication-naive group included 6258 patients who were initially started on either monotherapy metformin or monotherapy sulfonylurea. This comprised 77% of the medication-naive total population. Of these, 84.7% reached the HbA1c target <7.0%, and 90.8% reached the HbA1c target <8.0% before a change in their diabetes regimen.

Table 23a
EHR Database: Characteristics of the Medication-Naive Patients With Type 2 Diabetes Entering the Observation Period (N = 8107).

Table 23b
EHR Database: Demographics, HbA1c End Points, and Starting Diabetes Drug Class of the Medication-Naive Patients.
Comparing the monotherapy metformin group with any other diabetes medications group and with sulfonylurea only, we show the logistic regression model coefficients for achieving an HbA1c <7.0% using sex, age, race/ethnicity, income, BMI, hypertension, HbA1c, eGFR, and treatment as covariates in Tables 24a and 24b. From the logistic regression results, we see that both groups (all other medications: Table 24a, and sulfonylurea only: Table 24b) had lower odds of reaching an HbA1c <7.0% than did those using metformin. Controlling for treatment, we see that younger patients and women were also less likely to reach the HbA1c target goal than were older patients and men. Diagnoses of hypertension and slightly worse kidney impairment (lower eGFR) were associated with a higher probability of reaching the HbA1c target. Goodness-of-fit statistics were calculated using a generalized linear model with binary response and a logit link function. The information criteria are in smaller-is-better form, and the full log likelihood function is displayed and used in computing information criteria.

Table 24a
EHR Medication-Naive Database ORs for Demographic, Socioeconomic, and Treatment Regimen Variables for Reaching HbA1c Target <7.0% (All Diabetes Medications, N = 8107).

Table 24b
EHR Medication-Naive Database ORs for Demographic, Socioeconomic, and Treatment Regimen Variables for Reaching HbA1c Target <7.0% (Sulfonylurea and Metformin Monotherapy Only, N = 6258).
Similar estimates were observed for the HbA1c outcomes of <8.0% using the same logistic regression models (Tables 24c and 24d). Figure 14 shows the ROC curve for the HbA1c <8.0% regression model described in Table 24d. Modeling all classes of type 2 diabetes therapies, we found that each medication or combination of medications compared with metformin had lower odds of an HbA1c <7.0% (insulin: OR, 0.28 [95% CI, 0.23-0.34]; multiple agents: OR, 0.32 [95% CI, 0.27-0.37]; DPP-4i: OR, 0.38 [95% CI, 0.16-0.91]; other: OR, 0.41 [95% CI, 0.18-0.92]; thiazolidinedione: OR, 0.56 [95% CI, 0.35-0.91]; sulfonylurea: OR, 0.74 [95% CI, 0.61-0.90]) (Table 25).

Table 24c
EHR Medication-Naive Database ORs for Demographic, Socioeconomic, and Treatment Regimen Variables for Reaching HbA1c Target <8.0% (All Diabetes Medications, N = 8107).

Table 24d
EHR Medication-Naive Database ORs for Demographic, Socioeconomic, and Treatment Regimen Variables for Reaching HbA1c Target <8.0% (Sulfonylurea and Metformin Monotherapy Only, N = 6258).

Figure 14
ROC Curve for EHR Medication-Naive Database (N = 8107), Logistic Regression Model for Predicting HbA1c <8.0%.

Table 25
Logistic Regression EHR Medication-Naive Database ORs for Reaching HbA1c Target <7.0% Comparing Different Classes of Diabetes Medications With Metformin Monotherapy (N = 8107).
The HRs from the Cox regression model using the same set of covariates that we used for the multivariable linear regression models with an HbA1c end point of <7.0% (described in Tables 24a and 24b) are shown in Tables 26 and 27. Given that the person-days of follow-up varied among patients, as shown in Table 20b, we conducted a Cox regression model to investigate the variability of treatment effectiveness in terms of the amount of time it took to reach the HbA1c target goal, rather than just whether or not patients reached the HbA1c target goal as provided by the logistic regression analysis. The Cox regression showed that compared with metformin, patients categorized by all diabetes medications except metformin and sulfonylurea only reached the target goal more slowly. The time-to-goal survival curve comparisons between metformin and sulfonylurea monotherapy are shown in Figure 15. Younger patients and those who were female were more likely to reach target goal more slowly than older patients and those who were male. Patients who were Black or another race were more likely to reach target goal more slowly than were White patients. As also shown in Figure 15, approximately 40% of individuals in both the sulfonylurea and metformin groups rapidly reached the HbA1c target <7.0% compared with the remainder of the cohort, diverging in the rate of achieving the HbA1c target substantially after that time point. Among those reaching the goal before a switch in medication, the mean time to goal was 362 days for metformin and 471 days for sulfonylurea. When the start of the observation year was included in the logistic regression model, there was no trend over time detected; however, in the Cox regression model, a more recent start year was associated with reaching the goal more quickly. All other regimens (including sulfonylurea) combined had a lower HR of reaching an HbA1c <7.0% than metformin (HR = 0.61 [95% CI, 0.58-0.65], P < .001; N = 8107; Table 27). When each medication was compared separately with metformin, the HRs for reaching the HbA1c goal were all lower than those for metformin monotherapy (Table 28).

Table 26
Cox Regression EHR Medication-Naive Database HRs for Reaching HbA1c Target <7.0% (N = 6258), Comparing All Sulfonylureas With Metformin.

Table 27
EHR Medication-Naive Database Cox Regression HRs for Demographic and Treatment Regimen Variables for Reaching HbA1c Target <7.0% (N = 8107), All Other Medications vs Metformin.

Table 28
EHR Medication-Naive Database Cox Regression HRs for Models That Use Demographic and Treatment Regimen Variables to Estimate Reaching HbA1c Target <7.0% (N = 8107), Each Medication vs Metformin.
It should be noted that even though EHRs are collected longitudinally, and clinical trials typically use fixed-time-interval HbA1c assessments, one can compare the EHR data with the RCT data by using survival models to estimate the probability of reaching the HbA1c target for an individual given their characteristics at a specific time t. The Cox survival model that we described previously could be characterized by S(t|x) = exp [−exp(Bx) * (Ho(t)], where B refers to the coefficients, x is the covariate value, and Ho(t) is the cumulative hazard at time t. As such, the desired fixed-time probability estimate could be obtained by fitting a Cox model to a given survival object and applying the estimated coefficients to each individual depending on their individual covariates.
Social Media, Survey Databases, and Web-Based Benchmarking Calculators
Social Media Needs Assessments and Potential Barriers to Web-Based Applications
Internet applications are increasingly used to access and disseminate health information; however, there are limited data on their use in individuals with diabetes. To evaluate our social media implementation and dissemination plan for patients that included Web 2.0 technology, we analyzed a cross-sectional, probability-based telephone survey of 3014 US residents87 using survey-weighted descriptive statistics and logistic regression. Overall, 78% (up 5% since 2010) of US residents reported using the internet, of which 73% looked for health information online during the past year. As shown in Table 29, the logistic regression analysis indicated that men were less likely than women to use the internet. Internet use increased with annual income and education and decreased for each successive decade of age. Race and diabetes diagnosis were not significant predictors of internet use after controlling for age, sex, income, and education. A total of 374 (12.7%) of the survey respondents reported having diabetes. The diabetes subsample reported had a mean (SE) age of 59.9 (1.1) years; 70% were White, and 17% were Black; 30% were employed full or part time, 15% were unemployed, and 41% were retired; and 50% were married, 13% were divorced, and 12% were widowed. Diabetes comorbidities were hypertension in 66%, heart disease in 28%, pulmonary disease in 25%, and other conditions in 39%. Of the respondents with diabetes, 47% used email, 29% used a mobile device (cell phone, tablet, or other handheld), and 53% used the internet for information on a specific disease (60%). Individuals with diabetes also used the internet to retrieve information on treatments and procedures (50%), learn how to lose weight (28%), research a specific drug they saw advertised (23%), learn about test results (22%), and post experiences about a drug or treatment (7%). As shown in Table 30, individuals with diabetes were more likely to use the internet to get their health information than were those without diabetes.

Table 29
Logistic Regression Modeling Internet Use, “Do You Use the Internet at Least Occasionally?” by Sociodemographic Characteristics.

Table 30
Logistic Regression: Impact of Having Diabetes on the Likelihood of Responding “Yes” to Using the Internet to Obtain Various Types of Health Information After Adjusting for Predictors Shown in Table 29.
Using Social Media–Crowdsourced Data for Diabetes Patient-Centered Outcomes Research
Traditionally, brainstorming and focus groups are used to solicit patient feedback and gather input for health programs and research; however, we hypothesized that social health networks could potentially collect such information in a less structured and artificial environment. To evaluate the feasibility of using social networks to disseminate and enhance our models and benchmarking tools, we retrospectively tracked postings from online diabetes communities and general online health communities. In one analysis, 300 members were randomly chosen and unstructured textual data abstracted. Of these members, 88% had type 2 diabetes and 10.3% had type 1 diabetes, with mean (SD) diabetes duration of 22.0 (18.5) years for type 1 and 6.6 (6.6) years for type 2. Social media membership duration (October 2009-October 2014) was 2.6 (1.5) years. The 300 selected posts from the 300 members included 48 470 words averaging 162 words per post per member, ranging from 2 to 861 words per post. The postings dated from January 1, 2010 through April 23, 2015, with 1 (<1%) post in 2010, 12 (4%) in 2011, 36 (12%) in 2012, 85 (28%) in 2013, 131 (44%) in 2014, and 35 (12%) in 2015. These postings were in addition to clinical or other data available. Time since last online visit and last posting, number of total posts ever written, and number of peer commendations (as of April 27, 2015) were positively skewed, with 25% visiting the member website in the past 5 weeks and posting within the past 8 weeks, 50% visiting within the past 8 months and posting within the past 10 months, and 75% visiting and posting within the past 18 months. The median total number of posts was 90.2 (IQR, 304; range, 1-15 947), and the median total number of commendations was 15.5 (IQR, 40.4; range, 0-1917).
Qualitative content analysis found that 35% of patients with type 1 diabetes used an insulin pump. Of patients with type 2 diabetes, 70% were taking metformin, with or without other oral medications; 12% were taking insulin, with or without metformin; 9% were using DE only; and <10% were taking other diabetes medications not including metformin. Clinical information on HbA1c, FBG, and weight, where available, is summarized in Table 31. Major posting themes for members with type 1 diabetes were use of insulin pumps (19%); anxiety, BG control, use of continuous glucose monitoring (CGM), hypoglycemia, and symptoms (each 11%); depression and support (each 7%); and complications, daily living, and exercise (each 4%). Posting themes for members with 2 diabetes concerned diet and meals (16%); medications (15%); BG control (12%); diagnoses and complications (each 7%); exercise (6%); BG testing and HbA1c (each 4%); symptoms, health care, and daily living (each 3%); anxiety, comorbidities, hypoglycemia, insulin pumps, smoking, and support (each 2%); and CGM, insurance, prediabetes, pregnancy, supplements, and weight loss (each 1%).

Table 31
Clinical Information Obtained from Social Media Public Postings of Patients With Diabetes.
Among the 300 authors, there were a total of 158 558 postings, and we analyzed a sample of 300 postings (0.2%) rated as providing the greatest amount of content and relevance that included a total of 48 470 words. Initial classifications were undertaken by 2 raters. One rater used software to facilitate classification, whereas the other rater did not use software. The rater who did not use software identified 1019 instances from 1500 potential posting classifications (300 × 5), resulting in 33 broad classification themes, reduced from 50 more specific themes, whereas the software-assisted rater identified 1114 phrases out of 48,470 words, resulting in 28 classification nodes. The horizontal branching diagram (dendrogram) displayed in Figure 16 maps the contextual relationships among the themes identified by the qualitative analysis of the diabetes social media postings containing the online conversations of 300 individuals with diabetes involving the 24,105 words available from the sample of postings. This diagram is based on a cluster analysis that allows one to visualize social media–posting word patterns and relationships by grouping sources or nodes that share similar words, share similar attribute values, or are coded similarly by nodes. Sources or nodes in the diagram that appear close together are more similar than those that are far apart. The hierarchical structure allows one to see the parent and child domain relationships. For example, patient postings contain comments about government and policy and about tobacco and smoking that fall outside the primary conversation. Issues related to patient satisfaction dominate discussion, including comments about complications and physical functioning, positive mood and weight issues, social support, diabetes and health care providers, and several other subdomains. Comments about alcohol are associated with low BG and high BG. There is also a technology domain that offers its own area of discussion involving CGM, insulin pumps, alternative therapies and diabetes, and health applications. Figure 16 provides a graphical representation of sources or nodes to make it easy to see similarities and differences in 1 visual snapshot. We used these results to cross-validate some of the findings from our RCT models that highlighted the role of mood, anxiety, distress, and weight in achieving HbA1c target goals.

Figure 16
Dendrogram Mapping the Contextual Relationships Among Themes Identified by the Qualitative Analysis of Diabetes-Related Social Media Postings.
Social Media Learning Collaborative Platform for Dissemination of Diabetes Benchmarking Tools
We developed an online, case-based learning, interactive training tool kit as part of a social media learning collaborative feasibility study to facilitate translating our results so as to better evaluate the quality of clinical practice. The learning objective of the tool kit was to improve clinical competency adhering to the ADA and AACE guidelines for individualizing HbA1c targets based on patient-centered demographic, clinical, psychological, and socioeconomic differences, and to decrease disparities by helping clinicians understand the HTE within their patient population. Using the models and benchmarking calculators described in previous sections, we developed online apps using WebOS-, Android-, iOS-, and Windows-compatible WordPress software. Calculators were field tested and optimized within case-based learning exercises allowing the user to simultaneously modify patient subgroup characteristics to explore and visualize how different subgroup patient profiles might influence the probability of reaching target glycemic goals (see Figure 17a). Calculator 1 in Figure 17a allowed the user to evaluate DE vs DE+S monotherapy in patients with type 2 diabetes after 3 months of therapy using the HBP model from the RCT data described in Table 4 with the illustrative case given in Appendix 1.

Figure 17a
Calculator 1. Prototype HBPs Based on Data From Protocol 14 (Tables 1a and 1b) Entitled, “Diet and Exercise vs Sulfonylurea Monotherapy in Patients With Type 2 Diabetes”.
Preliminary evaluation of benchmarking tool case exercises
For the calculator 1 exercise, the user was asked to input the appropriate values for this case so that the calculator displays the HBPs for the DE only option. Then, the user was asked to compare those values with what would have been the HBP had the subgroup of patients defined by the set of characteristics chosen by the user been placed on a sulfonylurea instead of DE only. The calculator output for each case is shown in Figure 17a. Several online exercises allowed the user to change the input patient characteristics to demonstrate how factors such as sex, age, race, BMI, duration of diabetes, baseline FG, and HbA1c could result in different estimated HBPs.
Figure 17b shows 2 additional online calculators. The calculator 2 case exercise used the estimated coefficients from the EHR prototype benchmarking calculator obtained from clinical practice, and the user was referred to the same case description as described for calculator 1. Within the calculator 2 exercise, 2 reference databases were given. The phase 1 database used the EHR prototype data for medication-naive patients (N = 2477), whereas scenario 2 used the EHR expanded phase 2 database of medication-naive patients (N = 10 446 records, 8107 patients). The 2 scenarios represent different clinic populations with different case mixes. The calculators and case-based exercises were made available from the Diabetes and Stakeholder Communication Forum website (Figure 18 and Appendix 1).
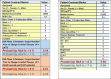
Figure 17b
Benchmarking Calculators.

Figure 18
Homepage of the Diabetes Stakeholders and Partner Forum Communication Portal’s New Social Media Learning Collaborative Website Where Benchmarking Tool Kits, Presentations, Posters, Publications, and Surveys Will Be Hosted.
Discussion
Decisional Context
By analyzing data from pooled RCTs and EHRs, we demonstrate how individual patient characteristics can potentially affect diabetes treatment response. Using pooled RCT and EHR data sources to incorporate demographic, socioeconomic, and patient-reported QOL and SAT into diabetes treatment goals and management is consistent with recommendations from the ADA and AACE/ACE guidelines. The breadth and scope of the diabetes epidemic ensures that the characteristics of the affected populations will continue to be highly variable and diverse, making responses to diabetes therapies susceptible to substantial variability. What makes our method of diabetes management personalization unique is the evidence-based incorporation of quantitative metrics into HbA1c benchmarking algorithms.
There is already strong epidemiologic evidence that the prevalence of diabetes impacts subgroups of the population by age, obesity, race/ethnicity, and socioeconomic status; however, tailoring treatments to patients using these factors is not common. A large portion of the increased prevalence of type 2 diabetes will occur in middle-aged working adults as the prevalence of obesity increases in that population.101 The percentage of deaths attributable to diabetes occurring before age 70 years is reported to be higher in low- and middle-income countries than in high-income countries.102 In the United States between 1994 and 2014, the age-adjusted prevalence of diabetes increased across all educational levels; however, it remained consistently higher for individuals with lower education. The prevalence for individuals with less than a high school education doubled from 6.8% to 13.6%; for those with a high school education, prevalence increased from 4.4% to 9.9%, and for those with more than a high school education, it increased from 3.5% to 8.3%.103 Racial and ethnic disparities in quality of care for diabetes have been explained to some degree by lack of insurance coverage and education.104
The association between neurological,105 cognitive,106 psychological functioning,107 and behavioral factors108 and diabetes has also been documented; however, applying these patient characteristics to precision medicine is even less common than applying demographic and socioeconomic characteristics. Patient-reported QOL outcomes can also yield valuable information for tailoring treatments; however, most clinics do not incorporate such assessments into their practices. Although patient SAT has been shown in some studies to impact treatment effectiveness and adherence to diabetes medication,109,110 tailoring therapies according to SAT is usually accomplished by trial and error in each patient. Our results indicate that both QOL and SAT baseline levels and associated changes during treatment can affect HbA1c outcomes.
Study Results in Context
Treatment differences associated with diabetes and its complications are related to a complex set of issues that include demographic, social, psychological, and economic factors. Because lifestyle-related behaviors such as DE and adherence to diabetes medications are largely under the patient’s control, research yielding information on the effectiveness of diabetes prevention and treatment in terms of patient-centered outcomes can inform patients, caregivers, and clinicians about how these modifiable patient characteristics can influence the effects of treatment. Our study results fill in part a critical gap in current knowledge as noted by the Agency for Healthcare Research and Quality (AHRQ)-sponsored systematic reviews, recommendations for development efforts, and suggested research prioritizations discussed below. Given that most diabetes clinical trials are conducted as part of the drug approval and postmarketing process and do not compare across drug classes in large numbers of diverse patients, our results pooling RCTs and using EHR databases can provide some of the additional evidence required for personalization. According to a 2014 government report, the cost of just 1 moderately sized Phase 3 clinical trial in endocrinology was $17 million, whereas a somewhat larger postmarketing study costs $27 million.111 We consider recycling RCT data to carry out secondary analyses of what needs to be included in the personalization models a worthwhile endeavor.
Data investigating the role of pretreatment and treatment-emergent factors on HbA1c response have been very limited. The first comprehensive, systematic review of the comparative effectiveness of oral medications for type 2 diabetes was published in 2011. It included 216 comparative clinical studies and concluded that most oral diabetes medications had a similar effect on reducing HbA1c; most drugs except for metformin and acarbose caused increases in body weight; and only metformin decreased LDL cholesterol.112 Relevant to our current research results, the authors concluded that the question, “Do oral diabetes medications for the treatment of adults with type 2 diabetes differ in their ability to influence other health outcomes, including quality of life and functional status?” was not answerable because of a lack of data. The review committee stated:
No conclusions could be drawn regarding the comparative effects of the medications on quality of life and functional status because of a limited number of studies and differences in the questionnaires used to assess quality of life.
This 2011 review was updated and again failed to report any information on glycemic outcomes or PROs by subgroups stratified by patient-centered or demographic, socioeconomic, or cultural characteristics that might respond differently.113,114 The authors also reiterated that PROs, such as medication adherence and barriers to adherence, and health-related QOL are important to consider in tailoring diabetes treatments. Subsequently, the American College of Physicians released their guidelines for oral pharmacologic treatment of type 2 diabetes based on the AHRQ systematic review.115 Another 2010 systematic review and meta-analysis of 61 placebo-controlled studies analyzed the treatment effects for different oral hypoglycemic drug classes, also noting the variability in effects.116 We believe that our results and methodologies address the very issues cited by the AHRQ, ADA, and AACE/ACE guidelines and consensus reports by focusing on the influence of individual patient characteristics and patient-centric measures on the therapeutic impact on glycemic outcomes.
Implementation of Study Results
The implementation of study results has implications at 3 levels: research policy, health systems, and clinical practice. The focus of our implementation and dissemination of results follows from these 3 areas, namely, diabetes research on precision medicine, benchmarks for diabetes quality of care, and clinical practice performance education. Concerning the first area, the Precision Medicine Initiative (now, the All of Us Program) was first announced in January 2015 (Collins and Varmus117) and was subsequently codified into law in December 2016 through the 21st Century Cures Act.118 The Cures Act provides multiyear funding for NIH’s Precision Medicine Initiative to “gain better insights into the biological, environmental, and behavioral factors that drive these diseases” by supporting precision medicine as “an emerging approach for disease treatment and prevention that considers individual variability in environment, lifestyle, and genes for each person.”119 Although funding is initially targeted to genetic testing for cancer research, our findings would support funding future research to discover more about the physiological, environmental, and behavioral factors that can help personalize medicine. In addition, the Cures Act included language to incorporate the voice of the patient and real-world evidence by using PROs and EHRs in drug development and research.
We envision using our benchmark models to assess the quality of diabetes care longitudinally among most or all patients with diabetes in a given practice. Longitudinal comparative benchmarking within and between practices can be used to guide “the decision cycle for person-centered glycemic management in type 2 diabetes using SMART (specific, measurable, achievable, realistic, time limited)” goals as detailed by Harris et al.7 Improvements in reaching an HbA1c target can be benchmarked against a given baseline adjusting for the patient characteristics, factors that affect treatment choices, and QOL. The RCT data and benchmarking models provided high internal validity and could also be used to set an adjusted high-quality benchmark for reaching HbA1c target goals. Rather than aiming for a single HbA1c target for the entire population, our results support creating several targets defined by individuals with similar characteristics known to impact treatment response. For example, if the HBP as set by the RCT database for an individual with known characteristics of age, sex, baseline HbA1c, BMI, depression, income, and education yields an HBP of 0.8 for achieving an HbA1c <7.0% on a regimen, the calculated CPP score of 0.6 would yield a performance compliance score not of 0.6/1.0 (60%), but of 0.6/0.8 (75%). The success rate in meeting HbA1c targets in patients with similar clinical characteristics, and thus similar CPPs, is the key measure for comparing one practice with other usual-care or benchmark practices. Knowledge regarding HTE can also be used to assess the potential impact on target HbA1c goals of personal characteristics that are modifiable or nonmodifiable independent of health care delivery and quality. For example, if individuals of the same age, BMI, treatment, sex, and socioeconomic status still exhibit large variability in HbA1c response, one could search for potentially modifiable factors, such as anxiety, depression, memory impairment, and sleep disturbance, that could explain a portion of that variability.
To be clear, there are fundamental differences between predictive individual HbA1c targets and population-based benchmarks for quality assessment. Our method does not address the former. An individual may be unlikely to meet an HbA1c target, but from a clinical standpoint, that target may still be the most appropriate one. However, if certain segments of the population are more or less likely to meet a particular HbA1c target after adjusting for individual characteristics, this could be useful information to prioritize the next step to optimizing glycemic control. Furthermore, using a calculator that indicates that certain individuals are unlikely to meet a particular HbA1c goal could be used to offer additional mechanisms of support that could help patients achieve their goal, thereby potentially reducing health disparities.120
The third level involves clinical practice applications. In clinical practice, maintaining an HbA1c <7.0% in individuals with either type 1 or type 2 diabetes has been shown to reduce microvascular and some macrovascular complications.121,122 Our results allowed us to develop web-based benchmarking calculators within the context of educational case studies. Data from the RCTs allowed models to estimate the high-benchmark standard for an individual patient, and the EHR provided probability estimates with high external validity. Although the HBP-adjusted scores are a useful reference, case-mix–adjusted benchmarking across different practices is also possible. The primary goal for any quality measurement program is to improve medical care to produce better health outcomes. Diabetes has been a focus of performance measurement for decades, serving as one of the first conditions for which disease-specific indicators based on evidence-based clinical guidelines have been used to evaluate the quality of care and preventive services.123,124 However, diabetes performance measures seldom consider case-mix adjustment.125 One recent tool, the Joslin Clinical Analytic Tool (JCAT), similar to the benchmarking calculators developed in our study, stratifies the population of individuals with diabetes into treatment groups and subpopulations that are most appropriate for targeted quality improvement efforts. The information for JCAT is taken from EHRs, which do not routinely collect information on PROs. Our results support collecting more detailed PRO data as part of routine clinical practice so that individual patient information can be used to implement personalized diabetes treatment quality-of-care programs.
We also found that crowdsourced observational studies can complement traditional group creativity methods for soliciting patient feedback and input for validating which characteristics are important to patients and should be included when personalizing HbA1c targets. Our crowdsourced data identified that social support, mood, weight, symptoms, and adverse effects were primary areas of online discussions, which were consistent with the factors identified in the RCT benchmarking models. Furthermore, we found that online, case-based, interactive training tool kits as part of a social media learning collaborative can be used to facilitate translation of patient-centered outcomes knowledge into clinical practice. The learning objective of the tool kit is to improve clinical competency adhering to the 2016 ADA and AACE guidelines for individualizing HbA1c targets based on patient-centered demographic, clinical, psychological, and socioeconomic differences and disparities. We believe that the practical utility of our research findings and tools is that they can be used by clinicians to set expectations for what is likely to be achieved in each patient with certain characteristics, and perhaps to modify the clinical practice standards to which clinicians are held .
Generalizability
The generalizability of the study results is limited to the characteristics of the individuals included in the RCTs and EHRs and also to those who met the inclusion criteria in the EHR database of having at least 2 notes in the medical record at least 1 year apart. As such, the usual-care benchmarking references might be somewhat higher in quality than would be expected in an average clinical practice. In addition, the most recent data are most reflective of current practice patterns. However, the benchmarking methodology is readily generalizable to any population where RCT data and EHRs are available for inclusion in the database. Regarding using social media, our analysis indicated that increasingly, individuals with diabetes are using the internet to manage their condition, but determinants of usage and access, such as age, sex, education, and income, still need to be considered when designing web-based programs and applications targeted to patients. We discuss some of the future opportunities for extending the generalization of our research findings under the “Future Research” section below.
Subpopulation Considerations
Although many treatment alternatives are available for individuals with diabetes, a substantial number of patients are still unable to achieve their glycemic target goals. There are few studies quantifying the magnitude of HTE and the associated characteristics of patients explaining variability in treatment response. Diabetes standards of care set HbA1c therapeutic target goals at <7.0% (ADA) or <6.5% (AACE and ACE). These goals are based primarily on RCT data using estimates derived from nonstratified, unadjusted, treatment-specific HbA1c end-point means that typically do not consider subpopulation outcomes. However, 2016-2020 guidelines and recommendations from the ADA, AACE, and ACE have emphasized tailoring HbA1c target goals to individual patient characteristics that incorporate patient preferences, education, and cultural factors. Our research used 2 secondary data sources, pooled RCTs and EHRs, to develop models in which multivariable adjustments for baseline HbA1c, BMI, age, sex, current treatment, duration of diabetes, race/ethnicity, income, education, and patient-reported QOL and SAT scores were used to investigate potential factors associated with response variability in order to adjust HbA1c target goals accordingly for subpopulations. Outcomes of HbA1c <8.0% and <7.0% were modeled, and the resulting estimators were used to develop user-friendly benchmarking calculators in the context of case-based learning examples. Given that the primary goal of this study was to personalize and benchmark therapeutic target goals and outcomes by subpopulations using data such as age, sex, race/ethnicity, income, educational and behavioral characteristics, QOL, and SAT, the subgroups that have not been investigated are those defined by variables other than those available from our RCT and EHR data sources.
To be clear, the regression models estimated the β coefficients associated with the diabetes treatments, PROs, and sociodemographic variables in order to estimate the HbA1C end-point outcomes for those individuals who shared common characteristics using past clinical performance data from either RCTs or EHRs. As such, our method provides case-mix adjustment when caregivers need to benchmark their performance against either high-quality or usual-care performance standards. The modeling in our study was not used for “prediction” purposes to compare the efficacy of different treatments to decide which treatment would be best for an individual patient going forward.
Study Limitations
External validity is both a weakness and a strength of this study. The data used for development of the analytics models were limited to the available clinical trials that included comprehensive assessments of QOL, patient satisfaction, demographics, and socioeconomic factors. As such, newer diabetes classes, such as the SGLT2s, were not included; however, as mentioned previously, we have recently added 2 clinical trials using the combination of SGLT and DPP-4 when added to metformin. In addition, the EHRs were obtained solely from the Partners HealthCare EHR systems. This database primarily reflects the population in the Health and Human Services Region 1 (New England), and of this population, individuals who are predominantly from Massachusetts. In the future, additional databases could be used for greater generalizability as described in the “Future Research” section below. In addition, the selection criteria for inclusion in the EHR portion of the study were restricted to patients who had at least 2 notes in their medical record at least 1 year apart and who had a baseline HbA1c and at least 1 follow-up measurement. As such, it should be made clear that this sample was a convenience sample and not a representative sample of all individuals with diabetes who had an encounter with the Partners HealthCare system and did not meet these selection criteria. On the other hand, the external validity of the Partners-based data is quite high, because the data are from a large number of practices.
Although the purpose of this study was not to compare the efficacy of different treatments to predict which treatment would be best for an individual patient, but rather to estimate the ability of the patient to achieve success (ie, meeting the HbA1c target), some factors may not have been included in the adjustment. Besides baseline HbA1c and age, the diabetes treatment was the primary contributor to heterogeneity among patients in terms of reaching their target goal. It is necessary to hold treatment constant when comparing the individual’s probability of success under their current care vs either the high-benchmark reference or the usual-care benchmark reference. However, when using the data from EHRs, unmeasured factors that are related to treatment choice and to the outcome might reduce the validity of the comparative benchmark.
Quality-of-care health administrators and managers are continually looking for ways to evaluate and improve their health care systems processes and outcomes. In the context of our study, it is important that they understand that although they may use the terms estimates, projections, predictions, and forecasts interchangeably, for statistical modeling, it is extremely important to distinguish between these terms. The estimates of the HbA1c outcomes for either individual or diabetes subgroup patient profiles are based on existing demographic, socioeconomic, and patient-reported characteristics as well as outcomes, whether derived from logistic, Cox, or linear regressions. These estimates should not be confused with projections or forecasts for individual patients or subgroups of patients. The estimates of the success or failure to meet the HbA1c target are indirect measures of a present or past condition that can be measured directly, as was done in our study. In contrast, a projection or prediction is a conditional statement about the future. Projections are calculations of future conditions that would exist as a result of adopting a set of underlying assumptions. A forecast is a judgmental statement of what the analyst believes to be the most likely future. Using estimates to describe one’s patient population so as to develop a reference benchmark or to compare with a reference benchmark does not constitute a projection or forecast. Also, there is often conflating of the terms prediction and estimation in medical decision-making. However, there is a sharp distinction between the 2 terms in the standard model of a statistical problem. An estimator uses data to guess at a parameter, whereas a predictor uses the data to guess at some random value that is not part of the data set. Estimation is always used for determining unknown parameters, whereas prediction is used for random variables. However, there is no difference in the regression models used. Prediction uses sample regression function to estimate a value for the dependent variable conditioned on some unobserved values of the independent variable, whereas estimation is the process or technique of calculating an unknown parameter or quantity of the population. Predictive models require validation and calibration, as discussed by Wasson et al,126 whereas estimation for benchmarking is ascertained from past performance data and does not involve validation or calibration.
However, model assumptions are still of concern when comparisons are being made. Health outcome high-quality benchmarks are estimated from population data representative of a health care practice assumed to yield the best possible health outcome. To ascertain how well any practice compares with this high-quality benchmark, one must use a statistical model to control for population differences in clinical, demographic, and socioeconomic variables between the high-quality and the comparison clinical practice. The selection of the case-mix adjustment model is optimized for a particular study population that might not be suitable for the comparison population. To carry out a valid comparison, the subgroup’s clinical, demographic, and socioeconomic characteristics should not be too different from each other, and the variables chosen in the model should have relatively comparable influences on the HbA1c dependent variable. For example, if a high-quality benchmark is derived using a population that is 90% White, 80% college graduates, and 95% employed, it would not be appropriate to use that model when comparing a population that has a substantially different case mix as measured using these variables. Although we did not carry out the validation and calibration methods that would be necessary to ascertain meeting the assumptions of relatively similar case mix, it is important to note that validation and calibration are methodologically important when using benchmarking methods in practice.
Future Research
There are several potential areas where future research will be applicable. The most promise lies in using and expanding the database sources amenable to patient-centered personalized therapeutic profiles. When our study was first designed and proposed in 2012, there were few opportunities for sharing RCT data or large structured electronic research databases. Since its inception 9 years ago, the FDA Sentinel system is now fully functional, and as of January 2018, it stored data from 310.8 million cumulative patient identifiers between 2000 and 2018, as well as 668 million person-years of observation time, 70.1 million people currently accruing new data, 11.7 pharmacy dispensings, 15 billion unique encounters, and 48.5 million people with >1 laboratory test result.127 The NIH Collaboratory Distributed Research Network allows for future collaboration and data sharing to reuse and leverage the work of the Sentinel project. The National Patient-Centered Clinical Research Network (PCORnet)128 is also a database that would be amenable to the types of methods applied here. Looking further into the future, the Precision Medicine Initiative longitudinal “cohort” of ≥1 million Americans will be asked to give consent for extensive characterization of biological specimens (cell populations, proteins, metabolites, RNA, and DNA, including whole-genome sequencing, when costs permit) and behavioral data, all linked to their EHRs. Because researchers will be able to apply for access to the cohort’s data, it would be possible to extend the methods used here to these new cohorts. It is the intent of this Precision Medicine Initiative to “enable observational studies of drugs and devices and potentially prompt more rigorous interventional studies that address specific questions.”119 Our research findings and methods for benchmarking and personalizing diabetes target goals would be particularly suited to this initiative.
Another area that we consider important is continuing our work in dissemination and implementation of results. Benchmark calculators were used as an aid to help clinicians gain an understanding of the potential utility of our research results. The online calculators were field tested and optimized within case-based learning exercises allowing the user to simultaneously modify patient characteristics to explore and visualize how individual patient profiles might influence the probability of reaching target glycemic goals. A social media interactive learning collaborative was developed for dissemination after the project end for sustainability and to help continue to translate diabetes patient-centered research findings into clinical practice, while providing a novel approach to competency-based training on ADA and AACE guidelines.
Conclusions
This research project evaluated the relationships between clinical, demographic, socioeconomic, psychosocial, and QOL patient characteristics, and diabetes HTE. We used data from pooled RCTs to estimate the best-practice benchmarks for the probability of reaching HbA1c target goals and from EHRs to estimate the usual-care benchmarks. The study results demonstrated how benchmarking metrics could provide practical feedback to physicians regarding reaching HbA1c goals relative to patients with similar characteristics compared with either benchmarked best-practice or usual-care references. The results also indicated substantial differences in patients achieving HbA1c goals based on their diabetes treatment and their clinical, demographic, socioeconomic, and patient-reported characteristics. Side effects and symptom distress, psychological distress, well-being, and satisfaction were significantly associated with a patient’s ability to achieve HbA1c targets. Benchmarking models could help physicians recognize gaps between their practice’s HbA1c outcomes and what is achievable in an HBP setting. They could also assure physicians that given their practice’s case mix, they are still achieving high-quality care even though not all patients reach the same HbA1c target.
The probabilities derived from the benchmarking models have 2 main uses. The first use helps inform an individual’s treatment plan by estimating the patient’s probability of achieving HbA1c goals. The second aggregates patients with similar characteristics and corresponding probabilities into subgroup cohorts to longitudinally measure the rates of achieving HbA1c goals across the practice, and to compare these rates with those of benchmark practices. Distinguishing between the delivery of health care, nonmodifiable patient characteristics, and modifiable factors also could be crucial. For example, if a patient presents with high levels of diabetes distress and anxiety, mental health programs might help them achieve their HbA1c goals. Rather than requiring a single HbA1c target value for all patients that is potentially unachievable for many patients, quality-of-care programs and metrics should provide clinicians with information that supports innovative ways to improve diabetes outcomes along a continuum of care with incremental steps that include tailoring individualized achievable HbA1c goals linked to personalized diabetes management strategies.
References
- 1.
- American Diabetes Association. 1. Strategies for improving care: Standards of Medical Care in Diabetes—2016. Diabetes Care. 2016;39(Suppl 1):S6-S12. [PubMed: 26696683]
- 2.
- American Diabetes Association. 1. Promoting health and reducing disparities in populations: Standards of Medical Care in Diabetes—2017. Diabetes Care. 2017;40(Suppl 1):S6-S10. [PubMed: 27979888]
- 3.
- American Diabetes Association. 5. Lifestyle management: Standards of Medical Care in Diabetes—2019. Diabetes Care. 2019;42(Suppl 1):S46-S60. [PubMed: 30559231]
- 4.
- American Diabetes Association. 5. Facilitating behavior change and well-being to improve health outcomes: Standards of Medical Care in Diabetes—2020. Diabetes Care. 2020;43(Suppl 1):S48-S65. [PubMed: 31862748]
- 5.
- Garber AJ, Abrahamson MJ, Barzilay JI, et al. Consensus Statement by the American Association of Clinical Endocrinologists and American College of Endocrinology on the comprehensive type 2 diabetes management algorithm—2017 executive summary. Endocr Pract. 2017;23(2):207-238. [PubMed: 28095040]
- 6.
- Garber AJ, Abrahamson MJ, Barzilay JI, et al. Consensus Statement by the American Association of Clinical Endocrinologists and American College of Endocrinology on the comprehensive type 2 diabetes management algorithm—2019 executive summary. Endocr Pract. 2019;25(1):69-100. [PubMed: 30742570]
- 7.
- Harris SB, Cheng AYY, Davies MJ, Gerstein HC, Green JB, Skolnik N. Person-Centered, Outcomes-Driven Treatment: A New Paradigm for Type 2 Diabetes in Primary Care. American Diabetes Association; 2019. Accessed February 24, 2021. https://professional.diabetes.org/sites/professional.diabetes.org/files/media/ada_cvd-renalcompendium_fin-web.pdf [PMC free article: PMC559432] [PubMed: 32667763]
- 8.
- American Diabetes Association. 6. Glycemic targets: Standards of Medical Care in Diabetes—2020. Diabetes Care. 2020;43(Suppl 1):S66-S76. [PubMed: 31862749]
- 9.
- National Committee on Quality Assurance. Diabetes recognition programs. 2020. Accessed January 31, 2017. https://www
.ncqa.org /programs/health-care-providers-practices /diabetes-recognition-program-drp/ - 10.
- Rosenthal MB, Dudley RA. Pay-for-performance: will the latest payment trend improve care? JAMA. 2007;297(7):740-744. [PubMed: 17312294]
- 11.
- Chen JY, Tian H, Juarez DT, et al. The effect of a PPO pay-for-performance program on patients with diabetes. Am J Manag Care. 2010;16(1):e11-e19. Accessed February 24, 2021. https://www
.ajmc.com /view/ajmc_2010janchenweb_e11t_e19 [PubMed: 20059287] - 12.
- National Committee for Quality Assurance. Health Plan Employer Data and Information Set: Comprehensive Diabetes Care (CDC) measures. 2020. Accessed October 1, 2018. https://www
.ncqa.org /hedis/measures/comprehensive-diabetes-care - 13.
- Rodbard HW, Jellinger PS, Davidson JA, et al. Statement by an American Association of Clinical Endocrinologists/American College of Endocrinology consensus panel on type 2 diabetes mellitus: an algorithm for glycemic control. Endocr Pract. 2009;15(6):540-559. [PubMed: 19858063]
- 14.
- Chou SC, Palmer RH, Ezhuthachan S, et al. Management of hyperbilirubinemia in newborns: measuring performance by using a benchmarking model. Pediatrics. 2003;112(6 Pt 1):1264-1273. [PubMed: 14654595]
- 15.
- Hermans MP, Elisaf M, Michel G, et al. Benchmarking is associated with improved quality of care in type 2 diabetes: the OPTIMISE randomized, controlled trial. Diabetes Care. 2013;36(11):3388-3395. [PMC free article: PMC3816864] [PubMed: 23846810]
- 16.
- Nicolucci A, Rossi MC, Pellegrini F, et al. Benchmarking network for clinical and humanistic outcomes in diabetes (BENCH-D) study: protocol, tools, and population. SpringerPlus. 2014;3:83. doi: 10.1186/2193-1801-3-83 [PMC free article: PMC3935032] [PubMed: 24600541] [CrossRef]
- 17.
- Pintaudi B, Lucisano G, Gentile S, et al; BENCH-D Study Group. Correlates of diabetes-related distress in type 2 diabetes: findings from the benchmarking network for clinical and humanistic outcomes in diabetes (BENCH-D) study. J Psychosom Res. 2015;79(5):348-354. [PubMed: 26526307]
- 18.
- Charalampopoulos D, Hermann JM, Svensson J, et al. Exploring variation in glycemic control across and within eight high-income countries: a cross-sectional analysis of 64,666 children and adolescents with type 1 diabetes. Diabetes Care. 2018;41(6):1180-1187. [PMC free article: PMC5961394] [PubMed: 29650804]
- 19.
- Lee AS, Colagiuri S, Flack JR. Successful implementation of diabetes audits in Australia: the Australian National Diabetes Information Audit and Benchmarking (ANDIAB) initiative. Diabet Med. 2018;35(7):929-936. [PubMed: 29633347]
- 20.
- Julin B, Willers C, Leksell J, et al. Association between sociodemographic determinants and health outcomes in individuals with type 2 diabetes in Sweden. Diabetes Metab Res Rev. 2018;34(4):e2984. doi:10.1002/dmrr.2984 [PubMed: 29377503] [CrossRef]
- 21.
- Bennett WL, Wilson LM, Bolen S, et al. Oral Diabetes Medications for Adults with Type 2 Diabetes: An Update. Report No.: 11-EHC038-EF. Agency for Healthcare Research and Quality; 2011. Accessed January 31, 2017. https://www
.ncbi.nlm .nih.gov/books/NBK55754/ [PubMed: 21735563] - 22.
- Bolen S, Tseng E, Hutfless S, et al. Diabetes Medications for Adults with Type 2 Diabetes: an Update. Comparative Effectiveness Review No. 173. Report No.: 16-EHC013-EF. Agency for Healthcare Research and Quality; 2016. Accessed January 31, 2017. https://www
.ncbi.nlm .nih.gov/books/NBK362863/ [PubMed: 27227214] - 23.
- Smith RJ, Nathan DM, Arslanian SA, Groop L, Rizza RA, Rotter JI. Individualizing therapies in type 2 diabetes mellitus based on patient characteristics: what we know and what we need to know. J Clin Endocrinol Metab. 2010;95(4):1566-1574. [PMC free article: PMC5393381] [PubMed: 20194712]
- 24.
- Del Prato S, LaSalle J, Matthaei S, Bailey CJ; Global partnership for effective diabetes management. Tailoring treatment to the individual in type 2 diabetes practical guidance from the Global Partnership for Effective Diabetes Management. Int J Clin Pract. 2010;64(3):295-304. [PMC free article: PMC2814090] [PubMed: 20456169]
- 25.
- Pozzilli P, Leslie RD, Chan J, et al. The A1c and ABCD of glycaemia management in type 2 diabetes: a physician’s personalized approach. Diabetes Metab Res Rev. 2010;26(4):239-244. [PubMed: 20503255]
- 26.
- US Food and Drug Administration. Paving the Way for Personalized Medicine: FDA’s Role in a New Era of Medical Product Development. Published October 2013. Accessed January 31, 2017. https://www
.fdanews.com /ext/resources/files /10/10-28-13-Personalized-Medicine .pdf - 27.
- Morris AP, Voight BF, Teslovich TM, et al. Large-scale association analysis provides insights into the genetic architecture and pathophysiology of type 2 diabetes. Nat Genet. 2012;44(9):981-990. [PMC free article: PMC3442244] [PubMed: 22885922]
- 28.
- Hamburg MA, Collins FS. The path to personalized medicine. N Engl J Med. 2010;363:301-304. [PubMed: 20551152]
- 29.
- Kleinberger JW, Pollin TI. Personalized medicine in diabetes mellitus: current opportunities and future prospects. Ann N Y Acad Sci. 2015;1346(1):45-56. [PMC free article: PMC4480162] [PubMed: 25907167]
- 30.
- Sethu S, Reddy K. Evolving to personalized medicine for type 2 diabetes. Endocrinol Metab Clin North Am. 2016;45(4):1011-1020. [PubMed: 27823602]
- 31.
- Agardh E, Allebeck P, Hallqvist J, Moradi T, Sidorchuk A. Type 2 diabetes incidence and socio-economic position: a systematic review and meta-analysis. Int J Epidemiol. 2011;40(3):804-818. [PubMed: 21335614]
- 32.
- Dwyer-Lindgren L, Mackenbach JP, van Lenthe FJ, Flaxman AD, Mokdad AH. Diagnosed and undiagnosed diabetes prevalence by county in the US, 1999-2012. Diabetes Care. 2016;39(9):1556-1562. [PubMed: 27555622]
- 33.
- Ismail-Beigi F, Moghissi E, Tiktin M, Hirsch IB, Inzucchi SE, Genuth S. Individualizing glycemic targets in type 2 diabetes mellitus: implications of recent clinical trials. Ann Intern Med. 2011;154(8):554-559. [PubMed: 21502652]
- 34.
- Laiteerapong N, John PM, Nathan AG, Huang ES. Public health implications of recommendations to individualize glycemic targets in adults with diabetes. Diabetes Care. 2013;36(1):84-89. [PMC free article: PMC3526201] [PubMed: 22961575]
- 35.
- Cantrell RA, Alatorre CI, Davis EJ, et al. A review of treatment response in type 2 diabetes: assessing the role of patient heterogeneity. Diabetes Obes Metab. 2010;12(10):845-857. [PubMed: 20920036]
- 36.
- Kaplan SH, Billimek J, Sorkin DH, Ngo-Metzger Q, Greenfield S. Who can respond to treatment? Identifying patient characteristics related to heterogeneity of treatment effects. Med Care. 2010;48(6 Suppl):S9-S16. [PMC free article: PMC5769476] [PubMed: 20473205]
- 37.
- Hardin DS, Rohwer RD, Curtis BH, et al. Understanding heterogeneity in response to antidiabetes treatment: a post hoc analysis using SIDES, a subgroup identification algorithm. J Diabetes Sci Technol. 2013;7(2):420-430. [PMC free article: PMC3737644] [PubMed: 23567001]
- 38.
- Kaplan SH, Billimek J, Sorkin DH, Ngo-Metzger Q, Greenfield S. Reducing racial/ethnic disparities in diabetes: the Coached Care (R2D2C2) project. J Gen Intern Med. 2013;28(10):1340-1349. [PMC free article: PMC3785664] [PubMed: 23645452]
- 39.
- Nathan DM, Buse JB, Kahn SE, et al; GRADE Study Research Group. Rationale and design of the glycemia reduction approaches in diabetes: a comparative effectiveness study (GRADE). Diabetes Care. 2013;36(8):2254-2261. [PMC free article: PMC3714493] [PubMed: 23690531]
- 40.
- Wexler DJ, Krause-Steinrauf H, Crandall JP, et al. Baseline characteristics of randomized participants in the Glycemia Reduction Approaches in Diabetes: A Comparative Effectiveness Study (GRADE). Diabetes Care. 2019;42(11):2098-2107. [PMC free article: PMC6804613] [PubMed: 31391203]
- 41.
- Rand Health. RAND 36-Item Health Survey 1.0 Questionnaire. RAND Corporation. Accessed June 30, 2018. https://www
.rand.org /health/surveys_tools /mos/36-item-short-form.html - 42.
- Kaplan RM, Bush JW, Berry CC. Health status: types of validity and the index of well-being. Health Serv Res. 1976;11(4):478-507. [PMC free article: PMC1071947] [PubMed: 1030700]
- 43.
- Bradley C, Gamsu DS. Guidelines for encouraging psychological well-being: report of a Working Group of the World Health Organization Regional Office for Europe and International Diabetes Federation European Region St Vincent Declaration Action Programme for Diabetes. Diabet Med. 1994;11(5):510-516. [PubMed: 8088133]
- 44.
- Bradley C. Diabetes treatment satisfaction questionnaire. Change version for use alongside status version provides appropriate solution where ceiling effects occur. Diabetes Care. 1999;22(3):530-532. [PubMed: 10097946]
- 45.
- Testa MA. Benchmarking the comparative effectiveness of diabetes treatments using patient-reported outcomes and socio-demographic factors. Harvard T.H. Chan School of Public Health. Accessed January 31, 2017. https://www.hsph.harvard.edu/comparative-effectiveness-of-diabetes-treatments/
- 46.
- Testa MA. Benchmarking the comparative effectiveness of diabetes treatments using patient-reported outcomes and socio-demographic factors. PCORI. Approved September 2013. Accessed January 31, 2017. http://www
.pcori.org /research-results/2013 /benchmarking-comparative-effectiveness-diabetes-treatments-using-patient - 47.
- Testa MA, Simonson DC. Development of a personalized, patient-centered glycemic control benchmarking tool for T2DM. Diabetes. 2014;62(Suppl 1):A303, 1165-P.
- 48.
- Testa MA, Turchin A, Sosina OA, Simonson DC. Benchmarking T2DM treatment effectiveness in clinical practice. Diabetes. 2015;64(Suppl 1):A346, 1330-P.
- 49.
- Simonson DC, Marc LG, Testa MA. Health education internet use by persons with diabetes in the US. Diabetes. 2015;64(Suppl 1):A159, 770-P.
- 50.
- Testa MA, Saldivar-Salazar A, Savoia E, Simonson D. Using social media crowdsourced data for diabetes patient-centered outcomes research (PCOR). Diabetes. 2015;64(Suppl 1):A55, 220-OR.
- 51.
- Testa MA, Su, M, Turchin A, Simonson DC. Patient-centered outcomes and socio-demographic predictors of diabetes treatment effectiveness. Diabetes. 2016;65(Suppl 1):A254, 1235-P.
- 52.
- Testa MA, Marc LG, Turchin A, Simonson DC. Impact of subclinical depression and anxiety on intensity and frequency of treatment-related side effects and symptoms. Diabetes. 2016;65(Suppl 1):A211, 823-P.
- 53.
- Turchin A, Sosina O, Zhang H, et al. Effect of medication reconciliation on emergency department visits and hospitalizations in patients with diabetes. Diabetes. 2016;65(Suppl 1):A319, 1216-P.
- 54.
- Zhang H, Hosomura N, Shubina M, Simonson DC, Testa MA, Turchin A. Electronic documentation of lifestyle counseling in primary care is associated with lower risk of cardiovascular events in patients with diabetes. Diabetes. 2016;65(Suppl 1):A363, 1387-P.
- 55.
- Testa MA, Saldivar-Salazar S, Su M, Marc LG, Simonson DC. A social media learning collaborative approach to competency-based training in diabetes. Diabetes. 2017;67(Suppl 1):A97, 368-OR.
- 56.
- Testa MA, Turchin A, Su M, Simonson DC. Impact of patient-centered factors on comparative effectiveness of initial therapies in treatment-naïve T2D. Diabetes. 2018;67(Suppl 1):1520-P, A407.
- 57.
- Testa MA, Su M, Simonson DC. Modeling pathways between psychological distress, emergent symptoms and side effects, and endpoint A1c during treatment. Diabetes. 2019;68(Suppl 1):850-P.
- 58.
- McDonnell ME. Diabetes 2.0 – Next-Generation Approach to Diagnosis and Treatment Video – Brigham and Women’s. February 25, 2015. Accessed February 24, 2021. https://youtu
.be/XlL6Cbgidk4 - 59.
- Phase V Technologies. Treatment Satisfaction modules. Accessed January 31, 2017. http://phasevtech.com/services/treatmentsatisfaction.html
- 60.
- Testa MA, Simonson DC. Health economic benefits and quality of life during improved glycemic control in patients with type 2 diabetes mellitus: a randomized controlled, double-blind trial. JAMA. 1998;280(17):1490-1496. [PubMed: 9809729]
- 61.
- Testa MA, Simonson DC. Satisfaction and quality of life with premeal inhaled versus injected insulin in adolescents and adults with type 1 diabetes. Diabetes Care. 2007;30(6):1399-1405. [PubMed: 17337493]
- 62.
- Bode B, Testa MA, Magwire M, et al. Patient-reported outcomes following treatment with the human GLP-1 analogue liraglutide or glimepiride in monotherapy: results from a randomized controlled trial in patients with type 2 diabetes. Diabetes Obes Metab. 2010;12(7):604-612. [PMC free article: PMC2901519] [PubMed: 20590735]
- 63.
- Quattrin T, Bélanger A, Bohannon NJ, Schwartz SL; Exubera Phase III Study Group. Efficacy and safety of inhaled insulin (Exubera) compared with subcutaneous insulin therapy in patients with type 1 diabetes: results of a 6-month, randomized, comparative trial. Diabetes Care. 2004;27(11):2622-2627. [PubMed: 15504996]
- 64.
- Horton ES, Clinkingbeard C, Gatlin M, Foley J, Mallows S, Shen S. Nateglinide alone and in combination with metformin improves glycemic control by reducing mealtime glucose levels in type 2 diabetes. Diabetes Care. 2000;23(11):1660-1665. [PubMed: 11092289]
- 65.
- US Food and Drug Administration. Drug approval package. Starlix (nateglinide) tablets. Novartis Pharmaceuticals Corporation Application No.: 21-204. Approval date: December 22, 2000. Accessed March 1, 2021. http://www
.accessdata .fda.gov/drugsatfda_docs /nda/2000/21-204_Stsrlix.cfm - 66.
- Skyler JS, Weinstock RS, Raskin P, et al; Inhaled Insulin Phase III Type 1 Diabetes Study Group. Use of inhaled insulin in a basal/bolus insulin regimen in type 1 diabetic subjects: a 6-month, randomized, comparative trial. Diabetes Care. 2005;28(7):1630-1635. [PubMed: 15983312]
- 67.
- Raskin P, Bode BW, Marks JB, et al. Continuous subcutaneous insulin infusion and multiple daily injection therapy are equally effective in type 2 diabetes: a randomized, parallel-group, 24-week study. Diabetes Care. 2003;26(9):2598-2603. [PubMed: 12941725]
- 68.
- Hollander PA, Blonde L, Rowe R, et al. Efficacy and safety of inhaled insulin (Exubera) compared with subcutaneous insulin therapy in patients with type 2 diabetes: results of a 6-month, randomized, comparative trial. Diabetes Care. 2004;27(10):2356-2362. [PubMed: 15451900]
- 69.
- Kourides I, Blonde L, Chaiken R. Patients with type 2 diabetes treated with glipizide GITS and acarbose alone and in combination. Diabetes. 2003;52(Suppl 1):A450.
- 70.
- Rosenstock J, Zinman B, Murphy LJ, et al. Inhaled insulin improves glycemic control when substituted for or added to oral combination therapy in type 2 diabetes: a randomized, controlled trial. Ann Intern Med. 2005;143(8):549-558. [PubMed: 16230721]
- 71.
- DeFronzo RA, Bergenstal RM, Cefalu WT, et al; Exubera Phase III Study Group. Efficacy of inhaled insulin in patients with type 2 diabetes not controlled with diet and exercise: a 12-week, randomized, comparative trial. Diabetes Care. 2005;28(8):1922-1928. [PubMed: 16043733]
- 72.
- Cefalu WT, Schneider DJ, Carlson HE, et al. Effect of combination glipizide GITS/metformin on fibrinolytic and metabolic parameters in poorly controlled type 2 diabetic subjects. Diabetes Care. 2002;25(12):2123-2128. [PubMed: 12453948]
- 73.
- US Food and Drug Administration. Drug approval package. Exubera (insulin human [rDNA origin] inhalation powder) and Exubera inhaler. Pfizer Global Research & Development. NDA: 021868. Approval date: January 27, 2006. Accessed March 1, 2021. http://www
.accessdata .fda.gov/drugsatfda_docs /nda/2006/021868_exubera_toc.cfm - 74.
- Testa MA, Turner RR, Hayes JF, Scranton RE, Simonson DC. Satisfaction and quality of life with sulfonylurea plus either metformin or Exubera: an international randomized phase 3 trial. Diabetes. 2004;53(Suppl 2):A115.
- 75.
- Testa MA. Glipizide GITS in managed care. Data on file. Phase V Technologies, Inc. http://phasevtech.com/contactus.html
- 76.
- Testa MA, Hayes JF, Turner RR, Simonson DC. Quality of life improvements in type 2 diabetes when Exubera is added after failure on metformin monotherapy: an international randomized Phase 3 trial. Diabetes. 2004;53(Suppl 2):A437.
- 77.
- Testa MA. Placebo controlled fixed dose clinical trials of PTP-112. Data on file. Phase V Technologies, Inc. http://phasevtech.com/contactus.html
- 78.
- Pfizer/Sanofi. Demonstrate Exubera works as well as Avandia when added to sulfonylurea + metformin in controlling glucose. Accessed January 15, 2017. ClinicalTrials.gov identifier: NCT00150410. https:
//clinicaltrials .gov/ct2/show/NCT00150410?titles =Demonstrate+Exubera+Works+as+Well+As+Avandia+when+Added+To+Sulfonylurea+ %2B+Metformin+in+Controlling+Glucose .&draw=2&rank=1 - 79.
- Testa MA, Gill J, Su M, Turner RR, Blonde L, Simonson DC. Comparative effectiveness of basal-bolus versus premix analogue insulin on glycemic variability and patient-centered outcomes during insulin intensification in type 1 and type 2 diabetes: a randomized, controlled, cross-over trial. J Clin Endocrinol Metab. 2012;97(10):3504-3514. [PubMed: 22851487]
- 80.
- Jean-Louis L. Insulin glargine plus insulin glulisine multiple daily injections (MDI) versus premix insulin treatment in subjects with diabetes mellitus (type 1 or type 2). ClinicalTrials.gov identifier: NCT00135941. Updated June 4, 2009. https:
//clinicaltrials .gov/ct2/show/NCT00135941 - 81.
- US Food and Drug Administration. Drug approval package. Victoza (liraglutide [rDNA]) injection. Novo Nordisk Inc. Application No.: 022341; Approval Date: 1/25/2010. Accessed March 1, 2021. http://www
.accessdata .fda.gov/drugsatfda_docs /nda/2010/022341s000TOC.cfm - 82.
- Blonde L, Guthrie RD, Testa MA, O’Brien, TW, Zimmerman RS, Sandberg MI. Diabetes management by a team of diabetes nurse educators, endocrinologists and primary care physicians in a managed care environment. Diabetes. 1999;48(Suppl 1):A198.
- 83.
- Testa MA, Blonde l, Hayes JF, Simonson DC. Symptom and psychological mediators of improved quality of life (QOL) during stages diabetes management (SDM) versus usual care (UC) in type 2 diabetes. Diabetes. 1999;48(Suppl 1):A9.
- 84.
- Blumenthal D, Tavenner M. The “meaningful use” regulation for electronic health records. N Engl J Med. 2010;363(6):501-504. [PubMed: 20647183]
- 85.
- Marcotte L, Seidman J, Trudel K, et al. Achieving meaningful use of health information technology: a guide for physicians to the EHR incentive programs. Arch Intern Med. 2012;172(9):731-736. [PubMed: 22782203]
- 86.
- Fox S, Duggan M. Tracking for health. Pew Research Center. Published January 28, 2013. Accessed January 31, 2017. http://www
.pewinternet .org/2013/01/28/tracking-for-health/ - 87.
- Fox S, Duggan M. Health online 2013. Pew Research Center. Published January 15, 2013. Accessed January 31, 2017. http://www
.pewinternet .org/2013/01/15/health-online-2013/ - 88.
- Mazze RS, Etzwiler DD, Strock E, et al. Staged diabetes management. Toward an integrated model of diabetes care. Diabetes Care. 1994;17(Suppl 1):56-66. [PubMed: 8088226]
- 89.
- Vilsbøll T, Ekholm E, Johnsson EK, Donamraju, Jabbour S, Lind M. Dapagliflozin plus saxagliptin shows noninferior A1c reduction vs. insulin glargine in patients with type 2 diabetes inadequately controlled by metformin with or without sulfonylurea. Diabetes. 2018;67(Suppl 1):260-OR, A70.
- 90.
- Frias JP, Gonsaliz-Galvez G, Johnsson EK, Maaske J, Peters AM. Dapagliflozin plus saxagliptin add-on vs. glimepiride add-on to metformin in patients with poorly controlled type 2 diabetes. Diabetes. 2018;67(Suppl 1):261-OR, A70.
- 91.
- Phase V Health Outcomes Information System. Quality-of-life assessments: core quality-of-life modules. Phase V Technologies; 2019. Accessed January 31, 2017. http://phasevtech.com/services/qualityoflife.html and http://www
.phasevtechnologies .com/services/life.php - 92.
- Testa MA, Hollenberg NK, Anderson RA, Williams GH. Assessment of quality of life by patient and spouse during antihypertensive therapy with atenolol and nifedipine GITS. Am J Hypertens. 1991;4(4 Pt 1):363-373. [PubMed: 2059396]
- 93.
- Testa MA, Anderson RB, Nackley JF, Hollenberg NK. Quality of life and antihypertensive therapy in men: a comparison of captopril with enalapril. N Engl J Med. 1993;328(13):907-913. [PubMed: 8446137]
- 94.
- Testa MA, Turner RR, Simonson DC, Krafcik MB, Calvo C, Luque-Otero M. Quality of life and calcium channel blockade with nifedipine GITS versus amlodipine in hypertensive patients in Spain. J Hypertens (Greenwich). 1998;16(12 Pt 1):1839-1847. [PubMed: 9869019]
- 95.
- Testa MA, Simonson DC, Turner RR. Valuing quality of life and improvements in glycemic control in people with type 2 diabetes. Diabetes Care. 1998;21(Suppl 3):C44-C52. [PubMed: 9850489]
- 96.
- Anderson RB, Testa MA. Symptom distress checklists as a component of quality-of life measurement: comparing prompted reports by patient and physician with concurrent adverse event reports via the physician. Drug Inf J. 1994;28(1):89-114.
- 97.
- Testa MA, Turner RR, Simonson DC. Effects of lower blood glucose and reduced inter-day glucose variability on quality of life in type 2 diabetes. Diabetes. 2003;52(Suppl 1):A420.
- 98.
- Testa MA, Hayes JF, Turner RR, Simonson DC. Improved quality of life is associated with improved glycemic control in type 2 diabetes: an international, multi-cultural, multi-center, placebo-control clinical trial. Diabetes. 2000;49(Suppl 1):A73.
- 99.
- Testa MA. Quality-of-life assessment in diabetes research: interpreting the magnitude and meaning of treatment effects. Diabetes Spectr. 2000;13:36-41.
- 100.
- Turchin A. Canary: a user friendly information extraction tool. Brigham and Women’s Hospital. Accessed July 1, 2018. http://canary
.bwh.harvard.edu/ - 101.
- Herman WH. The global agenda for the prevention of type 2 diabetes. Nutr Rev. 2017;75(Suppl 1):13-18. [PMC free article: PMC5207005] [PubMed: 28049746]
- 102.
- World Health Organization. Global Report on Diabetes. 2016. Accessed January 31, 2017. http://apps
.who.int/iris /bitstream/10665 /204871/1/9789241565257_eng .pdf?ua=1&ua=1 - 103.
- US Diabetes Surveillance System. Diagnosed diabetes. Centers for Disease Control and Prevention. Accessed January 31, 2017. https://gis
.cdc.gov/grasp /diabetes/DiabetesAtlas.html# - 104.
- Canedo JR, Miller ST, Schlundt D, Fadden MK, Sanderson M. Racial/ethnic disparities in diabetes quality of care: the role of healthcare access and socioeconomic status. J Racial Ethn Health Disparities. 2018;5(1):7-14. [PMC free article: PMC5505804] [PubMed: 28078660]
- 105.
- Bolo NR, Musen G, Simonson DC, et al. Functional connectivity of insula, basal ganglia, and prefrontal executive control networks during hypoglycemia in type 1 diabetes. J Neurosci 2015;35(31):11012-11023. [PMC free article: PMC4524972] [PubMed: 26245963]
- 106.
- Marder TJ, Flores VL, Bolo NR, et al. Task-induced brain activity patterns in type 2 diabetes: a potential biomarker for cognitive decline. Diabetes. 2014;63(9):3112-3119. [PMC free article: PMC4141362] [PubMed: 24705405]
- 107.
- Petrak F, Baumeister H, Skinner TC, Brown A, Holt RI. Depression and diabetes: treatment and health-care delivery. Lancet Diabetes Endocrinol. 2015;3(6):472-485. [PubMed: 25995125]
- 108.
- Brown SA, García AA, Brown A, et al. Biobehavioral determinants of glycemic control in type 2 diabetes: a systematic review and meta-analysis. Patient Educ Couns. 2016;99(10):1558-1567. [PMC free article: PMC5028237] [PubMed: 27036083]
- 109.
- Walz L, Pettersson B, Rosenqvist U, Deleskog A, Journath G, Wändell P. Impact of symptomatic hypoglycemia on medication adherence, patient satisfaction with treatment, and glycemic control in patients with type 2 diabetes. Patient Prefer Adherence. 2014;8:593-601. [PMC free article: PMC4011898] [PubMed: 24812495]
- 110.
- Pollack MF, Purayidathil FW, Bolge SC, Williams SA. Patient-reported tolerability issues with oral antidiabetic agents: associations with adherence; treatment satisfaction and health-related quality of life. Diabetes Res Clin Pract. 2010;87(2):204-210. [PubMed: 20036433]
- 111.
- Assistant Secretary of Planning and Evaluation (ASPE). Examination of clinical trial costs and barriers for drug development. Contract No. HHSP23320095634WC. US Department of Health and Human Services. Published July 25, 2014. Accessed January 31, 2017. https://aspe
.hhs.gov /report/examination-clinical-trial-costs-and-barriers-drug-development - 112.
- Bolen S, Wilson L, Vassy J, et al. Comparative Effectiveness and Safety of Oral Diabetes Medications for Adults With Type 2 Diabetes. Comparative Effectiveness Review No. 8. Agency for Healthcare Research and Quality. Published July 2007. Accessed January 31, 2017. http://www
.ncbi.nlm.nih .gov/books/NBK43056/pdf [PubMed: 20704051] - 113.
- Bennett WL, Wilson LM, Bolen S, et al. Oral Diabetes Medications for Adults With Type 2 Diabetes: An Update. Comparative Effectiveness Review No. 27. AHRQ Publication No. 11-EHC038-EF. Agency for Healthcare Research and Quality. Published March 2011. Accessed January 31, 2017. https:
//effectivehealthcare .ahrq.gov/sites /default/files/pdf /type-2-diabetes-medications-update _research.pdf - 114.
- Bennett WL, Balfe LM, Faysal JM. AHRQ’s comparative effectiveness research on oral medications for type 2 diabetes: a summary of the key findings. J Manag Care Pharm. 2012;18(1-a):S3-S20. [PMC free article: PMC10437459] [PubMed: 22313233]
- 115.
- Qaseem A, Barry MJ, Humphrey LL, Forciea MA; Clinical Guidelines Committee of the American College of Physicians. Oral pharmacologic treatment of type 2 diabetes mellitus: a clinical practice guideline update from the American College of Physicians. Ann Intern Med. 2017;166(4):279-290. [PubMed: 28055075]
- 116.
- Sherifali D1, Nerenberg K, Pullenayegum E, Cheng JE, Gerstein HC. The effect of oral antidiabetic agents on A1c levels: a systematic review and meta-analysis. Diabetes Care. 2010;33(8):1859-1864. [PMC free article: PMC2909079] [PubMed: 20484130]
- 117.
- Collins FS, Varmus H. A new initiative on precision medicine. N Engl J Med. 2015;372(9):793-795. [PMC free article: PMC5101938] [PubMed: 25635347]
- 118.
- Collins FS, Varmus H. The 21st Century Cures Act - a view from the NIH. N Engl J Med. 2017;376(2):111-113. [PMC free article: PMC6688288] [PubMed: 27959585]
- 119.
- National Institutes of Health. The All of Us Research Program. Accessed January 31, 2017. https://www
.nih.gov/research-training /allofus-research-program - 120.
- Testa MA, Simonson DC. Health disparities among patients with diabetes can be improved by new approaches. News release. American Diabetes Association; June 12, 2017. Accessed October 15, 2017. https://www
.prnewswire .com/news-releases /health-disparities-among-patients-with-diabetes-can-be-improved-by-new-approaches-and-insights-300472642.html - 121.
- Diabetes Control and Complications Trial Research Group, Nathan DM, Genuth S, et al. The effect of intensive treatment of diabetes on the development and progression of long-term complications in insulin-dependent diabetes mellitus. N Engl J Med. 1993;329(14):977-986. [PubMed: 8366922]
- 122.
- Stratton IM, Adler AI, Neil HA, et al. Association of glycaemia with macrovascular and microvascular complications of type 2 diabetes (UKPDS 35): prospective observational study. BMJ. 2000;321(7258):405-412. [PMC free article: PMC27454] [PubMed: 10938048]
- 123.
- Fleming BB, Greenfield S, Engelgau MM, Pogach LM, Clauser SB, Parrott MA. The Diabetes Quality Improvement Project: moving science into health policy to gain an edge on the diabetes epidemic. Diabetes Care. 2001;24(10):1815-1820. [PubMed: 11574448]
- 124.
- Pogach L, Aron DC. Sudden acceleration of diabetes quality measures. JAMA. 2011;305(7):709-710. [PubMed: 21325188]
- 125.
- Mitri J, Gabbay RA. Measuring the quality of diabetes care. Am J Manag Care. 2016;22(4 Spec No.):SP147-SP148. [PubMed: 29381309]
- 126.
- Wasson JH, Sox HC, Neff RK, Goldman L. Clinical prediction rules. Applications and methodological standards. N Engl J Med. 1985;313(13):793-799. [PubMed: 3897864]
- 127.
- Sentinel. Key database statistics. Sentinel Coordinating Center. Accessed December 1, 2019. https://www
.sentinelinitiative .org/sentinel /data/snapshot-database-statistics - 128.
- PCORnet, the National Patient-Centered Clinical Research Network. Accessed January 31, 2017. http://pcornet
.org/
Related Publications
- •.
- Testa MA, Su M, Simonson DC. Modeling pathways between psychological distress, emergent symptoms and side effects, and endpoint A1c during treatment. Diabetes. 2019;68(Suppl 1):850-P.
- •.
- Testa MA, Turchin A, Su M, Simonson DC. Impact of patient-centered factors on comparative effectiveness of initial therapies in treatment-naïve T2D. Diabetes. 2018;67(Suppl 1):1520-P, A407.
- •.
- Testa MA, Saldivar-Salazar S, Su M, Marc LG, Simonson DC. A social media learning collaborative approach to competency-based training in diabetes. Diabetes. 2017;67(Suppl 1):368-OR, A97.
- •.
- Zhang H, Hosomura N, Shubina M, Simonson DC, Testa MA, Turchin A. Electronic documentation of lifestyle counseling in primary care is associated with lower risk of cardiovascular events in patients with diabetes. Diabetes. 2016;65(Suppl 1):1387-P, A363.
- •.
- Turchin A, Sosina O, Zhang H, et al. Effect of medication reconciliation on emergency department visits and hospitalizations in patients with diabetes. Diabetes. 2016;65(Suppl 1):1216-P, A319.
- •.
- Testa MA, Marc LG, Turchin A, Simonson DC. Impact of subclinical depression and anxiety on intensity and frequency of treatment-related side effects and symptoms. Diabetes. 2016;65(Suppl 1):823-P, A211.
- •.
- Testa MA, Su, M, Turchin A, Simonson DC. Patient-centered outcomes and socio-demographic predictors of diabetes treatment effectiveness. Diabetes. 2016;65(Suppl 1):1235-P, A254.
- •.
- Testa MA, Saldivar-Salazar A, Savoia E, Simonson D. Using social media crowdsourced data for diabetes patient-centered outcomes research (PCOR). Diabetes. 2015;64(Suppl 1):220-OR, A55.
- •.
- Simonson DC, Marc LG, Testa MA. Health education internet use by persons with diabetes in the US. Diabetes. 2015;64(Suppl 1):770-P, A159.
- •.
- Testa MA, Turchin A, Sosina OA, Simonson DC. Benchmarking T2DM treatment effectiveness in clinical practice. Diabetes. 2015;64(Suppl 1):1330-P, A346.
- •.
- Testa MA, Simonson DC. Development of a personalized, patient-centered glycemic control benchmarking tool for T2DM. Diabetes. 2014;62(Suppl 1):A303, 1165-P.
- •.
- Testa MA, Simonson DC. Socioeconomic and demographic predictors of internet and social media use in persons with diabetes. Diabetes. 2013;61(Suppl 1).
- •.
- Zhang H, Goldberg SI, Hosomura N, et al. Lifestyle counseling and long-term clinical outcomes in patients with diabetes. Diabetes Care. 2019;42(9):1833-1836. [PubMed: 31371430]
- •.
- Turchin A, Sosina O, Zhang H, et al. Ambulatory medication reconciliation and frequency of hospitalizations and emergency department visits in patients with diabetes. Diabetes Care. 2018;41(8):1639-1645. [PubMed: 29891639]
- •.
- Simonson DC, Halperin F, Foster K, Vernon A, Goldfine AB. Clinical and patient-centered outcomes in obese patients with type 2 diabetes 3 years after randomization to Roux-en-Y gastric bypass surgery versus intensive lifestyle management: the SLIMM-T2D study. Diabetes Care. 2018;41(4):670-679. [PMC free article: PMC5860843] [PubMed: 29432125]
- •.
- Panosian J, Ding SA, Wewalka M, et al. Physical activity in obese type 2 diabetes after gastric bypass or medical management. Am J Med. 2017;130(1):83-92. [PMC free article: PMC5164867] [PubMed: 27555097]
- •.
- Palermo NE, Modzelewski KL, Farwell AP, et al. Open access to diabetes center from the emergency department reduces hospitalizations in the subsequent year. Endocr Pract. 2016;22(10):1161-1169. [PubMed: 27732094]
- •.
- Ding SA, Simonson DC, Wewalka M, et al. Adjustable gastric band surgery or medical management in patients with type 2 diabetes: a randomized clinical trial. J Clin Endocrinol Metab. 2015;100(7):2546-2556. [PMC free article: PMC4490302] [PubMed: 25909333]
- •.
- DeFronzo RA, Ferrannini E, Groop L, et al. Type 2 diabetes mellitus. Nat Rev Dis Primers. 2015;1:15019. doi: 10.1038/nrdp.2015.19 [PubMed: 27189025] [CrossRef]
Published Abstracts
Published Journal Articles
Acknowledgments
We would like to acknowledge the contributions of all the members of our research team, including Elena Savoia, MD, MPH (co-investigator); Linda G. Marc, ScD, MPH, MS (co-investigator); Olukayode Sosina, BS (research assistant); and Leesa Lin, MS (program manager). We also want to thank members or our advisory committee, including Ronald A. Arky, MD; Margo S. Hudson, MD; Rita McCarthy, ANP, CDE; Robert Sala; Sergio Saldivar-Salazar, MD, ScM; and Jayanti Mukherjee, PhD. In addition, we are thankful to our stakeholder and forum members, as well as the members and leadership of the ADA.
Research reported in this report was funded through a Patient-Centered Outcomes Research Institute® (PCORI®) Award (#CE-1304-6756). Further information available at: https://www.pcori.org/research-results/2013/including-patient-traits-benchmarks-diabetes-caret
Appendices
Appendix 1.
Stakeholder and Patient Social Media Engagement Websites (PDF, 3.5M)
Appendix 2.
Pooled RCT Outcomes Measures (PDF, 98K)
Suggested citation:
Testa MA, Su M, Turchin A, Simonson DC. (2021). Benchmarking the Comparative Effectiveness of Diabetes Treatments Using Patient-Reported Outcomes and Socio-Demographic Factors. Patient-Centered Outcomes Research Institute (PCORI). https://doi.org/10.25302/03.2021.CE.13046756
Disclaimer
The [views, statements, opinions] presented in this report are solely the responsibility of the author(s) and do not necessarily represent the views of the Patient-Centered Outcomes Research Institute® (PCORI®), its Board of Governors or Methodology Committee.
- NLM CatalogRelated NLM Catalog Entries
- PMCPubMed Central citations
- PubMedLinks to PubMed
- Folic acid supplementation and malaria susceptibility and severity among people taking antifolate antimalarial drugs in endemic areas.[Cochrane Database Syst Rev. 2022]Folic acid supplementation and malaria susceptibility and severity among people taking antifolate antimalarial drugs in endemic areas.Crider K, Williams J, Qi YP, Gutman J, Yeung L, Mai C, Finkelstain J, Mehta S, Pons-Duran C, Menéndez C, et al. Cochrane Database Syst Rev. 2022 Feb 1; 2(2022). Epub 2022 Feb 1.
- Review Behavioral and Pharmacotherapy Weight Loss Interventions to Prevent Obesity-Related Morbidity and Mortality in Adults: An Updated Systematic Review for the U.S. Preventive Services Task Force[ 2018]Review Behavioral and Pharmacotherapy Weight Loss Interventions to Prevent Obesity-Related Morbidity and Mortality in Adults: An Updated Systematic Review for the U.S. Preventive Services Task ForceLeBlanc EL, Patnode CD, Webber EM, Redmond N, Rushkin M, O’Connor EA. 2018 Sep
- Behavioural modification interventions for medically unexplained symptoms in primary care: systematic reviews and economic evaluation.[Health Technol Assess. 2020]Behavioural modification interventions for medically unexplained symptoms in primary care: systematic reviews and economic evaluation.Leaviss J, Davis S, Ren S, Hamilton J, Scope A, Booth A, Sutton A, Parry G, Buszewicz M, Moss-Morris R, et al. Health Technol Assess. 2020 Sep; 24(46):1-490.
- Behavioural interventions for type 2 diabetes: an evidence-based analysis.[Ont Health Technol Assess Ser....]Behavioural interventions for type 2 diabetes: an evidence-based analysis.Medical Advisory Secretariat. Ont Health Technol Assess Ser. 2009; 9(21):1-45. Epub 2009 Oct 1.
- Review Measuring the Effects of Social Risks on Patient Health Outcomes – A PCORnet® Study[ 2021]Review Measuring the Effects of Social Risks on Patient Health Outcomes – A PCORnet® StudyCottrell EK, Hendricks M, Todd J, Barker G, Cowburn S, Dambrun K, O'Malley J, Park B, Foley P, Ritchie F, et al. 2021 Aug
- Benchmarking the Comparative Effectiveness of Diabetes Treatments Using Patient-...Benchmarking the Comparative Effectiveness of Diabetes Treatments Using Patient-Reported Outcomes and Socio-Demographic Factors
Your browsing activity is empty.
Activity recording is turned off.
See more...