Diabetes in America is in the public domain of the United States. You may use the work without restriction in the United States.
NCBI Bookshelf. A service of the National Library of Medicine, National Institutes of Health.
Lawrence JM, Casagrande SS, Herman WH, et al., editors. Diabetes in America [Internet]. Bethesda (MD): National Institute of Diabetes and Digestive and Kidney Diseases (NIDDK); 2023-.
Summary
Type 1 diabetes is a complex disease that has both genetic and environmental determinants. Based on twin and family studies from largely European-ancestry populations, the estimated contribution of genetic factors to type 1 diabetes risk is ~50%. Genes and their variants within the human major histocompatibility complex (MHC), the human leukocyte antigen (HLA) loci, including class I (HLA-A, -B, and -C) and class II (HLA-DR, -DQ, and -DP), account for ~50% of the genetic risk of type 1 diabetes. In addition to the MHC region, type 1 diabetes risk loci were initially identified through candidate gene and linkage studies, including variants in or near the INS, CTLA4, IL2RA, and PTPN22 genes. Genome-wide association approaches have revealed additional loci containing common variants with relatively small individual effects on type 1 diabetes risk. International efforts led by the Type 1 Diabetes Genetics Consortium and others have identified more than 90 non-MHC loci and narrowed the likely candidate genes and variants substantially through fine-mapping and functional studies. Ancestry-specific genetic associations of type 1 diabetes have emerged. The majority of non-MHC variants are in noncoding sequences and most likely affect gene regulation rather than directly altering protein structure. In addition to genetic factors, epigenetic changes in the genome are implicated in type 1 diabetes. Research on large cohorts of non-European ancestry, longitudinal follow-up of individuals in the preclinical stages of type 1 diabetes, and identification of the genetic and environmental underpinnings of distinct phenotypes that are emerging within the wide spectrum of type 1 diabetes are needed. Analytic and molecular studies to assess the functional significance of type 1 diabetes susceptibility gene variants would help identify critical biologic pathways that could lead to novel interventions and therapeutics.
Introduction
Type 1 diabetes begins with precipitating events in an individual with genetic susceptibility. Twin and family studies provide evidence for genetic factors contributing to type 1 diabetes risk and estimates of the familial aggregation of the disease based on risk in relatives of an affected individual. In monozygotic twins, who share 100% of their genes, when a member of the pair has type 1 diabetes (proband twin), the risk to the co-twin is approximately 50%, suggesting that both genetic and nongenetic factors contribute to risk (1,2). This concordance for type 1 diabetes increases to 65% by age 60 years and 89% for autoantibody-positive pairs (2). High-risk genotypes of the human leukocyte antigen (HLA) loci (HLA-DR3, -DR4) in the major histocompatibility complex (MHC) (3) and the insulin (INS) gene (4) are found in higher frequency in concordant pairs, suggesting a major impact of genetic factors and heterogeneity in concordance rates. Dizygotic twins and non-twin siblings share only 50% of their genes, and accordingly, their concordance rate for type 1 diabetes is lower—under 8% (5,6). In contrast, the estimated prevalence of type 1 diabetes in the general population of the United States is approximately 4 per 1,000. Highlighting the role of the environment, siblings, who have lesser extent of common exposures than twins, have lower risk of development of islet autoimmunity and clinical type 1 diabetes than dizygotic twins (6).
Type 1 diabetes is most common in populations of European ancestry, but it also occurs in those of African, Hispanic, and Asian ancestry. The incidence and prevalence of type 1 diabetes in youth have been increasing in the United States over the past two decades, particularly among Hispanic and non-Hispanic Black youth (7,8). From 2002–2003 to 2017–2018, the adjusted annual incidence increase (95% confidence interval) of type 1 diabetes among African American, Hispanic American, and Asian American and Pacific Islander U.S. youth age <20 years was 2.93 (1.91–3.96), 4.14 (3.19–5.10), and 4.84 (2.57–7.15), respectively, compared to 0.60 (0.04–1.16) in non-Hispanic White youth. Despite extensive epidemiologic data on the incidence and prevalence of type 1 diabetes in various ethnic groups, there is a critical absence of data on risk to siblings in diverse populations, preventing estimation of the genetic impact on type 1 diabetes risk in non-White populations. Nearly one-half of type 1 diabetes cases in non-Hispanic White populations are diagnosed after age 20 years, and the incidence of type 1 diabetes seems to steadily increase throughout the adult years (9,10). However, the absence of information on the risk to siblings of individuals with adult-onset type 1 diabetes prevents estimation of the impact of genetic factors on risk of type 1 diabetes in adults of any population ancestry.
The genetic risk ratio in siblings (lambda-s), which is defined as the ratio of the risk in siblings of individuals with the disease to the population prevalence, is ~16 in type 1 diabetes of European ancestry (11). Yet, this figure provides no insight into either the number of genes contributing to type 1 diabetes risk or the sizes of their effects. About 50% of the risk of type 1 diabetes can be attributed to genetic (familial) factors; however, the contribution of specific genes and their variants to the overall genetic risk is variable. For example, in families with two children with type 1 diabetes, the expectation under the hypothesis that a variant in a gene has no effect on type 1 diabetes risk is that 25% of sibling pairs would share both copies of the variant (same genotype), 50% would share one copy of the variant in the genotype, and 25% would share no copies of the variant (completely different genotypes). In fact, the risk to siblings who share two HLA haplotypes is 55%, much greater than 25%, indicating that a significant proportion of the total genetic risk can be attributed to the HLA region (11).
The recognition that approximately half of risk for type 1 diabetes is genetic and half of that genetic risk is due to factors in the MHC provided the stimulus for two subsequent research paths. The first was to delineate the specific genes and mechanisms in the MHC (the HLA genes and other genes and variants) that account for the majority of type 1 diabetes genetic risk. The second was to identify the non-MHC genes that account for the remaining genetic risk for type 1 diabetes. Two primary study designs were used for these efforts: family-based (typically, families with two parents and two type 1 diabetes-affected children, i.e., affected sib pair [ASP] families) and case-control (a series of type 1 diabetes cases and a series of unaffected controls). The family-based designs were used for linkage analyses (i.e., to look for evidence of increased sharing of alleles or chromosomal segments in the ASP) or for association in the presence of linkage analyses (i.e., to evaluate whether the frequency of transmission of an allele from heterozygous parents to their affected child deviates from the expected 50% rate when there is no linkage). As collection of large numbers of ASP families (for robust statistical power) was difficult, much of the genetic evaluation of genes contributing to type 1 diabetes employed a case-control design.
Genome-wide association studies (GWAS) of cases of type 1 diabetes and controls with very large sample sizes, on the order of thousands, allowed the identification of multiple additional single nucleotide polymorphisms (SNPs) that are associated with type 1 diabetes, although each of them confers small risk (i.e., low odds ratio [OR]). Replication of findings to confirm the associations was followed by fine-mapping and functional studies to identify the causal genes and pathophysiological mechanisms that underlie the associations. Since the initial studies included participants mostly from European ancestry, there is great interest in cohorts from other ancestries to advance our understanding of type 1 diabetes in diverse populations.
Genetics improves the ability to predict type 1 diabetes in individuals at risk (12,13,14,15,16), is shedding light on the heterogeneity of the disease (17,18), and may provide evidence of underlying pathophysiological mechanisms (19) that could be targeted for prevention and treatment. The following sections expand on the evolution of genetics technology and findings related to the genetic basis of type 1 diabetes, including progress made since publication of Diabetes in America, 3rd edition (20).
Major Histocompatibility Complex and Type 1 Diabetes Risk
Between the 1970s and 2000, research efforts focused on the localization and identification of genes that contribute to the occurrence of type 1 diabetes, as well as other autoimmune diseases, particularly genes involved in the immune response. Clear candidates in this regard were genes encoding the HLA molecules, which are highly polymorphic and play critical roles in the immunologic distinction between self and non-self, as well as in the presentation of peptide antigens to the T lymphocytes. In humans, the MHC is a gene-rich region on chromosome 6p21.3 that includes genes encoding the HLA class I (HLA-A, -B, and -C) and class II (HLA-DR, -DQ, and -DP) molecules (Figure 1) (21). The importance of HLA in type 1 diabetes risk is likely through its role in antigen presentation; genetic variation in this system could interfere with central tolerance during T cell development in the thymus or with peripheral tolerance by altering the repertoire of antigens presented (Figure 2) (22). The cellular immune response is centered on antigen presentation, in which foreign antigens are recognized by antigen-presenting cells (APCs), processed into peptides, complexed to HLA class I or II (depending on the source of those antigens), and presented on the cell surface, where they can be recognized by T cells. Since the T cell does not recognize unbound foreign peptides, antigen presentation requires antigen processing, binding to the HLA molecule, and display of the HLA-bound peptide on the surface of the APC. Thus, a critical aspect of immunological protection is the ability of the HLA system to provide a broad array of molecules to bind and present the foreign peptide, which is determined by genetic variation.
The HLA genes contain many alternative forms (i.e., many alleles) and thereby provide extensive variation in humans, resulting in the most variable region of the human genome. This extraordinary variability is critical to the breadth of immune responses. The high allelic polymorphism of HLA class I and II genes makes it extremely unlikely that two unrelated individuals should share the same combination of HLA alleles. All normal cells in the body, except for erythrocytes, express HLA class I and constantly present self peptides within those HLA class I molecules to CD8 T cells. In addition, professional APCs, such as dendritic cells or macrophages, express HLA class II and present foreign peptides within either their HLA class I or class II molecules to CD8 or CD4 T cells, respectively. Recognition of the HLA-peptide by the T cell receptor (TCR) initiates an intracellular signal cascade that leads to T cell activation.
The initial report of a strong association of HLA with type 1 diabetes occurred in 1973 with alleles of the class I HLA-A (or then, HL-A) locus (23). Many reports confirmed and extended the association of type 1 diabetes with antigens/alleles of the class I (HLA-A and -B) and class II (HLA-DR, -DQ, and -DP) loci. Studies on the effect of HLA-A, -B, and -DR were conducted in small samples of cases and controls, which were robust due to the large effect of the HLA alleles on risk/protection. This approach had two consequences—a focus on the immune system in a number of candidate gene studies and the simultaneous publication of underpowered candidate gene studies (as the expected effect sizes were far too large, as evidenced by the Wellcome Trust Case-Control Consortium [WTCCC], powered to detect OR ~1.5 with SNPs at MAF [minor allele frequency] >0.05). Of course, a limitation of early studies was the absence of cost-effective, genome-wide coverage of reliable markers, with “candidate gene” evaluations primarily of a few coding, sometimes promoter, variants.
The MHC region on chromosome 6p21.3 spans ~4 Mb, is gene rich (Figure 1) (21), and lacks informative recombination events, resulting in strong linkage disequilibrium (LD). LD is the occurrence of combinations of alleles at adjacent loci more often than expected from the frequencies of the individual alleles; groups of alleles on a chromosomal segment can be inherited as a unit or haplotype. The human MHC displays strong LD, and specific HLA haplotypes are associated with type 1 diabetes risk, providing a “fingerprint” of the transmission of disease-associated variation across populations.
Statistical methods can help to construct HLA haplotypes from unrelated individuals; however, the most precise method for observation and estimation of haplotypic association comes from family studies. As noted, multiple family studies have been conducted in non-Hispanic White populations, typically restricted to probands diagnosed with type 1 diabetes before age 16 years but up to age 35 years in siblings. A common measure of association is the odds ratio, which measures the relationship between an exposure (i.e., genotype or haplotype) and an outcome (i.e., type 1 diabetes). As the odds ratio is used to compare the relative odds of the outcome given the exposure, it can be interpreted as “risk” (OR >1), “protection” (OR <1), or neutral (OR 1). A family study of type 1 diabetes (24) demonstrated that the strongest class I associations with type 1 diabetes occurred with the HLA-B8 (OR 3.20) and HLA-B15 (OR 3.69) alleles and the class II associations with the HLA-DR3 (OR 3.54) and HLA-DR4 (OR 6.81) alleles (risk), as well as the HLA-DR2 (OR 0.21), HLA-DR5 (OR 0.30), and HLA-DR7 (OR 0.24) alleles (protective). Presence of the HLA-B8, -B15, -DR3, and -DR4 alleles increased type 1 diabetes risk, while the presence of HLA-DR2, -DR5, and -DR7 decreased type 1 diabetes risk. However, the haplotypes formed by HLA-B7-DR2 (OR 0.10) and HLA-B15-DR4 (OR 7.55) exhibited stronger association than the individual alleles, highlighting the genetic complexity within the MHC association with type 1 diabetes.
Of note, type 1 diabetes-associated alleles and haplotypes were obtained from studies that primarily recruited individuals of European ancestry. The association of HLA alleles and haplotypes with type 1 diabetes remains strong across populations of non-European ancestry, but the specific alleles and their strength vary. Studies of the HLA region have identified ancestry-specific HLA alleles that harbor risk for type 1 diabetes and demonstrate the necessity of studying diverse populations (25,26) (reviewed in Redondo et al. (27)). Figure 3 shows the distribution of five HLA-DR-DQ haplotypes and their effect on risk or protection in different world regions (27). For example, in Asians, a high risk is seen for DRB1*09:01, an allele found at low frequency in Europeans and, therefore, not associated with type 1 diabetes risk in Europeans. There are also ethnic differences in HLA allele frequencies that differ from European populations. For instance, African-specific HLA-DR3 and HLA-DR7 haplotypes have opposite effects on type 1 diabetes susceptibility than their European counterparts. Assembling large cohorts of non-European type 1 diabetes cases or families is difficult, given differences in disease risk among races/ethnicities. The study of type 1 diabetes in non-European populations, from both epidemiologic and genetic perspectives, is an important area of research.
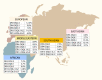
FIGURE 3.
Regional Frequencies of Five HLA-DR-DQ Haplotypes. This map depicts the prevalence of five well-known HLA-DR-DQ haplotype groups that confer susceptibility or resistance to type 1 diabetes in five different world regions.
Subsequent studies have investigated the association of class I and class II alleles with type 1 diabetes, adjusting for LD within the MHC in numerous populations. The most comprehensive evaluation of these associations occurred at the HLA and Immunogenetics Workshop (28). The class I alleles most significantly associated with type 1 diabetes are HLA-B*57:01 (protective) and HLA-B*39:06 (susceptible). The HLA-B*39:06 allele increases the risk of HLA-DR4 susceptibility haplotypes in Scandinavian populations, as well as other risk haplotypes (DR3, DR1, and DR8) in larger cohorts. Other significantly associated class I alleles include: HLA-A*24:02, HLA-A*02:01, HLA-B*18:01, and HLA-C*05:01 (susceptibility); and HLA-A*11:01, HLA-A*32:01, HLA-A*66:01, HLA-B*07:02, HLA-B*44;03, HLA-B*35:02, HLA-C*16:01, and HLA-C*04:01 (protective) (28,29).
HLA allele and haplotype frequencies differ among populations, perhaps contributing to the differences in type 1 diabetes prevalence across geographic areas. There is a North-South gradient in type 1 diabetes prevalence, with Scandinavians having the highest type 1 diabetes risk. An exception to this rule is Sardinia, an island in the Mediterranean Sea, in the South of Europe. The high prevalence of type 1 diabetes in Sardinia has been attributed to the founder effect that established particularly high frequencies of the type 1 diabetes-susceptible HLA-DR3 and HLA-DR4 alleles and haplotypes and low frequency of the type 1 diabetes-protective HLA-DR2 allele and haplotype (30). In addition, most of the Sardinian HLA-DR2 haplotypes carry a DQB1*05:02 allele that is not protective (31).
The influence of the MHC region on type 1 diabetes risk is highly complex. Variation in the genes encoding the HLA-DR and HLA-DQ molecules is associated with risk of type 1 diabetes with odds ratios in excess of 10 for susceptible genotypes (e.g., DR3/DR4 or DRB1*03:01-DQB1*02:01/DRB1*04-DQB1*03:02) or under 0.1 for protective genotypes (e.g., DR2/DRX, where “X” is neither DR3 nor DR4 [or DR2]; the dominantly protective haplotype is DRB1*15:01-DQB1*06:02). The class II molecules encoded by these genes combine to form heterodimeric (αβ) protein receptors that are expressed on the surface of APCs. HLA-DQ is determined by a polymorphic α-chain (encoded by the HLA-DQA1 locus) and a polymorphic β-chain (encoded by the HLA-DQB1 locus). On the other hand, HLA-DR is determined by a monomorphic α-chain (encoded by the HLA-DRA locus) paired with a highly polymorphic β-chain. Most DR haplotypes in the population contain two loci: DRB1 and a second DRB locus (either DRB3, DRB4, or DRB5). A few DR haplotypes (e.g., DRB1*01, DRB1*08, and DRB1*10) do not have a second DRB locus. A heterozygous individual could encode up to four different DRB molecules (32). Thus, type 1 diabetes risk is determined by specific genotype combinations. The genotype HLA-DRB1*03-DQB1*02/HLA-DRB1*04-DQB1*03:02 confers the highest risk and has the highest frequency in type 1 diabetes cases with youngest onset.
The allelic variations in the critical HLA class II genes (HLA-DRB1, HLA-DQA1, and HLA-DQB1) are thought to alter specific amino acid residues that affect binding of peptides leading to initiation of the autoimmune disease process. Independent amino acid positions and large-scale genetic studies allowed examination of the contribution of HLA class II alleles and their interactions in type 1 diabetes (33). A large series of European cases, controls, and families allowed the study of more than 7,000 SNPs and amino acid residues at 399 positions for eight HLA genes. As expected, the single most associated risk variant was HLA-DQB1*03:02 that encodes an alanine at HLA-DQB1 position 57, while the aspartic acid at this position is most protective (Figure 4) (33). The second independent association is at HLA-DRB1 position 13, where a histidine residue (tagging HLA-DR4) confers risk (arginine is protective), as does a serine residue that tags HLA-DR3 (tyrosine is protective). The HLA-DRB1 residue at position 71 is a third independent association, with lysine conferring strong risk (tagging HLA-DR3 and HLA-DR4), while an alanine residue at this site is protective.
The HLA-DQB1 position 57, HLA-DRB1 position 13, and HLA-DRB1 position 71 are each located in the peptide-binding groove of the HLA molecule (Figure 5) (33). HLA-DRB1 positions 13 and 71 line the P4 pocket of HLA-DR. The HLA-DQB1 position 57 alone accounts for ~15% of the total risk of type 1 diabetes, and HLA-DRB1 position 13 and HLA-DRB1 position 71 account for an additional 12% of risk (33). Therefore, together, these three amino acid positions capture ~27% of type 1 diabetes risk or ~80% of the MHC-associated risk. The total type 1 diabetes risk explained by independent (additive) effects in the classical HLA genes is ~34% (Figure 6) (33). Structurally, the known association of HLA-DQB1 position 57 would alter the HLA-DQ P9 pocket; however, the associations with the HLA-DRB1 position 13 and HLA-DRB1 position 71 sites would alter the HLA-DR P4 pocket. This structural site may be crucial in binding specific autoantigens that are associated with type 1 diabetes risk.
Although the influence of HLA class II genes on type 1 diabetes risk remains unquestioned, there is significant residual evidence of association with type 1 diabetes in the MHC region after controlling for the effects of HLA class II loci (33). Analysis of HLA haplotypes indicates that nonadditive effects are common within the MHC. HLA-DQB1 position 57 and HLA-DRB1 position 13 are the strongest factors in both additive and interactive effects on type 1 diabetes risk in the MHC (34). These two strongest interacting positions are in separate HLA molecules (HLA-DQ for position 57 and HLA-DR for position 13). In a detailed interaction analysis of the MHC, the effect of interaction within a site (dominance) was shown to have a small (~1%), but significant, impact on the contribution to risk of type 1 diabetes (34). Including interactions at the individual haplotypes, rather than alleles at a given site, resulted in an additional 3% of risk of type 1 diabetes. Jointly, the additive and interaction effects of the classical HLA sites and amino acid residues account for more than 90% of the MHC-type 1 diabetes association.
Non-Major Histocompatibility Complex Risk Loci
The two common methods by which genetic variation in association with type 1 diabetes risk is evaluated rely on assessing DNA sequence variation and studying either co-segregation with type 1 diabetes in families or differences between type 1 diabetes cases and controls. One such DNA variant occurs when a single nucleotide in the genome differs in a population with greater than 5% frequency; this variant is then termed a SNP. Millions of SNPs exist throughout the human genome—roughly one SNP every 2,000 bases.
Linkage Mapping
The major goal of genome-wide linkage scans in type 1 diabetes was to identify regions of the genome that exhibited a significant deviation of “sharing” (identity-by-descent, IBD) by sibling pairs from that expected for Mendelian segregation of chromosomes (e.g., 25% sharing two alleles IBD, 50% sharing one allele IBD, 25% sharing no alleles IBD). In type 1 diabetes, this process was typically performed on families with multiple affected siblings, with a large number of families required to achieve strong statistical power given the small expected size of risk associated to non-MHC genes (35,36). This strategy effectively detected genes with variant alleles that have a large effect on risk of type 1 diabetes.
Unlike the MHC, for which a sample of 100 ASP families could detect significant evidence of linkage, the lower risk anticipated for other genes suggested a sample size of as many as 4,000 ASP families. The Type 1 Diabetes Genetics Consortium (T1DGC) was created to assemble a collection of ASP families to conduct genome-wide linkage scans for type 1 diabetes (37). Although the resolution of the linkage approach was limited to ~1 Mb regions, the first major analysis of 2,658 ASP families confirmed the contribution of the HLA region, INS, and CTLA4 (35). With additional collection of ASP families, analysis of 4,422 ASP families continued to identify the contribution of the MHC and the INS locus, but no compelling evidence for other susceptibility genes with similar large effects was found (36). These results suggested that additional loci contributing as much as 50% of the genetic risk have smaller effects and may be common, with allele frequency greater than 5% in the population, or private (unique) to individual families, and thus, they require a different experimental approach for detection.
Genome-Wide Association Studies
With the improved genomic coverage through SNP discovery from the International HapMap Project (38) and decreasing costs of genotyping, the use of GWAS with robust statistical power became feasible. The primary source of participants for GWAS is unrelated type 1 diabetes cases and controls, as the statistical method is association and not linkage. Association studies take advantage of LD. Generally, the lead variant identified by GWAS is most likely not the “true” type 1 diabetes causal variant but in high LD with it. However, family-based association analyses provide important complementary results because of their resistance to stratification bias, which occurs, for example, when genetic differences in cases and controls occur due to sampling from populations of different ancestry and are unrelated to disease.
The first GWAS publications in type 1 diabetes focused on nonsynonymous SNPs (i.e., those that modify the amino acid sequence of protein), with the assumption that variants resulting in an amino acid variation would increase the likelihood of detecting a functional change associated with type 1 diabetes risk (39). This design led to the discovery of a novel gene, IFIH1, as having multiple, rare, protein-coding variants associated with type 1 diabetes. The function of IFIH1 was not in the obvious immune pathway of cytokines or beta cell proteins—IFIH1 encodes a cytoplasmic sensor of viral RNA that is part of the innate immune system and the response to viral infection. Notably, IFIH1 is involved in the response to picornaviruses, a family that includes viruses previously implicated in type 1 diabetes risk (40). Rare, inactivating alleles of IFIH1 are underrepresented among those with type 1 diabetes, suggesting that loss-of-function is protective from type 1 diabetes. In this manner, GWAS results can lead to discovery of genes that identify novel biologic pathways and avenues to novel drug targets to prevent or reduce the burden of type 1 diabetes.
The WTCCC conducted the first extensive GWAS using the Affymetrix GeneChip 500K Mapping Array Set in participants from the United Kingdom (41). Approximately 2,000 subjects for each of seven diseases and a shared set of approximately 3,000 controls were genotyped. Case-control analyses identified seven significant, independent associations with type 1 diabetes. Six genes/regions had strong preexisting statistical support for a role in type 1 diabetes susceptibility: namely, MHC, INS, CTLA4, PTPN22, IL2RA/CD25, and IFIH1. The WTCCC study demonstrated the power of the GWAS approach to identify novel loci associated with type 1 diabetes in an unbiased manner and suggested that larger samples with greater density of SNP genotyping could uncover additional novel genes and pathways related to type 1 diabetes risk. Following the WTCCC study, the 16p13 region containing DEXI and KIAA0350 was identified in a second GWAS (42) and as a novel locus of susceptibility for other autoimmune diseases, consistent with pervasive sharing of genetic risk across immune-mediated disorders (43).
The T1DGC expanded the results from the WTCCC by starting a new GWAS of 3,983 type 1 diabetes cases and 3,999 controls, followed by a combined meta-analysis with previously published studies that added 3,715 additional cases and 5,069 controls with information from more than 841,622 SNPs. From the meta-analysis of 7,514 cases and 9,045 controls with confirmation in 4,267 cases, 4,670 controls, and 2,319 ASP families, 28 novel regions were shown to be significantly associated with type 1 diabetes risk (44). Eighteen novel regions were strongly replicated from the GWAS meta-analysis.
In total, the number of loci contributing significantly to type 1 diabetes risk reached 42 in 2009, although each locus varied in the size of the interval, number of candidate genes in the locus, size of the effect on type 1 diabetes risk for the most associated SNP, and strength of the evidence supporting the most likely candidate. The genes (loci) of largest impact on type 1 diabetes risk were identified in the 30-year period from the early 1970s (HLA) to 2000 (INS) as the result of small case-control and family studies, reflecting the fact that variants with larger effects can be identified with smaller sample sizes. In 2001–2006, with the advent of large-scale genotyping arrays and assembly of larger case-control series, investigators identified several additional loci (including PTPN22, CTLA4, and IFIH1) that slightly increased the amount of genetic risk identified. In 2007–2008, the first well-powered GWAS by the WTCCC greatly increased the number of loci of modest to small contribution to risk (e.g., IL2RA). In 2009, the T1DGC GWAS meta-analysis (44) with almost 8,000 cases and 9,000 controls plus replication, nearly doubled the number of loci, with each having small effect. Of interest, this meta-analysis in the T1DGC ASP families confirmed the directions of the risk allele effect. However, the size of the effect was lower in the families than in the case-control study, and statistical evidence for replication in families was not reached for 11 of the 18 loci. This finding may indicate the greater role of the MHC in type 1 diabetes risk when multiple family members express disease.
The T1DGC further examined the 18 novel loci (45) by expanding the analyses in 2,322 additional families, which combined with the original 2,319 families, provided improved protection from population stratification bias and increased power for further replication support. With this larger sample of families, only one (C14orf181/14q24.1) of the 18 novel loci did not attain statistical significance. Furthermore, all of the novel type 1 diabetes risk loci had consistent direction of effects (e.g., risk or protective for type 1 diabetes risk) with the original GWAS meta-analysis (44,45) without evidence of variability in the disease associations across family collections, despite significant SNP genotype frequency differences. After clear replication of type 1 diabetes risk loci, the next steps involved dense SNP mapping to better describe causal genes and variants. Identification of genes with both positional and functional evidence for a role in type 1 diabetes pathogenesis could shed light on the pathways underlying type 1 diabetes.
In 2021, Chiou et al. (46) increased the power of type 1 diabetes GWAS by combining datasets to achieve a larger sample size. The compiled dataset included 18,942 individuals with type 1 diabetes and 501,638 control individuals (total of 520,580 European ancestry samples). Through meta-analysis, Chiou et al. identified 81 loci that reached genome-wide significance (P<5x10-8), including 33 loci that were previously unreported (Table 1).

TABLE 1.
Type 1 Diabetes-Associated Genomic Regions From Fine-Mapping Efforts
Fine-Mapping Within Type 1 Diabetes Susceptibility Loci
Most common variants identified through a GWAS are located between genes (intergenic), in regions that contain regulatory DNA, or if located in a gene, in a region that is not protein coding (intronic). Relatively few robustly type 1 diabetes-associated variants with likely deleterious effects have been located in coding regions (exons). Thus, the variants that are associated with type 1 diabetes from GWAS typically do not have obvious functional consequences. This result may suggest that many of the disease-associated variants affect the regulation of gene expression. However, initial GWAS results do not allow exclusion of the possibility that these variants are associated with one or more causative variants within a coding region. The information provided by GWAS on a likely causal gene is limited because of the sparse coverage of a given region of the genome even with high-density SNP genotyping arrays. Thus, prior to detailed examination of genes and regulation, dense SNP mapping of a type 1 diabetes-associated locus is required to firmly establish the most likely associated SNPs and causal genes.
For example, the T1DGC GWAS meta-analysis (44) identified more than 40 loci that are significantly associated with type 1 diabetes risk. However, each type 1 diabetes locus contained an average of seven genes, with a range of 0 to 27 (44,47). Some regions harbored potential candidate genes, for example, involved in the immune response, but in other cases, only a single gene without obvious functional relevance to type 1 diabetes or autoimmunity was found. Indeed, for a few regions, noncoding genes were present. The novel loci identified in the GWAS meta-analysis typically had modest or small effects on type 1 diabetes risk, with C10orf159 (RNLS, renalase) having the largest effect (OR ~1.3) and others with smaller effect sizes, consistent with the dependence of their detection on large sample sizes. A large number of previously detected loci had small effects, including those with strong or confirmed candidate causal genes (e.g., CTLA4, IFIH1).
In most GWAS regions, the locus associated with disease is defined by few (perhaps 100) SNPs. The size of the average type 1 diabetes susceptibility locus from the T1DGC meta-analysis (44) was defined by an ad hoc algorithm based on the most associated SNP at the center with boundaries for that region defined by sites of high recombination. Since there are many candidate genes in each type 1 diabetes region, functional studies are needed to define a role for a particular gene and/or allelic variant in type 1 diabetes pathogenesis. Such studies require significant time and resources, and unless at least the disease-relevant gene within a region is known, those efforts could well target the incorrect gene or variant. To address this concern and refine GWAS regions of susceptibility for type 1 diabetes, dense genotyping (fine-mapping) was required that could comprehensively evaluate the influence of thousands of SNPs in a region.
A GWAS seeks to capture genomic architecture with high information content using a standard set of SNPs. In contrast, fine-mapping requires saturation of specific regions of the genome, with the predetermined boundaries of each region defining the set of SNPs to be genotyped. The ImmunoChip Consortium was established with the purpose of meeting the needs of type 1 diabetes and other autoimmune diseases with genome-wide significant regions to be interrogated. The Consortium consisted of investigators who provided data (including unpublished) to design a genotyping array (the ImmunoChip) for fine-mapping autoimmune disease susceptibility genes. The ImmunoChip is a custom Illumina Infinium high-density genotyping array comprising 196,524 SNPs over 186 regions of the human genome.
For type 1 diabetes, the initial analyses of ImmunoChip data focused on European ancestry subjects and included 6,670 type 1 diabetes cases, 9,416 controls, 2,601 T1DGC ASP families, and 69 T1DGC trio (affected child and both parents) families (48). A large proportion of these samples (98% of cases, 76% of controls, and 57% of family samples) were part of the previous GWAS meta-analysis that initially identified many of the type 1 diabetes genetic regions (44). Fifty-five non-MHC loci were evaluated, including loci that were significantly associated with another autoimmune disease but only nominally with type 1 diabetes. Of the non-MHC type 1 diabetes-associated regions, 36 were significantly associated with type 1 diabetes risk from the ImmunoChip data (including 33 of 45 that had been reported by the T1DGC or others previously). In addition, three loci were nominally associated with type 1 diabetes that were also robustly associated with other autoimmune diseases (49) and were, therefore, included as new type 1 diabetes loci: 1q32.1, also associated with ulcerative colitis, Crohn’s disease, and celiac disease; 4q32.3, also implicated in celiac disease; and 5p13.2, also associated with multiple sclerosis (50,51,52,53,54,55).
While the majority of the large-scale GWAS in type 1 diabetes focused on European ancestry populations, the second phase of T1DGC ImmunoChip fine-mapping analyses focused on ancestrally diverse type 1 diabetes cases, controls, and affected families. Robertson et al. (56) assigned each study subject (n=61,427) to one of five ancestry groups by utilizing principal-component analysis: European (n=47,319), Finnish (n=6,991), East Asian (n=588), African admixed (n=4,290), and other admixed (n=2,239). To improve discovery and fine-mapping resolution, genotypes within ImmunoChip regions were imputed using the Trans-Omics for Precision Medicine (TOPMed) multiancestry reference panel (57). A meta-analysis of the case-control and family results identified 78 chromosome regions associated with type 1 diabetes (P<5×10−8), including 36 new regions (Table 1). Fine-mapping efforts both by Robertson et al. (56) and Chiou et al. (46) revealed that more than one-third of the type 1 diabetes risk loci contain more than one independent association. For example, both studies identified three independent association signals in the PTPN2 locus. This observation suggests that much work remains to be accomplished to identify the causal genes, variants within the genes that modify risk, and functional impact of those variants.
Type 1 Diabetes Risk Loci in Diverse Populations
Until the late 2010s, studies of the genetics of type 1 diabetes focused largely on European populations where the ascertainment of large numbers of individual cases or multiple-case families is easier. The lack of knowledge on the genetic architecture of type 1 diabetes and other common diseases in non-European ancestry populations is widely recognized as a major problem in the era where we aim to utilize genetics to apply precision medicine with the ultimate goal of improving health outcomes in all individuals (58,59). The Major Histocompatibility Complex and Type 1 Diabetes Risk section covers information on ancestry-specific HLA alleles that confer risk for type 1 diabetes. As of 2023, the number of studies with sufficient sample size to discover genetic risk variants outside the HLA region in populations of non-European ancestry is limited.
As yet, the largest GWAS focused on type 1 diabetes in individuals of African ancestry included 1,021 participants with type 1 diabetes and 2,928 control individuals (60). Association studies in the African-ancestry cohort provided concordant findings for some type 1 diabetes loci identified in European-ancestry populations, i.e., 2q33.2 (CTLA4), 6p32 (MHC), 6q22.32 (CENPW), 11p15.5 (INS), 12q13.2 (IKZF4, RPS26, and ERBB3), and 17q12 (IKZF3, ORMDL3, and GSDMB). In addition, it revealed alternative risk alleles at 10q23.31 (RNLS).
In East Asian populations, two GWAS have been conducted. The first study was carried out in 2,596 type 1 diabetes cases and 5,082 control participants with Chinese Han ancestry (61). Zhu et al. identified four loci with genome-wide significance (P<5×10−8), including two known type 1 diabetes risk regions—6p32 (MHC) and 12q13.2 (IKZF4, RPS26, and ERBB3)—and two novel regions—one in 6q22.2 (BTN3A1) and one in 10p14 (GATA3). The 10p14 region harbors type 1 diabetes risk variants also found in European-ancestry individuals (46). A second study analyzed 638 type 1 diabetes cases from Biobank Japan but, likely due to the small sample size, only identified type 1 diabetes risk variants in 6p32 (MHC) (62).
Data on the genetic architecture of type 1 diabetes risk in populations from other regions of the world, such as Ethiopia (63), the Philippines (64), and Indonesia (65), or in the United States, Hispanic individuals (66) and American Indian, Alaska Native, Native Hawaiian, or Pacific Islander populations, are still limited.
From Variant to Function
At a small number of non-MHC loci, a fine-mapped risk variant alters the protein coding sequence of a gene. Genes with protein-altering type 1 diabetes risk variants include PTPN22 (R620W, rs2476601), TYK2 (P1104A, rs34536443; I684S, rs12720356), IFIH1 (I923V, rs35667974; N160D, rs74162074; A946T, rs1990760), SH2B3 (W262R, rs3184504), C1QTNF6 (G21V, rs229527), SIRPG (V263A, rs6043409), CTSH, AIRE (R471C, rs74203920), and PRF1 (A91V, rs35947132). Most protein-altering variants affecting type 1 diabetes are missense and, thus, change an amino acid. An exception is FUT2, where the fine-mapped type 1 diabetes-associated variant W143X (rs601338) introduces an early stop codon, leading to a truncated, nonfunctional protein. Protein-altering risk variants encourage functional studies of the allelic forms of specific proteins to determine whether they impact cellular or organismal phenotypes that could plausibly contribute to type 1 diabetes pathogenesis. In addition, variants can be evaluated for association to other traits and endophenotypes related to type 1 diabetes, such as in phenome-wide association studies (PheWAS) (67), which can also provide insight into variant function.
Functional studies of genes with protein-altering risk variants, including of different protein-coding alleles, support a prominent role for T cells in type 1 diabetes pathophysiology, as well as APCs, beta cells, thymus, and other cell types that modulate immune responses. Variants affecting adaptive immune cell function include PTPN22 R620W, which impacts TCR signaling and T cell activation (68,69,70,71,72), as well as B cell signaling (73,74,75); TYK2 P1104A and I684S, which regulate cellular responses to cytokine signaling (76,77,78,79,80,81,82); PRF1 A91V (83,84); IFIH1 I923V and A946T (85,86); and SIRPG V263A (87,88). Protein-altering variants in other genes instead impact innate immune cell function, including SH2B3 W262R (89) and C1QTNF6 G21V (90,91). Protein-coding variants that affect the beta cell include IFIH1 (92), which modulates intracellular responses to viral RNA, as well as CTSH (93) and TYK2 (76). Finally, protein-altering variants at AIRE R471C and FUT2 W143X implicate such processes as thymic selection and response to bacterial or viral infection, respectively (94,95,96,97).
For most type 1 diabetes-associated loci identified and fine-mapped in GWAS studies, risk variants map to noncoding sequences and likely affect gene regulation (48). Annotating noncoding variants requires detailed maps of cis-regulatory elements in the human genome. High-throughput epigenomic assays that measure hallmarks of gene regulatory activity, such as accessible chromatin, histone modifications, transcription factor binding, and DNA methylation, have been extensively applied to human cell lines, tissues, and primary cells as part of such projects as ENCODE (98,99) and the NIH Epigenome Roadmap (100). These projects have defined tissue and cell-type maps of regulatory elements, including regions of enhancer and promoter activity (98,99,100,101). Technological developments have further enabled profiling the epigenome of single cells, where regulatory elements can be defined in each specific cell type within a heterogeneous tissue (102).
As shown by the T1DGC ImmunoChip study (48) and others (46,56,103), type 1 diabetes-associated variants preferentially map in enhancer regions active in CD4 and CD8 T cells, as well as other immune cell types, such as B cells and macrophages. While type 1 diabetes-associated variants are not localized in pancreatic beta cell enhancers in the basal state, they preferentially map in the subset of beta cell enhancers activated upon proinflammatory cytokine exposure (104,105). Type 1 diabetes-associated variants also map in enhancers specifically active in other cell types, such as acinar and ductal cells of the exocrine pancreas (46).
Risk variants overlapping regulatory elements in type 1 diabetes-relevant cells may not necessarily be functional, and therefore, mapping variant effects on regulatory activity is needed to distinguish functional from benign variants. Approaches to derive risk variant effects on regulatory activity in T cells and other relevant cell types include chromatin accessibility or histone quantitative trait locus (caQTL or hQTL) mapping (106,107,108,109,110,111), allelic imbalance (AI) mapping (112), massively parallel gene reporter assays (MPRAs) (113,114), and machine learning predictions (115,116,117). For example, caQTL mapping in CD4 T cells identified type 1 diabetes-associated variants at multiple loci affecting T cell chromatin (56,118), and an hQTL mapping study determined that type 1 diabetes-associated variants affected enhancer-related histone marks in T cells more often than in other tested cell types (108).
Type 1 diabetes-associated variants that affect enhancer activity can regulate the expression of one or more genes, including over large distances (119,120). Techniques to link enhancers to the genes they affect include 3D physical interactions with Hi-C (a genomic method that captures chromatin conformation) and its derivations (121), co-activity (118,122), the activity-by-contact (ABC) method (123,124), and CRISPR deletion or interference (CRISPRi) (125,126). Enhancer interactions with gene promoters mapped using promoter capture Hi-C (pcHi-C) in 17 primary immune cell types (127) demonstrated that type 1 diabetes-associated variants interacted with genes involved in TCR signaling and other immune signaling pathways in CD4 and CD8 T cells. Another approach for determining genes affected by noncoding variants is QTL mapping of gene expression levels (eQTL) (128). Variants at multiple type 1 diabetes loci are eQTLs in immune cell types, such as monocytes and T cells (108,129,130).
Type 1 diabetes risk loci that affect T cell regulatory activity include IL2RA (131,132,133,134), CTLA4 (135,136,137,138,139), BACH2 (56,108,114,127,140,141), and UBASH3A (142,143). For example, at BACH2, type 1 diabetes-associated variants affect CD4 T cell chromatin accessibility, transcription factor binding, and BACH2 expression, and reduced BACH2 activity affects T cell activation and differentiation (114,144). Other loci likely affect beta cell regulatory activity, such as GLIS3 (145,146,147,148), 16p13 for DEXI and SOCS1 (104,105), and TNFSF18 (104,149). A subset of type 1 diabetes loci also affect pancreatic exocrine cell regulatory activity and function, for example, CFTR and CTRB1/2 (46,150,151,152). At the INS locus, the INS promoter VNTR (variable number of tandem repeats region) has been argued to affect expression of insulin in the thymus, as well as in beta cells (153,154,155,156,157), suggesting that it may have dual mechanisms.
Many additional candidate genes at type 1 diabetes loci affect relevant beta cell or immune cell phenotypes in cell or animal models, e.g., PTPN2 (158), RNLS (159), ERBB3 (160), TNFAIP3 (161,162), STAT6 (163), BCL2L11 (158), ALOX15 (164), CAMK4 (165,166), IL10 (167,168,169), IL2 (170,171), IL27 (172), and IL7R (173), but have not necessarily been linked to type 1 diabetes risk variant activity directly. For IL10, IL2, and IL7R, these are almost certainly the genes through which type 1 diabetes risk is mediated in humans given their ability in mouse models to directly cause or prevent type 1 diabetes, but risk variant effects on their activities remain unknown.
As additional studies profile the activity of protein-coding and noncoding risk variants, the interpretation of type 1 diabetes variant function will reveal novel mechanisms of disease risk that can be targeted for prevention and treatment.
Genes and Initiation of Type 1 Diabetes
Diagnosis of type 1 diabetes follows a preclinical phase of variable duration during which autoimmunity attacks beta cells in the pancreatic islets. As a consequence, beta cell function is reduced below the threshold required to maintain normal glucose concentration in blood. The discovery of biomarkers, namely detectable serum islet autoantibodies, enabled the exploration of this process and its contributors, including genetics. The first GWAS of islet autoantibody positivity in type 1 diabetes was conducted in T1DGC cases with measurement of two islet autoantibodies (glutamate decarboxylase [GAD] and insulinoma-associated antigen 2 [IA-2A]), antibodies to the autoimmune thyroid (Graves’) disease autoantigen thyroid peroxidase, and antibodies against gastric parietal cells (PCA) that are associated with autoimmune gastritis (174). Genome-wide, variants in FCRL3/1q23 were associated with IA-2A positivity, and variants in ABO/9q34 were associated with the presence of PCA (174). The T1DGC samples were obtained from individuals with type 1 diabetes (i.e., prevalent cases), which is a limitation because the influential genetic (and environmental factors) may vary along this process, and islet autoantibodies can become undetectable in individuals with long-duration type 1 diabetes. Therefore, the evaluation of genetic factors that initiate type 1 diabetes requires a different approach: namely, identifying and following initially nondiabetic individuals who will develop type 1 diabetes.
Since the incidence of type 1 diabetes in the general population is relatively low, studies aiming to identify individuals who will develop type 1 diabetes require extremely large sample sizes, which limits the feasibility of this research. To lower the estimated sample size, studies increase the expected incidence of the disease by focusing on individuals with higher risk. For example, The Environmental Determinants of Diabetes in the Young (TEDDY) study (175) longitudinally follows children with HLA genotypes known to increase the risk of type 1 diabetes. The Diabetes and Autoimmunity Study in the Young (DAISY) included a cohort with siblings or parents of children with type 1 diabetes and another cohort of children from the general population who carried high-risk HLA genotypes (13,176). The observational arm of the Type 1 Diabetes TrialNet study Pathway to Prevention follows autoantibody-positive relatives of individuals with type 1 diabetes (177). The Finnish Type 1 Diabetes Prediction and Prevention (DIPP) study longitudinally followed newborns carrying type 1 diabetes-associated HLA genotypes (178). These and other studies have provided invaluable insights on the genetic differences in the initiation and progression of type 1 diabetes.
In a study of almost 8,000 genetically at-risk infants participating in TEDDY, HLA-DR3-DQ2/DR4-DQ8 was the best predictor for the initiation of islet autoimmunity (179). Another analysis of TEDDY participants found additional genetic influences for autoimmunity initiation from non-HLA SNPs, such as rs2476601 in PTPN22, rs2292239 in erb-b2 receptor tyrosine kinase 3 (ERBB3), rs3184504 in SH2B adaptor protein 3 (SH2B3), and rs1004446 in INS (180). In the TEDDY cohort, PTPN22 rs2476601 was the best predictor for insulin autoantibodies appearing first and SNPs rs12708716 in CLEC16A and rs2292239 in ERBB3 for GAD autoantibodies as the first-appearing autoantibody (179). In DAISY, among non-HLA genes, the appearance of islet autoantibodies was associated with SNPs in PTPN22 (rs2476601) and UBASH3A (rs11203203 and rs9976767) (181,182).
The influence of HLA-DR3-DQ2/DR4-DQ8 was demonstrated to be stronger on the initiation of islet autoimmunity than on the progression to clinical diabetes (183,184). In approximately 3,400 participants in the TrialNet Pathway to Prevention study, HLA-DRB1*15:01-DQA1*01:02-DQB1*06:02 was shown to offer protection from islet autoimmunity initiation, as well as progression from single to multiple autoantibody positivity and then to type 1 diabetes, which occurs in very few individuals with this haplotype (185). Progression from single to multiple autoantibody positivity was influenced by HLA genotypes and rs3087243 in CTLA4, while diabetes progression was affected by HLA genotypes, rs6476839 in GLIS family zinc finger 3 (GLIS3), and rs3184504 in SH2B3 (186); in this study, age-related differential genetic influences were seen. DAISY also found that INS, UBASH3A, and IFIH1 were associated with progression from islet autoimmunity to diabetes (181,182). An analysis in the TrialNet Pathway to Prevention study demonstrated that type 2 diabetes-associated variants in the TCF7L2 locus influenced progression from single to multiple positivity with different effects depending on the autoantibody specificity and body mass index (BMI) (187), suggesting complex interactions among different diabetogenic mechanisms that may be present in a given individual. Critically, genome-wide (rather than candidate gene) approaches are necessary to interrogate and discover those variants contributing to initiation, as well as progression, of the autoimmune process.
Age at Onset of Type 1 Diabetes: Genetic Underpinnings
The age at onset of type 1 diabetes varies widely from infancy to adulthood. There have been conflicting reports of possible effects of type 1 diabetes risk variants on age at onset, largely from studies of modest sample size. However, the role of HLA alleles, particularly HLA-DR and HLA-DQ, on age at onset has been reported by multiple studies (188). Children who are diagnosed under age 6 years are more likely to have the HLA-DR3 allele and the HLA-DR3/DR4 genotype. For example, children who develop type 1 diabetes before age 7 years have increased insulitis, beta cell stress, and beta cell loss compared with older children and adolescents (189,190). TEDDY longitudinally follows newborns with high genetic risk for type 1 diabetes and has reported differences associated with the specific sequence of islet autoantibody seroconversion before the clinical onset of diabetes. In this study, insulin autoantibodies appeared first in very young children who carried HLA-DR4-DQ8 haplotypes and progressed rapidly to clinical disease, while GAD65 autoantibodies appeared first in older children who carried HLA-DR3-DQ2 (191,192). Among children with newly diagnosed type 1 diabetes, insulin autoantibody positivity and higher titers were associated with younger age at onset of type 1 diabetes, while GADA65 positivity was more common in females and those with thyroid peroxidase antibodies (193).
Well-powered studies allowed testing of the association of age of onset with type 1 diabetes-associated SNPs identified in GWAS and fine-mapping studies (194). Two loci, RNLS at 10q23.31 and IL2 at 4q27, displayed significant associations with age at onset. For IL2/4q27, the G allele for the rs2069763 variant is “protective”: that is, the age at diagnosis increases with the number of protective alleles (mean age at diagnosis for those with the TT genotype is 8.7 years, the GT genotype 9.0 years, and the GG genotype 9.3 years). This effect is also seen for the RNLS/10q23.3 rs10500540 variant, in that the mean age at diagnosis increases with the number of protective C alleles. The influence of HLA-DQB1*03:02 (tagged by rs9273363 SNP) on younger age of onset was confirmed by a GWAS that also demonstrated the effect on the same direction of the 6q22.33 region that contains the genes encoding protein tyrosine phosphatase receptor kappa (PTPRK) and thymocyte-expressed molecule involved in selection (THEMIS) (195). Furthermore, children with type 1 diabetes diagnosed under the age of 7 years had increased frequency of the HLA-DR3-DQ2/DR4-DQ8 genotype, A*2402 and B39*06 alleles, as well as non-HLA regions involved in the immune system (e.g., ILSRA, IL10, THEMIS, Ikaros family zinc finger 3 [IKZF3]) or the beta cell (e.g., GLIS3) (196).
Adults who present with type 1 diabetes can develop rapid insulin insufficiency, which is important for clinicians to bear in mind (197). However, most studies to date have shown that compared with childhood type 1 diabetes, adult-onset type 1 diabetes has weaker associations with HLA genotypes and stronger associations with INS and some loci associated with type 2 diabetes (198). Similarly, adult-onset type 1 diabetes seems to progress more slowly overall, and at the extreme of the spectrum, some individuals, although diagnosed with diabetes, do not become insulin dependent for months or years after clinical onset; this subtype of autoimmune type 1 diabetes is often referred to as latent autoimmune diabetes in adults (LADA). Genetically, LADA is closer to type 1 diabetes but has commonalities with type 2 diabetes (199). HLA genotypes typically associated with type 1 diabetes are less common in individuals who develop type 1 diabetes at an older age, with higher BMI, and lower number of islet autoantibodies. In these individuals, their relatively conserved C-peptide production suggests insulin resistance as a contributing factor to their diabetes (200). Furthermore, compared with individuals with multiple positive islet autoantibodies, those with single autoantibody positivity are more likely to carry a TCF7L2 genetic variant that confers strong risk for type 2 diabetes (18).
Overall, although clusters of characteristics suggest common pathogenic mechanisms, clear endotypes (defined as pathophysiologically distinct subtypes of disease that may respond differently to treatment (201)) have not emerged yet. Uncovering the function of genetic factors associated with type 1 diabetes may help improve the current understanding of the heterogeneity of diabetes with the ultimate goal of applying precision medicine, that is, applying “the right treatment, for each patient, at the right time” (202).
Genetics and Prediction of Type 1 Diabetes Risk
One of the goals of increasing the understanding of type 1 diabetes pathogenesis is to recognize the risk factors and biomarkers that can be used for prediction of disease. Individuals identified as having high risk for type 1 diabetes are less likely to present with diabetic ketoacidosis (203) and can participate in clinical trials to prevent diabetes (204), or if meeting the approved indications, they can receive a recently approved treatment with teplizumab to delay the onset of clinical type 1 diabetes (205).
The first attempts to predict type 1 diabetes began more than 30 years ago (206,207) after the discovery of assays to measure islet autoantibodies in serum and the knowledge of HLA genotypes that confer risk. Since then, multiple islet autoantibodies have been discovered, and high-throughput methods for standardized detection of islet autoantibodies in serum have been developed. Furthermore, it has become clear that other factors, in particular age, have a profound influence on risk and rate of progression and the characteristics of the disease. The trajectory of metabolic measures, e.g., C-peptide and glucose, before clinical onset of diabetes has also been described and used for prediction (208).
Improved understanding of the sequence of events in the natural history of type 1 diabetes allowed investigators to break down the preclinical course of type 1 diabetes into stages beginning with the expression of multiple islet autoantibodies, when the risk of developing clinical disease within 10 years is 70% and virtually 100% within 20 years (209). Therefore, stage 1 type 1 diabetes is marked by positivity for multiple islet autoantibodies and normal glucose metabolism; stage 2, by multiple autoantibody positivity and dysglycemia (i.e., subclinical abnormalities that do not meet criteria for diabetes); and stage 3, by glucose in the diabetic range (i.e., clinical type 1 diabetes) (Figure 7) (210). Although refinements to this system are likely to be developed, the concept of stages provides a framework for prediction and prevention of type 1 diabetes.

FIGURE 7.
Phases in the Natural History of Type 1 Diabetes. Abs, islet autoantibodies; IA, islet autoimmunity; T1D, type 1 diabetes.
Aiding in type 1 diabetes prediction, more than 90 genetic regions within and outside the HLA region that are associated with type 1 diabetes risk have been identified. As reviewed in the Genes and Initiation of Type 1 Diabetes section, genetic influences on the initiation of islet autoimmunity may differ from those that affect further progression of islet autoimmunity and, finally, onset of type 1 diabetes (179,181,182,185,186,187). There are also differences by age at seroconversion (191,192,194,195,196,198,199). This information has been integrated to predict the development of type 1 diabetes in individuals at various preclinical stages. Survival analysis-based estimation of risks of first autoantibody seroconversion, spread to multiple autoantibody positivity, and then progression to clinical type 1 diabetes demonstrated interactions, with multiplicative effects, of combinations of high-risk HLA genotypes (e.g., HLA-DR3-DQ2/DR4-DQ8) and non-HLA loci (e.g., INS, PTPN22) (211).
While metabolic milestones, such as the development of dysglycemia, are the strongest markers of short-term risk, the value of genetics is with long-term horizons before metabolic deterioration occurs (15). Similarly, genetics may have less predictive power in the presence of multiple islet autoantibodies or autoantibodies that reflect advanced progression (e.g., IA-2A autoantibodies). On the other hand, in earlier stages, genetics has the advantage of being permanent and, thus, not needing repeated assessment, as opposed to other indicators of risk, such as autoantibodies (212).
The higher prevalence of additional autoimmune conditions in individuals with type 1 diabetes may be explained, at least in part, by common genetic factors (213). For example, HLA-DR3-DQ2 and/or, to a lesser degree, HLA-DR4-DQ8 are found in most patients with celiac disease (214,215); HLA-DR4-DQ8 and non-HLA genes, such as PTPN22 and CTLA4, are associated with rheumatoid arthritis (216); and while autoimmune thyroid disease has specific genetic influences (e.g., thyroglobulin, thyrotropin receptor, and CD40), HLA-DR3-DQ2, HLA-DR4-DQ8, PTPN22, and CTLA4 are shared with type 1 diabetes (217). These commonalities highlight pathways that underlie autoimmunity in general and might offer the potential pragmatic use of predicting additional autoimmune diseases in individuals with type 1 diabetes.
Additional gaps in knowledge must be covered to optimize the use of genetics for type 1 diabetes prediction. As reviewed in previous sections, the specific genes that facilitate triggering of islet autoimmunity differ from the genes that regulate its progression and the loss of beta cell function (218), but the latter are less well known in the setting of type 1 diabetes. In addition, resolving discrepancies in results among studies with different populations and designs is still necessary. The genetic underpinnings of the differences marked by age are only beginning to be understood (195,196), and data remain scarce in adult-onset type 1 diabetes. Research in this area may reveal the differential pathogenic mechanisms that underlie the development of type 1 diabetes across the lifespan (219). Although most knowledge on the natural history of type 1 diabetes before clinical onset stems from observational studies conducted in participants with high genetic risk or a relative with type 1 diabetes, prediction models and screening strategies for type 1 diabetes risk must be tested in the general population (212,220). This endeavor has become particularly pressing since the demonstration of effective and safe interventions to delay the onset of type 1 diabetes in individuals with stage 2 type 1 diabetes (205).
An additional barrier to using genetics in type 1 diabetes for prediction and selection of candidates for clinical trials or standard treatment was the pragmatic problem of how to integrate the elevated number of genetic loci that contribute to the development of type 1 diabetes, with variable effect sizes, in the predictive models and algorithms. This need has been addressed by the development of type 1 diabetes genetic risk scores.
Type 1 Diabetes Genetic Risk Scores
Incorporating the influences of a very large number of genes for pragmatic uses was difficult. For a long time, the two highest risk HLA haplotypes, i.e., HLA-DR4-DQ8 and HLA-DR3, and the strongest protective haplotype, i.e., HLA-DR2-DQB1*06:02, were the only genetic factors commonly used in type 1 diabetes prediction. However, the development of polygenic scores that combine multiple genes known to affect type 1 diabetes risk into a single number (reviewed in Redondo et al. (221)) has facilitated the use of genetics in classification of diabetes and type 1 diabetes prediction.
Distinguishing between diabetes types can be a challenge because of the absence of pathognomonic features for type 1 or type 2 diabetes, which are the most frequent forms. Type 1 diabetes polygenic scores can help discriminate type 1 and type 2 diabetes (222), even in young adults (223) and children (224), and predict clinical course (225). These scores can also help distinguish between type 1 diabetes and monogenic diabetes (226). Since the constellation of characteristics used to diagnose type 1 diabetes was established in study cohorts of primarily European-ancestry individuals with pediatric-onset, clinically-diagnosed type 1 diabetes, misclassification is more frequent in individuals of non-European ancestry, adults, and those with atypical features. The addition of a type 1 diabetes polygenic score improves phenotypic algorithms to identify individuals with type 1 diabetes (227).
Type 1 diabetes polygenic scores have been successfully incorporated in the predictive model for type 1 diabetes in individuals at risk. A type 1 diabetes genetic risk score improved prediction over only HLA genotypes and other markers in children followed since birth (15,228) and in relatives of patients across the lifespan (14). As the genetics of type 1 diabetes in non-European ancestries are uncovered, investigators are developing ancestry-specific genetic risk scores, such as Japanese (229), African (60), and Chinese Han (61) specific scores (reviewed in Redondo et al. (27)). However, given the increasing frequency of individuals who have multiple ancestries, developing scores that can be used in populations of any ancestry would be beneficial, and thus, more studies in global populations are warranted.
Epigenetics of Type 1 Diabetes
In addition to genetic factors, epigenetic changes in the genome have been implicated in type 1 diabetes. Epigenetics refers to modifications that affect gene regulation without altering DNA sequence directly, such as methylation of cytosine residues or post-translational modification of histone tails (230). Cytosine methylation is found most prominently at CpG dinucleotides, which are preferentially located at gene promoters and are associated with gene repression (231). Histone tail residues can be modified in several ways, such as methylation or acetylation, and depending on the residue and modification, can lead to activated or repressed transcription (232). Importantly, epigenetic marks, including DNA methylation and histone modifications, reflect both genetic and external influences (233) and, therefore, can help capture disease processes driven by environmental factors.
A common strategy is to profile monozygotic twin pairs, which have nearly identical genome sequence, discordant for type 1 diabetes to determine whether differences in epigenetic profiles contribute to disease risk (234,235,236,237). For example, an epigenome-wide association study (EWAS) compared CpG site methylation in discordant twin pairs in T cells, B cells, and monocytes and found thousands of CpG sites in each cell type associated with type 1 diabetes (238). The variability in CpG site methylation did not appear to be due to genetic factors; therefore, environmental factors are a likely contributor to these changes.
Further studies have shown differences in immune cell-type CpG methylation in pre-stages of disease, such as in islet autoantibody-positive individuals, suggesting that methylation changes precede disease onset and may potentially be useful biomarkers (234,239,240). Methylation profiles of beta cell-specific genes detected in circulating blood have also been evaluated as biomarkers of beta cell death in type 1 diabetes (241,242,243).
In addition to DNA methylation, studies have profiled changes in histone modification in type 1 diabetes. For example, researchers have measured levels of histone modifications, such as dimethylation and acetylation of H3 lysine 9 (H3K9me2, H3K9ac), in lymphocytes and monocytes and found altered levels in type 1 diabetes compared to controls, including in type 1 diabetes risk loci, e.g., CTLA4 and class II MHC (244,245). Changes in histone modification levels, as well as histone acetyltransferase localization, have been observed in immune cells in disease-relevant environmental conditions, such as hyperglycemia (246,247).
A caveat to many of these studies is that epigenetic changes observed in type 1 diabetes may reflect consequences of the disease process that do not contribute to disease pathogenesis directly. Mapping epigenetic changes mediated by genetic risk variants can help to define which epigenetic features may play a causal role in disease. For example, CpG sites at the CTSH locus are affected by both environmental stimuli and genetic risk variants, supporting the hypothesis that methylation of these CpG sites may causally affect disease (248). Compared to genetic studies, the sample sizes of epigenetic studies have been relatively small, and consequently, results across different studies have had limited consistency. Therefore, larger studies are needed to enable well-powered analyses.
Conclusions
Type 1 diabetes is a complex disorder that results from the individual and, possibly, the combined effects of multiple genetic and environmental risk factors. The advent of high-throughput genotyping methodologies enabling GWAS combined with large collections of biospecimens from type 1 diabetes cases and unaffected control individuals has allowed the identification of a large number of genetic loci that contribute to type 1 diabetes risk. Fine-mapping studies building on GWAS results have substantially lowered the number of possible causal genes. Some genes involved in type 1 diabetes risk have been identified and have consistent and strong effects (e.g., HLA class I and class II loci or INS). Other genes have small individual contributions to risk; nevertheless, these genes may represent components in common biologic pathways with rate-limiting reactions or products that may be amenable to manipulation for therapy. While a subset of genes involved in type 1 diabetes risk are associated with changes to the protein product (e.g., PTPN22, TYK2, IFIH1, FUT2), most genes are affected by noncoding variants that modulate expression in disease-relevant cell types. Research on the function of protein-coding and noncoding variants associated with type 1 diabetes is shedding light on the pathophysiological mechanisms that underlie type 1 diabetes risk, as well as helping to dissect disease heterogeneity.
Future studies of the genetics of type 1 diabetes focusing on the relationship of genotype and phenotype would help elucidate the molecular, cellular, and organismal effects of individual risk variants on gene activity. As most variants affecting type 1 diabetes risk are located in noncoding regions of the genome, more detailed maps of regulatory elements and genes regulated by these elements would enable researchers to link risk variants to their gene targets. These studies, building upon unbiased searches for risk genes and variants, hold the promise of providing novel insights into the pathogenesis of type 1 diabetes, targets for potential preventive therapies, and markers of response that can be used to predict type 1 diabetes and select candidates who may respond to specific preventive agents. In addition, genetic studies in global populations, as well as the incorporation of ancestry in prediction models, would fill a gap in identifying individuals of any race or ethnicity who are at high risk of developing type 1 diabetes. Learning to use genetics, along with environmental factors, to screen for type 1 diabetes risk in the general population offers a key opportunity for finding those who are most likely to benefit from preventive therapies. The development of genetic risk scores and the lower cost of genotyping methods will facilitate the incorporation of genetics in clinical practice to help in diagnosis of diabetes type, prognosis of clinical course (e.g., C-peptide decline, development of additional autoimmune diseases, etc.), and potentially, selection of best clinical management approaches.
List of Abbreviations
- APC
antigen-presenting cell
- ASP
affected sib (sibling) pair
- BMI
body mass index
- caQTL
chromatin accessibility QTL
- DAISY
Diabetes and Autoimmunity Study in the Young
- DNA
deoxyribonucleic acid
- eQTL
expression QTL
- GAD
glutamate decarboxylase
- GWAS
genome-wide association study
- HLA
human leukocyte antigen
- hQTL
histone QTL
- IA-2A
insulinoma-associated antigen 2
- IBD
identity-by-descent
- INS
insulin gene
- LADA
latant autoimmune diabetes in adults
- LD
linkage disequilibrium
- MHC
major histocompatibility complex
- OR
odds ratio
- PCA
parietal cell antibodies
- QTL
quantitative trait locus
- SNP
single nucleotide polymorphism
- T1DGC
Type 1 Diabetes Genetics Consortium
- TCR
T cell receptor
- TEDDY
The Environmental Determinants of Diabetes in the Young study
- WTCCC
Wellcome Trust Case-Control Consortium
Funding
Support was provided by the National Institute of Diabetes and Digestive and Kidney Diseases grants DK124395 (MJR, SOG), DK121843 (MJR), DK114650 (KJG), DK122607 (KJG), and DK120429 (KJG).
Acknowledgment
This is an update of: Rich SS, Erlich H, Concannon P: Genetics of Type 1 Diabetes. Chapter 12 in Diabetes in America, 3rd ed. Cowie CC, Casagrande SS, Menke A, Cissell MA, Eberhardt MS, Meigs JB, Gregg EW, Knowler WC, Barrett-Connor E, Becker DJ, Brancati FL, Boyko EJ, Herman WH, Howard BV, Narayan KMV, Rewers M, Fradkin JE, Eds. Bethesda, MD, National Institutes of Health, NIH Pub No. 17-1468, 2018, p. 12.1–12.16
Article History
Received in final form on June 16, 2023.
References
- 1.
- Barnett AH, Eff C, Leslie RD, Pyke DA. Diabetes in identical twins. A study of 200 pairs. Diabetologia. 1981;20(2):87-93. doi:10.1007/BF00262007 [PubMed: 7193616] [CrossRef]
- 2.
- Redondo MJ, Jeffrey J, Fain PR, Eisenbarth GS, Orban T. Concordance for islet autoimmunity among monozygotic twins. N Engl J Med. 2008;359(26):2849-2850. doi:10.1056/NEJMc0805398 [PubMed: 19109586] [CrossRef]
- 3.
- Johnston C, Pyke DA, Cudworth AG, Wolf E. HLA-DR typing in identical twins with insulin-dependent diabetes: difference between concordant and discordant pairs. Br Med J (Clin Res Ed). 1983;286(6361):253-255. doi:10.1136/bmj.286.6361.253 [PMC free article: PMC1546460] [PubMed: 6402059] [CrossRef]
- 4.
- Metcalfe KA, Hitman GA, Rowe RE, et al. Concordance for type 1 diabetes in identical twins is affected by insulin genotype. Diabetes Care. 2001;24(5):838-842. doi:10.2337/diacare.24.5.838 [PubMed: 11347740] [CrossRef]
- 5.
- Hyttinen V, Kaprio J, Kinnunen L, Koskenvuo M, Tuomilehto J. Genetic liability of type 1 diabetes and the onset age among 22,650 young Finnish twin pairs: a nationwide follow-up study. Diabetes. 2003;52(4):1052-1055. doi:10.2337/diabetes.52.4.1052 [PubMed: 12663480] [CrossRef]
- 6.
- Triolo TM, Fouts A, Pyle L, et al. Identical and nonidentical twins: risk and factors involved in development of islet autoimmunity and type 1 diabetes. Diabetes Care. 2019;42(2):192-199. doi:10.2337/dc18-0288 [PMC free article: PMC6341285] [PubMed: 30061316] [CrossRef]
- 7.
- Lawrence JM, Divers J, Isom S, et al. Trends in prevalence of type 1 and type 2 diabetes in children and adolescents in the US, 2001–2017. JAMA. 2021;326(8):717-727. doi:10.1001/jama.2021.11165 [PMC free article: PMC8385600] [PubMed: 34427600] [CrossRef]
- 8.
- Wagenknecht LE, Lawrence JM, Isom S, et al. Trends in incidence of youth-onset type 1 and type 2 diabetes in the USA, 2002–18: results from the population-based SEARCH for Diabetes in Youth study. Lancet Diabetes Endocrinol. 2023;11(4):242-250. doi:10.1016/S2213-8587(23)00025-6 [PMC free article: PMC10091237] [PubMed: 36868256] [CrossRef]
- 9.
- Rogers MAM, Kim C, Banerjee T, Lee JM. Fluctuations in the incidence of type 1 diabetes in the United States from 2001 to 2015: a longitudinal study. BMC Med. 2017;15(1):199. doi:10.1186/s12916-017-0958-6 [PMC free article: PMC5688827] [PubMed: 29115947] [CrossRef]
- 10.
- Harding JL, Wander PL, Zhang X, et al. The incidence of adult-onset type 1 diabetes: a systematic review from 32 countries and regions. Diabetes Care. 2022;45(4):994-1006. doi:10.2337/dc21-1752 [PMC free article: PMC9016739] [PubMed: 35349653] [CrossRef]
- 11.
- Rich SS. Mapping genes in diabetes. Genetic epidemiological perspective. Diabetes. 1990;39(11):1315-1319. doi:10.2337/diab.39.11.1315 [PubMed: 2227105] [CrossRef]
- 12.
- Hagopian WA, Lernmark Å, Rewers MJ, et al. TEDDY—The Environmental Determinants of Diabetes in the Young: an observational clinical trial. Ann N Y Acad Sci. 2006;1079:320-326. doi:10.1196/annals.1375.049 [PubMed: 17130573] [CrossRef]
- 13.
- Rewers M, Bugawan TL, Norris JM, et al. Newborn screening for HLA markers associated with IDDM: Diabetes Autoimmunity Study in the Young (DAISY). Diabetologia. 1996;39(7):807-812. doi:10.1007/s001250050514 [PubMed: 8817105] [CrossRef]
- 14.
- Redondo MJ, Geyer S, Steck AK, et al. A type 1 diabetes genetic risk score predicts progression of islet autoimmunity and development of type 1 diabetes in individuals at risk. Diabetes Care. 2018;41(9):1887-1894. doi:10.2337/dc18-0087 [PMC free article: PMC6105323] [PubMed: 30002199] [CrossRef]
- 15.
- Ferrat LA, Vehik K, Sharp SA, et al. A combined risk score enhances prediction of type 1 diabetes among susceptible children. Nat Med. 2020;26(8):1247-1255. doi:10.1038/s41591-020-0930-4 [PMC free article: PMC7556983] [PubMed: 32770166] [CrossRef]
- 16.
- Winkler C, Haupt F, Heigermoser M, et al. Identification of infants with increased type 1 diabetes genetic risk for enrollment into primary prevention trials—GPPAD-02 study design and first results. Pediatr Diabetes. 2019;20(6):720-727. doi:10.1111/pedi.12870 [PMC free article: PMC6851563] [PubMed: 31192505] [CrossRef]
- 17.
- Krischer JP, Lynch KF, Schatz DA, et al. The 6 year incidence of diabetes-associated autoantibodies in genetically at-risk children: the TEDDY study. Diabetologia. 2015;58(5):980-987. doi:10.1007/s00125-015-3514-y [PMC free article: PMC4393776] [PubMed: 25660258] [CrossRef]
- 18.
- Redondo MJ, Geyer S, Steck AK, et al. TCF7L2 genetic variants contribute to phenotypic heterogeneity of type 1 diabetes. Diabetes Care. 2018;41(2):311-317. doi:10.2337/dc17-0961 [PMC free article: PMC5780048] [PubMed: 29025879] [CrossRef]
- 19.
- Sioofy-Khojine AB, Richardson SJ, Locke JM, et al. Detection of enterovirus RNA in peripheral blood mononuclear cells correlates with the presence of the predisposing allele of the type 1 diabetes risk gene IFIH1 and with disease stage. Diabetologia. 2022;65(10):1701-1709. doi:10.1007/s00125-022-05753-y [PMC free article: PMC9477938] [PubMed: 35867130] [CrossRef]
- 20.
- Rich SS, Erlich H, Concannon P. Genetics of Type 1 Diabetes. In: Cowie CC, Casagrande CS, Menke A, et al, eds. Diabetes in America. 3rd ed. National Institutes of Health; 2018:12.1-12.16:chap 12. Accessed December 8, 2023. https://www
​.ncbi.nlm ​.nih.gov/books/NBK567984/ - 21.
- HLA MHC Complex. Updated October 23, 2009. Accessed December 27, 2015. http://commons
​.wikimedia ​.org/wiki/File:HLA ​.svg#mediaviewer/File:HLA.svg - 22.
- Atkinson MA, Eisenbarth GS, Michels AW. Type 1 diabetes. Lancet. 2014;383(9911):69-82. doi:10.1016/S0140-6736(13)60591-7 [PMC free article: PMC4380133] [PubMed: 23890997] [CrossRef]
- 23.
- Singal DP, Blajchman MA. Histocompatibility (HL-A) antigens, lymphocytotoxic antibodies and tissue antibodies in patients with diabetes mellitus. Diabetes. 1973;22(6):429-432. doi:10.2337/diab.22.6.429 [PubMed: 4541338] [CrossRef]
- 24.
- Rich SS, Weitkamp LR, Barbosa J. Genetic heterogeneity of insulin-dependent (type I) diabetes mellitus: evidence from a study of extended haplotypes. Am J Hum Genet. 1984;36(5):1015-1023. [PMC free article: PMC1684521] [PubMed: 6594040]
- 25.
- Noble JA, Johnson J, Lane JA, Valdes AM. HLA class II genotyping of African American type 1 diabetic patients reveals associations unique to African haplotypes. Diabetes. 2013;62(9):3292-3299. doi:10.2337/db13-0094 [PMC free article: PMC3749336] [PubMed: 23801574] [CrossRef]
- 26.
- Howson JM, Roy MS, Zeitels L, Stevens H, Todd JA. HLA class II gene associations in African American type 1 diabetes reveal a protective HLA-DRB1*03 haplotype. Diabet Med. 2013;30(6):710-716. doi:10.1111/dme.12148 [PMC free article: PMC3709123] [PubMed: 23398374] [CrossRef]
- 27.
- Redondo MJ, Gignoux CR, Dabelea D, et al. Type 1 diabetes in diverse ancestries and the use of genetic risk scores. Lancet Diabetes Endocrinol. 2022;10(8):597-608. doi:10.1016/S2213-8587(22)00159-0 [PMC free article: PMC10024251] [PubMed: 35724677] [CrossRef]
- 28.
- Steenkiste A, Valdes AM, Feolo M, et al. 14th International HLA and Immunogenetics Workshop: report on the HLA component of type 1 diabetes. Tissue Antigens. 2007;69(Suppl 1):214-225. doi:10.1111/j.1399-0039.2006.00772.x [PubMed: 17445204] [CrossRef]
- 29.
- Noble JA, Valdes AM. Genetics of the HLA region in the prediction of type 1 diabetes. Curr Diab Rep. 2011;11(6):533-542. doi:10.1007/s11892-011-0223-x [PMC free article: PMC3233362] [PubMed: 21912932] [CrossRef]
- 30.
- Cucca F, Muntoni F, Lampis R, et al. Combinations of specific DRB1, DQA1, DQB1 haplotypes are associated with insulin-dependent diabetes mellitus in Sardinia. Hum Immunol. 1993;37(2):85-94. doi:10.1016/0198-8859(93)90146-r [PubMed: 8226139] [CrossRef]
- 31.
- Carcassi C, Trucco G, Trucco M, Contu L. A new HLA-DR2 extended haplotype is involved in insulin-dependent diabetes mellitus susceptibility. Hum Immunol. 1991;31(3):159-164. doi:10.1016/0198-8859(91)90021-z [PubMed: 1890017] [CrossRef]
- 32.
- Erlich HA, Valdes AM, McDevitt SL, et al. Next generation sequencing reveals the association of DRB3*02:02 with type 1 diabetes. Diabetes. 2013;62(7):2618-2622. doi:10.2337/db12-1387 [PMC free article: PMC3712046] [PubMed: 23462545] [CrossRef]
- 33.
- Hu X, Deutsch AJ, Lenz TL, et al. Additive and interaction effects at three amino acid positions in HLA-DQ and HLA-DR molecules drive type 1 diabetes risk. Nat Genet. 2015;47(8):898-905. doi:10.1038/ng.3353 [PMC free article: PMC4930791] [PubMed: 26168013] [CrossRef]
- 34.
- Lenz TL, Deutsch AJ, Han B, et al. Widespread non-additive and interaction effects within HLA loci modulate the risk of autoimmune diseases. Nat Genet. 2015;47(9):1085-1090. doi:10.1038/ng.3379 [PMC free article: PMC4552599] [PubMed: 26258845] [CrossRef]
- 35.
- Concannon P, Chen WM, Julier C, et al. Genome-wide scan for linkage to type 1 diabetes in 2,496 multiplex families from the Type 1 Diabetes Genetics Consortium. Diabetes. 2009;58(4):1018-1022. doi:10.2337/db08-1551 [PMC free article: PMC2661598] [PubMed: 19136655] [CrossRef]
- 36.
- Morahan G, Mehta M, James I, et al. Tests for genetic interactions in type 1 diabetes: linkage and stratification analyses of 4,422 affected sib-pairs. Diabetes. 2011;60(3):1030-1040. doi:10.2337/db10-1195 [PMC free article: PMC3046821] [PubMed: 21266329] [CrossRef]
- 37.
- Rich SS, Akolkar B, Concannon P, et al. Overview of the Type I Diabetes Genetics Consortium. Genes Immun. 2009;10(Suppl 1):S1-S4. doi:10.1038/gene.2009.84 [PMC free article: PMC2805448] [PubMed: 19956093] [CrossRef]
- 38.
- International HapMap Consortium. The International HapMap Project. Nature. 2003;426(6968):789-796. doi:10.1038/nature02168 [PubMed: 14685227] [CrossRef]
- 39.
- Smyth DJ, Cooper JD, Bailey R, et al. A genome-wide association study of nonsynonymous SNPs identifies a type 1 diabetes locus in the interferon-induced helicase (IFIH1) region. Nat Genet. 2006;38(6):617-619. doi:10.1038/ng1800 [PubMed: 16699517] [CrossRef]
- 40.
- Nejentsev S, Walker N, Riches D, Egholm M, Todd JA. Rare variants of IFIH1, a gene implicated in antiviral responses, protect against type 1 diabetes. Science. 2009;324(5925):387-389. doi:10.1126/science.1167728 [PMC free article: PMC2707798] [PubMed: 19264985] [CrossRef]
- 41.
- Wellcome Trust Case Control Consortium. Genome-wide association study of 14,000 cases of seven common diseases and 3,000 shared controls. Nature. 2007;447(7145):661-678. doi:10.1038/nature05911 [PMC free article: PMC2719288] [PubMed: 17554300] [CrossRef]
- 42.
- Hakonarson H, Grant SF, Bradfield JP, et al. A genome-wide association study identifies KIAA0350 as a type 1 diabetes gene. Nature. 2007;448(7153):591-594. doi:10.1038/nature06010 [PubMed: 17632545] [CrossRef]
- 43.
- Cotsapas C, Voight BF, Rossin E, et al. Pervasive sharing of genetic effects in autoimmune disease. PLoS Genet. 2011;7(8):e1002254. doi:10.1371/journal.pgen.1002254 [PMC free article: PMC3154137] [PubMed: 21852963] [CrossRef]
- 44.
- Barrett JC, Clayton DG, Concannon P, et al. Genome-wide association study and meta-analysis find that over 40 loci affect risk of type 1 diabetes. Nat Genet. 2009;41(6):703-707. doi:10.1038/ng.381 [PMC free article: PMC2889014] [PubMed: 19430480] [CrossRef]
- 45.
- Cooper JD, Howson JM, Smyth D, et al. Confirmation of novel type 1 diabetes risk loci in families. Diabetologia. 2012;55(4):996-1000. doi:10.1007/s00125-012-2450-3 [PMC free article: PMC3296014] [PubMed: 22278338] [CrossRef]
- 46.
- Chiou J, Geusz RJ, Okino ML, et al. Interpreting type 1 diabetes risk with genetics and single-cell epigenomics. Nature. 2021;594(7863):398-402. doi:10.1038/s41586-021-03552-w [PMC free article: PMC10560508] [PubMed: 34012112] [CrossRef]
- 47.
- Concannon P, Rich SS, Nepom GT. Genetics of type 1A diabetes. N Engl J Med. 2009;360(16):1646-1654. doi:10.1056/NEJMra0808284 [PubMed: 19369670] [CrossRef]
- 48.
- Onengut-Gumuscu S, Chen WM, Burren O, et al. Fine mapping of type 1 diabetes susceptibility loci and evidence for colocalization of causal variants with lymphoid gene enhancers. Nat Genet. 2015;47(4):381-386. doi:10.1038/ng.3245 [PMC free article: PMC4380767] [PubMed: 25751624] [CrossRef]
- 49.
- Trynka G, Hunt KA, Bockett NA, et al. Dense genotyping identifies and localizes multiple common and rare variant association signals in celiac disease. Nat Genet. 2011;43(12):1193-1201. doi:10.1038/ng.998 [PMC free article: PMC3242065] [PubMed: 22057235] [CrossRef]
- 50.
- Raychaudhuri S, Thomson BP, Remmers EF, et al. Genetic variants at CD28, PRDM1 and CD2/CD58 are associated with rheumatoid arthritis risk. Nat Genet. 2009;41(12):1313-1318. doi:10.1038/ng.479 [PMC free article: PMC3142887] [PubMed: 19898481] [CrossRef]
- 51.
- Anderson CA, Boucher G, Lees CW, et al. Meta-analysis identifies 29 additional ulcerative colitis risk loci, increasing the number of confirmed associations to 47. Nat Genet. 2011;43(3):246-252. doi:10.1038/ng.764 [PMC free article: PMC3084597] [PubMed: 21297633] [CrossRef]
- 52.
- McGovern DP, Gardet A, Törkvist L, et al. Genome-wide association identifies multiple ulcerative colitis susceptibility loci. Nat Genet. 2010;42(4):332-337. doi:10.1038/ng.549 [PMC free article: PMC3087600] [PubMed: 20228799] [CrossRef]
- 53.
- Franke A, McGovern DP, Barrett JC, et al. Genome-wide meta-analysis increases to 71 the number of confirmed Crohn’s disease susceptibility loci. Nat Genet. 2010;42(12):1118-1125. doi:10.1038/ng.717 [PMC free article: PMC3299551] [PubMed: 21102463] [CrossRef]
- 54.
- International Multiple Sclerosis Genetics Consortium, Wellcome Trust Case Control Consortium, Sawcer S, et al. Genetic risk and a primary role for cell-mediated immune mechanisms in multiple sclerosis. Nature. 2011;476(7359):214-219. doi:10.1038/nature10251 [PMC free article: PMC3182531] [PubMed: 21833088] [CrossRef]
- 55.
- Zhang Z, Duvefelt K, Svensson F, et al. Two genes encoding immune-regulatory molecules (LAG3 and IL7R) confer susceptibility to multiple sclerosis. Genes Immun. 2005;6(2):145-152. doi:10.1038/sj.gene.6364171 [PubMed: 15674389] [CrossRef]
- 56.
- Robertson CC, Inshaw JRJ, Onengut-Gumuscu S, et al. Fine-mapping, trans-ancestral and genomic analyses identify causal variants, cells, genes and drug targets for type 1 diabetes. Nat Genet. 2021;53(7):962-971. doi:10.1038/s41588-021-00880-5 [PMC free article: PMC8273124] [PubMed: 34127860] [CrossRef]
- 57.
- Taliun D, Harris DN, Kessler MD, et al. Sequencing of 53,831 diverse genomes from the NHLBI TOPMed Program. Nature. 2021;590(7845):290-299. doi:10.1038/s41586-021-03205-y [PMC free article: PMC7875770] [PubMed: 33568819] [CrossRef]
- 58.
- Martin AR, Kanai M, Kamatani Y, Okada Y, Neale BM, Daly MJ. Clinical use of current polygenic risk scores may exacerbate health disparities. Nat Genet. 2019;51(4):584-591. doi:10.1038/s41588-019-0379-x [PMC free article: PMC6563838] [PubMed: 30926966] [CrossRef]
- 59.
- Wojcik GL, Graff M, Nishimura KK, et al. Genetic analyses of diverse populations improves discovery for complex traits. Nature. 2019;570(7762):514-518. doi:10.1038/s41586-019-1310-4 [PMC free article: PMC6785182] [PubMed: 31217584] [CrossRef]
- 60.
- Onengut-Gumuscu S, Chen WM, Robertson CC, et al. Type 1 diabetes risk in African-ancestry participants and utility of an ancestry-specific genetic risk score. Diabetes Care. 2019;42(3):406-415. doi:10.2337/dc18-1727 [PMC free article: PMC6385701] [PubMed: 30659077] [CrossRef]
- 61.
- Zhu M, Xu K, Chen Y, et al. Identification of novel T1D risk loci and their association with age and islet function at diagnosis in autoantibody-positive T1D individuals: based on a two-stage genome-wide association study. Diabetes Care. 2019;42(8):1414-1421. doi:10.2337/dc18-2023 [PubMed: 31152121] [CrossRef]
- 62.
- Shirai Y, Nakanishi Y, Suzuki A, et al. Multi-trait and cross-population genome-wide association studies across autoimmune and allergic diseases identify shared and distinct genetic component. Ann Rheum Dis. 2022;81(9):1301-1312. doi:10.1136/annrheumdis-2022-222460 [PMC free article: PMC9380494] [PubMed: 35753705] [CrossRef]
- 63.
- Balcha SA, Demisse AG, Mishra R, et al. Type 1 diabetes in Africa: an immunogenetic study in the Amhara of North-West Ethiopia. Diabetologia. 2020;63(10):2158-2168. doi:10.1007/s00125-020-05229-x [PMC free article: PMC7476916] [PubMed: 32705316] [CrossRef]
- 64.
- Bugawan TL, Mirel DB, Valdes AM, Panelo A, Pozzilli P, Erlich HA. Association and interaction of the IL4R, IL4, and IL13 loci with type 1 diabetes among Filipinos. Am J Hum Genet. 2003;72(6):1505-1514. doi:10.1086/375655 [PMC free article: PMC1180310] [PubMed: 12748907] [CrossRef]
- 65.
- Rohana AG, Loh KC, Tin SK, et al. HLA-DQ A1, -DQB1 and -DRB1 gene polymorphism—in Malay type 1 diabetes mellitus patients and their use for risk prediction. Med J Malaysia. 2011;66(2):133-137. [PubMed: 22106694]
- 66.
- Emery LM, Babu S, Bugawan TL, et al. Newborn HLA-DR,DQ genotype screening: age- and ethnicity-specific type 1 diabetes risk estimates. Pediatr Diabetes. 2005;6(3):136-144. doi:10.1111/j.1399-543X.2005.00117.x [PMC free article: PMC1351310] [PubMed: 16109069] [CrossRef]
- 67.
- Bastarache L, Denny JC, Roden DM. Phenome-wide association studies. JAMA. 2022;327(1):75-76. doi:10.1001/jama.2021.20356 [PMC free article: PMC8880207] [PubMed: 34982132] [CrossRef]
- 68.
- Yu X, Sun JP, He Y, et al. Structure, inhibitor, and regulatory mechanism of Lyp, a lymphoid-specific tyrosine phosphatase implicated in autoimmune diseases. Proc Natl Acad Sci U S A. 2007;104(50):19767-19772. doi:10.1073/pnas.0706233104 [PMC free article: PMC2148373] [PubMed: 18056643] [CrossRef]
- 69.
- Bottini N, Vang T, Cucca F, Mustelin T. Role of PTPN22 in type 1 diabetes and other autoimmune diseases. Semin Immunol. 2006;18(4):207-213. doi:10.1016/j.smim.2006.03.008 [PubMed: 16697661] [CrossRef]
- 70.
- Sharp RC, Abdulrahim M, Naser ES, Naser SA. Genetic variations of PTPN2 and PTPN22: role in the pathogenesis of type 1 diabetes and Crohn’s disease. Front Cell Infect Microbiol. 2015;5:95. doi:10.3389/fcimb.2015.00095 [PMC free article: PMC4689782] [PubMed: 26734582] [CrossRef]
- 71.
- Sharp RC, Beg SA, Naser SA. Polymorphisms in Protein Tyrosine Phosphatase Non-Receptor Type 2 and 22 (PTPN2/22) are linked to hyper-proliferative T-cells and susceptibility to mycobacteria in rheumatoid arthritis. Front Cell Infect Microbiol. 2018;8:11. doi:10.3389/fcimb.2018.00011 [PMC free article: PMC5788942] [PubMed: 29423382] [CrossRef]
- 72.
- Fiorillo E, Orru V, Stanford SM, et al. Autoimmune-associated PTPN22 R620W variation reduces phosphorylation of lymphoid phosphatase on an inhibitory tyrosine residue. J Biol Chem. 2010;285(34):26506-26518. doi:10.1074/jbc.M110.111104 [PMC free article: PMC2924087] [PubMed: 20538612] [CrossRef]
- 73.
- Menard L, Saadoun D, Isnardi I, et al. The PTPN22 allele encoding an R620W variant interferes with the removal of developing autoreactive B cells in humans. J Clin Invest. 2011;121(9):3635-3644. doi:10.1172/JCI45790 [PMC free article: PMC3163953] [PubMed: 21804190] [CrossRef]
- 74.
- Habib T, Funk A, Rieck M, et al. Altered B cell homeostasis is associated with type I diabetes and carriers of the PTPN22 allelic variant. J Immunol. 2012;188(1):487-496. doi:10.4049/jimmunol.1102176 [PMC free article: PMC3670766] [PubMed: 22105996] [CrossRef]
- 75.
- Arechiga AF, Habib T, He Y, et al. Cutting edge: the PTPN22 allelic variant associated with autoimmunity impairs B cell signaling. J Immunol. 2009;182(6):3343-3347. doi:10.4049/jimmunol.0713370 [PMC free article: PMC2797545] [PubMed: 19265110] [CrossRef]
- 76.
- Marroqui L, Dos Santos RS, Fløyel T, et al. TYK2, a candidate gene for type 1 diabetes, modulates apoptosis and the innate immune response in human pancreatic beta-cells. Diabetes. 2015;64(11):3808-3817. doi:10.2337/db15-0362 [PubMed: 26239055] [CrossRef]
- 77.
- Murray PJ. The JAK-STAT signaling pathway: input and output integration. J Immunol. 2007;178(5):2623-2629. doi:10.4049/jimmunol.178.5.2623 [PubMed: 17312100] [CrossRef]
- 78.
- Tokarski JS, Zupa-Fernandez A, Tredup JA, et al. Tyrosine kinase 2-mediated signal transduction in T lymphocytes is blocked by pharmacological stabilization of its pseudokinase domain. J Biol Chem. 2015;290(17):11061-11074. doi:10.1074/jbc.M114.619502 [PMC free article: PMC4409266] [PubMed: 25762719] [CrossRef]
- 79.
- O’Sullivan LA, Liongue C, Lewis RS, Stephenson SE, Ward AC. Cytokine receptor signaling through the Jak-Stat-Socs pathway in disease. Mol Immunol. 2007;44(10):2497-2506. doi:10.1016/j.molimm.2006.11.025 [PubMed: 17208301] [CrossRef]
- 80.
- Dendrou CA, Cortes A, Shipman L, et al. Resolving TYK2 locus genotype-to-phenotype differences in autoimmunity. Sci Transl Med. 2016;8(363):363ra149. doi:10.1126/scitranslmed.aag1974 [PMC free article: PMC5737835] [PubMed: 27807284] [CrossRef]
- 81.
- Enerback C, Sandin C, Lambert S, et al. The psoriasis-protective TYK2 I684S variant impairs IL-12 stimulated pSTAT4 response in skin-homing CD4+ and CD8+ memory T-cells. Sci Rep. 2018;8(1):7043. doi:10.1038/s41598-018-25282-2 [PMC free article: PMC5935702] [PubMed: 29728633] [CrossRef]
- 82.
- Tokumasa N, Suto A, Kagami S, et al. Expression of Tyk2 in dendritic cells is required for IL-12, IL-23, and IFN-gamma production and the induction of Th1 cell differentiation. Blood. 2007;110(2):553-560. doi:10.1182/blood-2006-11-059246 [PubMed: 17395783] [CrossRef]
- 83.
- Martínez-Pomar N, Lanio N, Romo N, Lopez-Botet M, Matamoros N. Functional impact of A91V mutation of the PRF1 perforin gene. Hum Immunol. 2013;74(1):14-17. doi:10.1016/j.humimm.2012.10.011 [PubMed: 23073290] [CrossRef]
- 84.
- Voskoboinik I, Sutton VR, Ciccone A, et al. Perforin activity and immune homeostasis: the common A91V polymorphism in perforin results in both presynaptic and postsynaptic defects in function. Blood. 2007;110(4):1184-1190. doi:10.1182/blood-2007-02-072850 [PubMed: 17475905] [CrossRef]
- 85.
- Gorman JA, Hundhausen C, Errett JS, et al. The A946T variant of the RNA sensor IFIH1 mediates an interferon program that limits viral infection but increases the risk for autoimmunity. Nat Immunol. 2017;18(7):744-752. doi:10.1038/ni.3766 [PMC free article: PMC5697900] [PubMed: 28553952] [CrossRef]
- 86.
- Chistiakov DA, Voronova NV, Savost’Anov KV, Turakulov RI. Loss-of-function mutations E6 27X and I923V of IFIH1 are associated with lower poly(I:C)-induced interferon-beta production in peripheral blood mononuclear cells of type 1 diabetes patients. Hum Immunol. 2010;71(11):1128-1134. doi:10.1016/j.humimm.2010.08.005 [PubMed: 20736039] [CrossRef]
- 87.
- Sharp RC, Brown ME, Shapiro MR, Posgai AL, Brusko TM. The immunoregulatory role of the signal regulatory protein family and CD47 signaling pathway in type 1 diabetes. Front Immunol. 2021;12:739048. doi:10.3389/fimmu.2021.739048 [PMC free article: PMC8481641] [PubMed: 34603322] [CrossRef]
- 88.
- Smith MJ, Pastor L, Newman JRB, Concannon P. Genetic control of splicing at SIRPG modulates risk of type 1 diabetes. Diabetes. 2022;71(2):350-358. doi:10.2337/db21-0194 [PMC free article: PMC8914281] [PubMed: 34799406] [CrossRef]
- 89.
- Maslah N, Cassinat B, Verger E, Kiladjian JJ, Velazquez L. The role of LNK/SH2B3 genetic alterations in myeloproliferative neoplasms and other hematological disorders. Leukemia. 2017;31(8):1661-1670. doi:10.1038/leu.2017.139 [PubMed: 28484264] [CrossRef]
- 90.
- Murayama MA, Kakuta S, Inoue A, et al. CTRP6 is an endogenous complement regulator that can effectively treat induced arthritis. Nat Commun. 2015;6:8483. doi:10.1038/ncomms9483 [PMC free article: PMC4598845] [PubMed: 26404464] [CrossRef]
- 91.
- Lei X, Seldin MM, Little HC, Choy N, Klonisch T, Wong GW. C1q/TNF-related protein 6 (CTRP6) links obesity to adipose tissue inflammation and insulin resistance. J Biol Chem. 2017;292(36):14836-14850. doi:10.1074/jbc.M116.766808 [PMC free article: PMC5592665] [PubMed: 28726640] [CrossRef]
- 92.
- Domsgen E, Lind K, Kong L, et al. An IFIH1 gene polymorphism associated with risk for autoimmunity regulates canonical antiviral defence pathways in Coxsackievirus infected human pancreatic islets. Sci Rep. 2016;6:39378. doi:10.1038/srep39378 [PMC free article: PMC5175199] [PubMed: 28000722] [CrossRef]
- 93.
- Fløyel T, Brorsson C, Nielsen LB, et al. CTSH regulates beta-cell function and disease progression in newly diagnosed type 1 diabetes patients. Proc Natl Acad Sci U S A. 2014;111(28):10305-10310. doi:10.1073/pnas.1402571111 [PMC free article: PMC4104872] [PubMed: 24982147] [CrossRef]
- 94.
- Azad MB, Wade KH, Timpson NJ. FUT2 secretor genotype and susceptibility to infections and chronic conditions in the ALSPAC cohort. Wellcome Open Res. 2018;3:65. doi:10.12688/wellcomeopenres.14636.2 [PMC free article: PMC6171556] [PubMed: 30345375] [CrossRef]
- 95.
- Blackwell CC, Jonsdottir K, Hanson M, et al. Non-secretion of ABO antigens predisposing to infection by Neisseria meningitidis and Streptococcus pneumoniae. Lancet. 1986;2(8501):284-285. doi:10.1016/s0140-6736(86)92103-3 [PubMed: 2874307] [CrossRef]
- 96.
- Smyth DJ, Cooper JD, Howson JM, et al. FUT2 nonsecretor status links type 1 diabetes susceptibility and resistance to infection. Diabetes. 2011;60(11):3081-3084. doi:10.2337/db11-0638 [PMC free article: PMC3198057] [PubMed: 22025780] [CrossRef]
- 97.
- Anderson MS, Su MA. Aire and T cell development. Curr Opin Immunol. 2011;23(2):198-206. doi:10.1016/j.coi.2010.11.007 [PMC free article: PMC3073725] [PubMed: 21163636] [CrossRef]
- 98.
- ENCODE Project Consortium. An integrated encyclopedia of DNA elements in the human genome. Nature. 2012;489(7414):57-74. doi:10.1038/nature11247 [PMC free article: PMC3439153] [PubMed: 22955616] [CrossRef]
- 99.
- ENCODE Project Consortium, Moore JE, Purcaro MJ, et al. Expanded encyclopaedias of DNA elements in the human and mouse genomes. Nature. 2020;583(7818):699-710. doi:10.1038/s41586-020-2493-4 [PMC free article: PMC7410828] [PubMed: 32728249] [CrossRef]
- 100.
- Roadmap Epigenomics Consortium, Kundaje A, Meuleman W, et al. Integrative analysis of 111 reference human epigenomes. Nature. 2015;518(7539):317-330. doi:10.1038/nature14248 [PMC free article: PMC4530010] [PubMed: 25693563] [CrossRef]
- 101.
- Boix CA, James BT, Park YP, Meuleman W, Kellis M. Regulatory genomic circuitry of human disease loci by integrative epigenomics. Nature. 2021;590(7845):300-307. doi:10.1038/s41586-020-03145-z [PMC free article: PMC7875769] [PubMed: 33536621] [CrossRef]
- 102.
- Preissl S, Gaulton KJ, Ren B. Characterizing cis-regulatory elements using single-cell epigenomics. Nat Rev Genet. 2023;24(1):21-43. doi:10.1038/s41576-022-00509-1 [PMC free article: PMC9771884] [PubMed: 35840754] [CrossRef]
- 103.
- Farh KK, Marson A, Zhu J, et al. Genetic and epigenetic fine mapping of causal autoimmune disease variants. Nature. 2015;518(7539):337-343. doi:10.1038/nature13835 [PMC free article: PMC4336207] [PubMed: 25363779] [CrossRef]
- 104.
- Ramos-Rodríguez M, Raurell-Vila H, Colli ML, et al. The impact of proinflammatory cytokines on the beta-cell regulatory landscape provides insights into the genetics of type 1 diabetes. Nat Genet. 2019;51(11):1588-1595. doi:10.1038/s41588-019-0524-6 [PMC free article: PMC7040466] [PubMed: 31676868] [CrossRef]
- 105.
- Benaglio P, Zhu H, Okino ML, et al. Type 1 diabetes risk genes mediate pancreatic beta cell survival in response to proinflammatory cytokines. Cell Genom. 2022;2(12):100214. doi:10.1016/j.xgen.2022.100214 [PMC free article: PMC9903835] [PubMed: 36778047] [CrossRef]
- 106.
- Waszak SM, Delaneau O, Gschwind AR, et al. Population variation and genetic control of modular chromatin architecture in humans. Cell. 2015;162(5):1039-1050. doi:10.1016/j.cell.2015.08.001 [PubMed: 26300124] [CrossRef]
- 107.
- Gutierrez-Arcelus M, Lappalainen T, Montgomery SB, et al. Passive and active DNA methylation and the interplay with genetic variation in gene regulation. eLife. 2013;2:e00523. doi:10.7554/eLife.00523 [PMC free article: PMC3673336] [PubMed: 23755361] [CrossRef]
- 108.
- Chen L, Ge B, Casale FP, et al. Genetic drivers of epigenetic and transcriptional variation in human immune cells. Cell. 2016;167(5):1398-1414.e24. doi:10.1016/j.cell.2016.10.026 [PMC free article: PMC5119954] [PubMed: 27863251] [CrossRef]
- 109.
- Kumasaka N, Knights AJ, Gaffney DJ. Fine-mapping cellular QTLs with RASQUAL and ATAC-seq. Nat Genet. 2016;48(2):206-213. doi:10.1038/ng.3467 [PMC free article: PMC5098600] [PubMed: 26656845] [CrossRef]
- 110.
- McVicker G, van de Geijn B, Degner JF, et al. Identification of genetic variants that affect histone modifications in human cells. Science. 2013;342(6159):747-749. doi:10.1126/science.1242429 [PMC free article: PMC3947669] [PubMed: 24136359] [CrossRef]
- 111.
- Alasoo K, Rodrigues J, Mukhopadhyay S, et al. Shared genetic effects on chromatin and gene expression indicate a role for enhancer priming in immune response. Nat Genet. 2018;50(3):424-431. doi:10.1038/s41588-018-0046-7 [PMC free article: PMC6548559] [PubMed: 29379200] [CrossRef]
- 112.
- Tehranchi A, Hie B, Dacre M, et al. Fine-mapping cis-regulatory variants in diverse human populations. eLife. 2019;8:e39595. doi:10.7554/eLife.39595 [PMC free article: PMC6335058] [PubMed: 30650056] [CrossRef]
- 113.
- Inoue F, Ahituv N. Decoding enhancers using massively parallel reporter assays. Genomics. 2015;106(3):159-164. doi:10.1016/j.ygeno.2015.06.005 [PMC free article: PMC4540663] [PubMed: 26072433] [CrossRef]
- 114.
- Mouri K, Guo MH, de Boer CG, et al. Prioritization of autoimmune disease-associated genetic variants that perturb regulatory element activity in T cells. Nat Genet. 2022;54(5):603-612. doi:10.1038/s41588-022-01056-5 [PMC free article: PMC9793778] [PubMed: 35513721] [CrossRef]
- 115.
- Lee D, Gorkin DU, Baker M, et al. A method to predict the impact of regulatory variants from DNA sequence. Nat Genet. 2015;47(8):955-961. doi:10.1038/ng.3331 [PMC free article: PMC4520745] [PubMed: 26075791] [CrossRef]
- 116.
- Kelley DR, Snoek J, Rinn JL. Basset: learning the regulatory code of the accessible genome with deep convolutional neural networks. Genome Res. 2016;26(7):990-999. doi:10.1101/gr.200535.115 [PMC free article: PMC4937568] [PubMed: 27197224] [CrossRef]
- 117.
- Zhou J, Troyanskaya OG. Predicting effects of noncoding variants with deep learning-based sequence model. Nat Methods. 2015;12(10):931-934. doi:10.1038/nmeth.3547 [PMC free article: PMC4768299] [PubMed: 26301843] [CrossRef]
- 118.
- Gate RE, Cheng CS, Aiden AP, et al. Genetic determinants of co-accessible chromatin regions in activated T cells across humans. Nat Genet. 2018;50(8):1140-1150. doi:10.1038/s41588-018-0156-2 [PMC free article: PMC6097927] [PubMed: 29988122] [CrossRef]
- 119.
- Smemo S, Tena JJ, Kim KH, et al. Obesity-associated variants within FTO form long-range functional connections with IRX3. Nature. 2014;507(7492):371-375. doi:10.1038/nature13138 [PMC free article: PMC4113484] [PubMed: 24646999] [CrossRef]
- 120.
- Claussnitzer M, Dankel SN, Kim KH, et al. FTO obesity variant circuitry and adipocyte browning in humans. N Engl J Med. 2015;373(10):895-907. doi:10.1056/NEJMoa1502214 [PMC free article: PMC4959911] [PubMed: 26287746] [CrossRef]
- 121.
- Yu M, Ren B. The three-dimensional organization of mammalian genomes. Annu Rev Cell Dev Biol. 2017;33:265-289. doi:10.1146/annurev-cellbio-100616-060531 [PMC free article: PMC5837811] [PubMed: 28783961] [CrossRef]
- 122.
- Pliner HA, Packer JS, McFaline-Figueroa JL, et al. Cicero predicts cis-regulatory DNA interactions from single-cell chromatin accessibility data. Mol Cell. 2018;71(5):858-871.e8. doi:10.1016/j.molcel.2018.06.044 [PMC free article: PMC6582963] [PubMed: 30078726] [CrossRef]
- 123.
- Fulco CP, Nasser J, Jones TR, et al. Activity-by-contact model of enhancer-promoter regulation from thousands of CRISPR perturbations. Nat Genet. 2019;51(12):1664-1669. doi:10.1038/s41588-019-0538-0 [PMC free article: PMC6886585] [PubMed: 31784727] [CrossRef]
- 124.
- Nasser J, Bergman DT, Fulco CP, et al. Genome-wide enhancer maps link risk variants to disease genes. Nature. 2021;593(7858):238-243. doi:10.1038/s41586-021-03446-x [PMC free article: PMC9153265] [PubMed: 33828297] [CrossRef]
- 125.
- Anzalone AV, Koblan LW, Liu DR. Genome editing with CRISPR-Cas nucleases, base editors, transposases and prime editors. Nat Biotechnol. 2020;38(7):824-844. doi:10.1038/s41587-020-0561-9 [PubMed: 32572269] [CrossRef]
- 126.
- Nakamura M, Gao Y, Dominguez AA, Qi LS. CRISPR technologies for precise epigenome editing. Nat Cell Biol. 2021;23(1):11-22. doi:10.1038/s41556-020-00620-7 [PubMed: 33420494] [CrossRef]
- 127.
- Javierre BM, Burren OS, Wilder SP, et al. Lineage-specific genome architecture links enhancers and non-coding disease variants to target gene promoters. Cell. 2016;167(5):1369-1384.e19. doi:10.1016/j.cell.2016.09.037 [PMC free article: PMC5123897] [PubMed: 27863249] [CrossRef]
- 128.
- GTEx Consortium. The GTEx Consortium atlas of genetic regulatory effects across human tissues. Science. 2020;369(6509):1318-1330. doi:10.1126/science.aaz1776 [PMC free article: PMC7737656] [PubMed: 32913098] [CrossRef]
- 129.
- Wallace C, Rotival M, Cooper JD, et al. Statistical colocalization of monocyte gene expression and genetic risk variants for type 1 diabetes. Hum Mol Genet. 2012;21(12):2815-2824. doi:10.1093/hmg/dds098 [PMC free article: PMC3363338] [PubMed: 22403184] [CrossRef]
- 130.
- Bossini-Castillo L, Glinos DA, Kunowska N, et al. Immune disease variants modulate gene expression in regulatory CD4(+) T cells. Cell Genom. 2022;2(4). doi:10.1016/j.xgen.2022.100117 [PMC free article: PMC9010307] [PubMed: 35591976] [CrossRef]
- 131.
- Vella A, Cooper JD, Lowe CE, et al. Localization of a type 1 diabetes locus in the IL2RA/CD25 region by use of tag single-nucleotide polymorphisms. Am J Hum Genet. 2005;76(5):773-779. doi:10.1086/429843 [PMC free article: PMC1199367] [PubMed: 15776395] [CrossRef]
- 132.
- Boyman O, Sprent J. The role of interleukin-2 during homeostasis and activation of the immune system. Nat Rev Immunol. 2012;12(3):180-190. doi:10.1038/nri3156 [PubMed: 22343569] [CrossRef]
- 133.
- Garg G, Tyler JR, Yang JH, et al. Type 1 diabetes-associated IL2RA variation lowers IL-2 signaling and contributes to diminished CD4+CD25+ regulatory T cell function. J Immunol. 2012;188(9):4644-4653. doi:10.4049/jimmunol.1100272 [PMC free article: PMC3378653] [PubMed: 22461703] [CrossRef]
- 134.
- Yamanouchi J, Rainbow D, Serra P, et al. Interleukin-2 gene variation impairs regulatory T cell function and causes autoimmunity. Nat Genet. 2007;39(3):329-337. doi:10.1038/ng1958 [PMC free article: PMC2886969] [PubMed: 17277778] [CrossRef]
- 135.
- Chen Y, Chen S, Gu Y, et al. CTLA-4 +49 G/A, a functional T1D risk SNP, affects CTLA-4 level in Treg subsets and IA-2A positivity, but not beta-cell function. Sci Rep. 2018;8(1):10074. doi:10.1038/s41598-018-28423-9 [PMC free article: PMC6031668] [PubMed: 29973665] [CrossRef]
- 136.
- Ueda H, Howson JM, Esposito L, et al. Association of the T-cell regulatory gene CTLA4 with susceptibility to autoimmune disease. Nature. 2003;423(6939):506-511. doi:10.1038/nature01621 [PubMed: 12724780] [CrossRef]
- 137.
- Atabani SF, Thio CL, Divanovic S, et al. Association of CTLA4 polymorphism with regulatory T cell frequency. Eur J Immunol. 2005;35(7):2157-2162. doi:10.1002/eji.200526168 [PubMed: 15940668] [CrossRef]
- 138.
- Gerold KD, Zheng P, Rainbow DB, Zernecke A, Wicker LS, Kissler S. The soluble CTLA-4 splice variant protects from type 1 diabetes and potentiates regulatory T-cell function. Diabetes. 2011;60(7):1955-1963. doi:10.2337/db11-0130 [PMC free article: PMC3121435] [PubMed: 21602513] [CrossRef]
- 139.
- Stumpf M, Zhou X, Bluestone JA. The B7-independent isoform of CTLA-4 functions to regulate autoimmune diabetes. J Immunol. 2013;190(3):961-969. doi:10.4049/jimmunol.1201362 [PMC free article: PMC3568535] [PubMed: 23293354] [CrossRef]
- 140.
- Vuckovic D, Bao EL, Akbari P, et al. The polygenic and monogenic basis of blood traits and diseases. Cell. 2020;182(5):1214-1231.e11. doi:10.1016/j.cell.2020.08.008 [PMC free article: PMC7482360] [PubMed: 32888494] [CrossRef]
- 141.
- Yan J, Qiu Y, Ribeiro Dos Santos AM, et al. Systematic analysis of binding of transcription factors to noncoding variants. Nature. 2021;591(7848):147-151. doi:10.1038/s41586-021-03211-0 [PMC free article: PMC9367673] [PubMed: 33505025] [CrossRef]
- 142.
- Ge Y, Paisie TK, Newman JRB, McIntyre LM, Concannon P. UBASH3A mediates risk for type 1 diabetes through inhibition of T-cell receptor-induced NF-kappaB signaling. Diabetes. 2017;66(7):2033-2043. doi:10.2337/db16-1023 [PMC free article: PMC5482087] [PubMed: 28607106] [CrossRef]
- 143.
- Ge Y, Concannon P. Molecular-genetic characterization of common, noncoding UBASH3A variants associated with type 1 diabetes. Eur J Hum Genet. 2018;26(7):1060-1064. doi:10.1038/s41431-018-0123-5 [PMC free article: PMC6018660] [PubMed: 29491471] [CrossRef]
- 144.
- Tsukumo S, Unno M, Muto A, et al. Bach2 maintains T cells in a naive state by suppressing effector memory-related genes. Proc Natl Acad Sci U S A. 2013;110(26):10735-10740. doi:10.1073/pnas.1306691110 [PMC free article: PMC3696756] [PubMed: 23754397] [CrossRef]
- 145.
- Scoville D, Lichti-Kaiser K, Grimm S, Jetten A. GLIS3 binds pancreatic beta cell regulatory regions alongside other islet transcription factors. J Endocrinol. 2019;243(1):1-14. doi:10.1530/JOE-19-0182 [PMC free article: PMC6938561] [PubMed: 31340201] [CrossRef]
- 146.
- Scoville DW, Jetten AM. GLIS3: a critical transcription factor in islet beta-cell generation. Cells. 2021;10(12):3471. doi:10.3390/cells10123471 [PMC free article: PMC8700524] [PubMed: 34943978] [CrossRef]
- 147.
- Nogueira TC, Paula FM, Villate O, et al. GLIS3, a susceptibility gene for type 1 and type 2 diabetes, modulates pancreatic beta cell apoptosis via regulation of a splice variant of the BH3-only protein Bim. PLoS Genet. 2013;9(5):e1003532. doi:10.1371/journal.pgen.1003532 [PMC free article: PMC3667755] [PubMed: 23737756] [CrossRef]
- 148.
- Aylward A, Chiou J, Okino ML, Kadakia N, Gaulton KJ. Shared genetic risk contributes to type 1 and type 2 diabetes etiology. Hum Mol Genet. 2018. doi:10.1093/hmg/ddy314 [PubMed: 30407494] [CrossRef]
- 149.
- Ray A, Basu S, Williams CB, Salzman NH, Dittel BN. A novel IL-10-independent regulatory role for B cells in suppressing autoimmunity by maintenance of regulatory T cells via GITR ligand. J Immunol. 2012;188(7):3188-3198. doi:10.4049/jimmunol.1103354 [PMC free article: PMC3311743] [PubMed: 22368274] [CrossRef]
- 150.
- ‘t Hart LM, Fritsche A, Nijpels G, et al. The CTRB1/2 locus affects diabetes susceptibility and treatment via the incretin pathway. Diabetes. 2013;62(9):3275-3281. doi:10.2337/db13-0227 [PMC free article: PMC3749354] [PubMed: 23674605] [CrossRef]
- 151.
- Yazdanpanah N, Yazdanpanah M, Wang Y, et al. Clinically relevant circulating protein biomarkers for type 1 diabetes: evidence from a two-sample Mendelian randomization study. Diabetes Care. 2022;45(1):169-177. doi:10.2337/dc21-1049 [PubMed: 34758976] [CrossRef]
- 152.
- Emilsson V, Gudmundsdottir V, Gudjonsson A, et al. Coding and regulatory variants are associated with serum protein levels and disease. Nat Commun. 2022;13(1):481. doi:10.1038/s41467-022-28081-6 [PMC free article: PMC8789809] [PubMed: 35079000] [CrossRef]
- 153.
- Vafiadis P, Bennett ST, Todd JA, et al. Insulin expression in human thymus is modulated by INS VNTR alleles at the IDDM2 locus. Nat Genet. 1997;15(3):289-292. doi:10.1038/ng0397-289 [PubMed: 9054944] [CrossRef]
- 154.
- Vafiadis P, Bennett ST, Colle E, Grabs R, Goodyer CG, Polychronakos C. Imprinted and genotype-specific expression of genes at the IDDM2 locus in pancreas and leucocytes. J Autoimmun. 1996;9(3):397-403. doi:10.1006/jaut.1996.0054 [PubMed: 8816977] [CrossRef]
- 155.
- Durinovic-Belló I, Wu RP, Gersuk VH, Sanda S, Shilling HG, Nepom GT. Insulin gene VNTR genotype associates with frequency and phenotype of the autoimmune response to proinsulin. Genes Immun. 2010;11(2):188-193. doi:10.1038/gene.2009.108 [PMC free article: PMC2845516] [PubMed: 20054344] [CrossRef]
- 156.
- Durinovic-Belló I, Jelinek E, Schlosser M, et al. Class III alleles at the insulin VNTR polymorphism are associated with regulatory T-cell responses to proinsulin epitopes in HLA-DR4, DQ8 individuals. Diabetes. 2005;54(Suppl 2):S18-S24. doi:10.2337/diabetes.54.suppl_2.s18 [PubMed: 16306335] [CrossRef]
- 157.
- Wang S, Flibotte S, Camunas-Soler J, MacDonald PE, Johnson JD. A new hypothesis for type 1 diabetes risk: the at-risk allele at rs3842753 associates with increased beta-cell INS messenger RNA in a meta-analysis of single-cell RNA-sequencing data. Can J Diabetes. 2021;45(8):775-784.e2. doi:10.1016/j.jcjd.2021.03.007 [PubMed: 34052132] [CrossRef]
- 158.
- Santin I, Moore F, Colli ML, et al. PTPN2, a candidate gene for type 1 diabetes, modulates pancreatic beta-cell apoptosis via regulation of the BH3-only protein Bim. Diabetes. 2011;60(12):3279-3288. doi:10.2337/db11-0758 [PMC free article: PMC3219938] [PubMed: 21984578] [CrossRef]
- 159.
- Cai EP, Ishikawa Y, Zhang W, et al. Genome-scale in vivo CRISPR screen identifies RNLS as a target for beta cell protection in type 1 diabetes. Nat Metab. 2020;2(9):934-945. doi:10.1038/s42255-020-0254-1 [PMC free article: PMC7502486] [PubMed: 32719542] [CrossRef]
- 160.
- Kaur S, Mirza AH, Brorsson CA, et al. The genetic and regulatory architecture of ERBB3-type 1 diabetes susceptibility locus. Mol Cell Endocrinol. 2016;419:83-91. doi:10.1016/j.mce.2015.10.002 [PubMed: 26450151] [CrossRef]
- 161.
- Cheng L, Zhang D, Chen B. Tumor necrosis factor alpha-induced protein-3 protects zinc transporter 8 against proinflammatory cytokine-induced downregulation. Exp Ther Med. 2016;12(3):1509-1514. doi:10.3892/etm.2016.3457 [PMC free article: PMC4997978] [PubMed: 27588072] [CrossRef]
- 162.
- Tavares RM, Turer EE, Liu CL, et al. The ubiquitin modifying enzyme A20 restricts B cell survival and prevents autoimmunity. Immunity. 2010;33(2):181-191. doi:10.1016/j.immuni.2010.07.017 [PMC free article: PMC2931361] [PubMed: 20705491] [CrossRef]
- 163.
- Leslie KA, Russell MA, Taniguchi K, Richardson SJ, Morgan NG. The transcription factor STAT6 plays a critical role in promoting beta cell viability and is depleted in islets of individuals with type 1 diabetes. Diabetologia. 2019;62(1):87-98. doi:10.1007/s00125-018-4750-8 [PMC free article: PMC6290857] [PubMed: 30338340] [CrossRef]
- 164.
- Piñeros AR, Kulkarni A, Gao H, et al. Proinflammatory signaling in islet beta cells propagates invasion of pathogenic immune cells in autoimmune diabetes. Cell Rep. 2022;39(13):111011. doi:10.1016/j.celrep.2022.111011 [PMC free article: PMC9297711] [PubMed: 35767947] [CrossRef]
- 165.
- Koga T, Ichinose K, Mizui M, Crispin JC, Tsokos GC. Calcium/calmodulin-dependent protein kinase IV suppresses IL-2 production and regulatory T cell activity in lupus. J Immunol. 2012;189(7):3490-3496. doi:10.4049/jimmunol.1201785 [PMC free article: PMC3448834] [PubMed: 22942433] [CrossRef]
- 166.
- Koga T, Kawakami A. The role of CaMK4 in immune responses. Mod Rheumatol. 2018;28(2):211-214. doi:10.1080/14397595.2017.1413964 [PubMed: 29252071] [CrossRef]
- 167.
- Xu A, Zhu W, Li T, et al. Interleukin-10 gene transfer into insulin-producing beta cells protects against diabetes in non-obese diabetic mice. Mol Med Rep. 2015;12(3):3881-3889. doi:10.3892/mmr.2015.3809 [PubMed: 25998958] [CrossRef]
- 168.
- Yang Z, Chen M, Wu R, et al. Suppression of autoimmune diabetes by viral IL-10 gene transfer. J Immunol. 2002;168(12):6479-6485. doi:10.4049/jimmunol.168.12.6479 [PubMed: 12055268] [CrossRef]
- 169.
- Lu J, Liu J, Li L, Lan Y, Liang Y. Cytokines in type 1 diabetes: mechanisms of action and immunotherapeutic targets. Clin Transl Immunology. 2020;9(3):e1122. doi:10.1002/cti2.1122 [PMC free article: PMC7074462] [PubMed: 32185024] [CrossRef]
- 170.
- Hulme MA, Wasserfall CH, Atkinson MA, Brusko TM. Central role for interleukin-2 in type 1 diabetes. Diabetes. 2012;61(1):14-22. doi:10.2337/db11-1213 [PMC free article: PMC3237657] [PubMed: 22187370] [CrossRef]
- 171.
- Grinberg-Bleyer Y, Baeyens A, You S, et al. IL-2 reverses established type 1 diabetes in NOD mice by a local effect on pancreatic regulatory T cells. J Exp Med. 2010;207(9):1871-1878. doi:10.1084/jem.20100209 [PMC free article: PMC2931175] [PubMed: 20679400] [CrossRef]
- 172.
- Ciecko AE, Foda B, Barr JY, et al. Interleukin-27 is essential for type 1 diabetes development and Sjogren syndrome-like inflammation. Cell Rep. 2019;29(10):3073-3086.e5. doi:10.1016/j.celrep.2019.11.010 [PMC free article: PMC6914223] [PubMed: 31801074] [CrossRef]
- 173.
- Lee LF, Logronio K, Tu GH, et al. Anti-IL-7 receptor-alpha reverses established type 1 diabetes in nonobese diabetic mice by modulating effector T-cell function. Proc Natl Acad Sci U S A. 2012;109(31):12674-12679. doi:10.1073/pnas.1203795109 [PMC free article: PMC3412026] [PubMed: 22733769] [CrossRef]
- 174.
- Plagnol V, Howson JM, Smyth DJ, et al. Genome-wide association analysis of autoantibody positivity in type 1 diabetes cases. PLoS Genet. 2011;7(8):e1002216. doi:10.1371/journal.pgen.1002216 [PMC free article: PMC3150451] [PubMed: 21829393] [CrossRef]
- 175.
- Elding Larsson H, Vehik K, Gesualdo P, et al. Children followed in the TEDDY study are diagnosed with type 1 diabetes at an early stage of disease. Pediatr Diabetes. 2014;15(2):118-126. doi:10.1111/pedi.12066 [PMC free article: PMC3866211] [PubMed: 24034790] [CrossRef]
- 176.
- Rewers M, Norris JM, Eisenbarth GS, et al. Beta-cell autoantibodies in infants and toddlers without IDDM relatives: Diabetes Autoimmunity Study in the Young (DAISY). J Autoimmun. 1996;9(3):405-410. doi:10.1006/jaut.1996.0055 [PubMed: 8816978] [CrossRef]
- 177.
- Mahon JL, Sosenko JM, Rafkin-Mervis L, et al. The TrialNet Natural History Study of the Development of Type 1 Diabetes: objectives, design, and initial results. Pediatr Diabetes. 2009;10(2):97-104. doi:10.1111/j.1399-5448.2008.00464.x [PubMed: 18823409] [CrossRef]
- 178.
- Nejentsev S, Sjöroos M, Soukka T, et al. Population-based genetic screening for the estimation of type 1 diabetes mellitus risk in Finland: selective genotyping of markers in the HLA-DQB1, HLA-DQA1 and HLA-DRB1 loci. Diabet Med. 1999;16(12):985-992. doi:10.1046/j.1464-5491.1999.00186.x [PubMed: 10656226] [CrossRef]
- 179.
- Krischer JP, Liu X, Lernmark Å, et al. Predictors of the initiation of islet autoimmunity and progression to multiple autoantibodies and clinical diabetes: the TEDDY study. Diabetes Care. 2022;45(10):2271-2281. doi:10.2337/dc21-2612 [PMC free article: PMC9643148] [PubMed: 36150053] [CrossRef]
- 180.
- Törn C, Hadley D, Lee HS, et al. Role of type 1 diabetes-associated SNPs on risk of autoantibody positivity in the TEDDY study. Diabetes. 2015;64(5):1818-1829. doi:10.2337/db14-1497 [PMC free article: PMC4407865] [PubMed: 25422107] [CrossRef]
- 181.
- Steck AK, Dong F, Wong R, et al. Improving prediction of type 1 diabetes by testing non-HLA genetic variants in addition to HLA markers. Pediatr Diabetes. 2014;15(5):355-362. doi:10.1111/pedi.12092 [PMC free article: PMC4116638] [PubMed: 25075402] [CrossRef]
- 182.
- Johnson K, Wong R, Barriga KJ, et al. rs11203203 is associated with type 1 diabetes risk in population pre-screened for high-risk HLA-DR,DQ genotypes. Pediatr Diabetes. 2012;13(8):611-615. doi:10.1111/j.1399-5448.2012.00888.x [PMC free article: PMC4886718] [PubMed: 22776074] [CrossRef]
- 183.
- Ilonen J, Kiviniemi M, Lempainen J, et al. Genetic susceptibility to type 1 diabetes in childhood - estimation of HLA class II associated disease risk and class II effect in various phases of islet autoimmunity. Pediatr Diabetes. 2016;17(Suppl 22):8-16. doi:10.1111/pedi.12327 [PubMed: 27411431] [CrossRef]
- 184.
- Redondo MJ, Babu S, Zeidler A, et al. Specific human leukocyte antigen DQ influence on expression of antiislet autoantibodies and progression to type 1 diabetes. J Clin Endocrinol Metab. 2006;91(5):1705-1713. doi:10.1210/jc.2005-1695 [PubMed: 16464953] [CrossRef]
- 185.
- Pugliese A, Boulware D, Yu L, et al. HLA-DRB1*15:01-DQA1*01:02-DQB1*06:02 haplotype protects autoantibody-positive relatives from type 1 diabetes throughout the stages of disease progression. Diabetes. 2016;65(4):1109-1119. doi:10.2337/db15-1105 [PMC free article: PMC4806662] [PubMed: 26822082] [CrossRef]
- 186.
- Steck AK, Xu P, Geyer S, et al. Can non-HLA single nucleotide polymorphisms help stratify risk in TrialNet relatives at risk for type 1 diabetes? J Clin Endocrinol Metab. 2017;102(8):2873-2880. doi:10.1210/jc.2016-4003 [PMC free article: PMC5546868] [PubMed: 28520980] [CrossRef]
- 187.
- Redondo MJ, Steck AK, Sosenko J, et al. Transcription Factor 7-Like 2 (TCF7L2) gene polymorphism and progression from single to multiple autoantibody positivity in individuals at risk for type 1 diabetes. Diabetes Care. 2018;41(12):2480-2486. doi:10.2337/dc18-0861 [PMC free article: PMC6245213] [PubMed: 30275285] [CrossRef]
- 188.
- Tait BD, Harrison LC, Drummond BP, Stewart V, Varney MD, Honeyman MC. HLA antigens and age at diagnosis of insulin-dependent diabetes mellitus. Hum Immunol. 1995;42(2):116-122. doi:10.1016/0198-8859(94)00075-2 [PubMed: 7744614] [CrossRef]
- 189.
- Leete P, Willcox A, Krogvold L, et al. Differential insulitic profiles determine the extent of beta-cell destruction and the age at onset of type 1 diabetes. Diabetes. 2016;65(5):1362-1369. doi:10.2337/db15-1615 [PubMed: 26858360] [CrossRef]
- 190.
- Leete P, Oram RA, McDonald TJ, et al. Studies of insulin and proinsulin in pancreas and serum support the existence of aetiopathological endotypes of type 1 diabetes associated with age at diagnosis. Diabetologia. 2020;63(6):1258-1267. doi:10.1007/s00125-020-05115-6 [PMC free article: PMC7228905] [PubMed: 32172310] [CrossRef]
- 191.
- Krischer JP, Liu X, Vehik K, et al. Predicting islet cell autoimmunity and type 1 diabetes: an 8-year TEDDY study progress report. Diabetes Care. 2019;42(6):1051-1060. doi:10.2337/dc18-2282 [PMC free article: PMC6609953] [PubMed: 30967432] [CrossRef]
- 192.
- Vehik K, Bonifacio E, Lernmark Å, et al. Hierarchical order of distinct autoantibody spreading and progression to type 1 diabetes in the TEDDY study. Diabetes Care. 2020;43(9):2066-2073. doi:10.2337/dc19-2547 [PMC free article: PMC7440899] [PubMed: 32641373] [CrossRef]
- 193.
- Nieto J, Castillo B, Astudillo M, et al. Islet autoantibody types mark differential clinical characteristics at diagnosis of pediatric type 1 diabetes. Pediatr Diabetes. 2021;22(6):882-888. doi:10.1111/pedi.13238 [PubMed: 34018301] [CrossRef]
- 194.
- Howson JM, Cooper JD, Smyth DJ, et al. Evidence of gene-gene interaction and age-at-diagnosis effects in type 1 diabetes. Diabetes. 2012;61(11):3012-3017. doi:10.2337/db11-1694 [PMC free article: PMC3478521] [PubMed: 22891215] [CrossRef]
- 195.
- Inshaw JRJ, Walker NM, Wallace C, Bottolo L, Todd JA. The chromosome 6q22.33 region is associated with age at diagnosis of type 1 diabetes and disease risk in those diagnosed under 5 years of age. Diabetologia. 2018;61(1):147-157. doi:10.1007/s00125-017-4440-y [PMC free article: PMC5719131] [PubMed: 28983737] [CrossRef]
- 196.
- Inshaw JRJ, Cutler AJ, Crouch DJM, Wicker LS, Todd JA. Genetic variants predisposing most strongly to type 1 diabetes diagnosed under age 7 years lie near candidate genes that function in the immune system and in pancreatic beta-cells. Diabetes Care. 2020;43(1):169-177. doi:10.2337/dc19-0803 [PMC free article: PMC6925581] [PubMed: 31558544] [CrossRef]
- 197.
- Thomas NJ, Hill AV, Dayan CM, et al. Age of diagnosis does not alter the presentation or progression of robustly defined adult-onset type 1 diabetes. Diabetes Care. 2023;46(6):1156-1163. doi:10.2337/dc22-2159 [PMC free article: PMC7614569] [PubMed: 36802355] [CrossRef]
- 198.
- Mishra R, Chesi A, Cousminer DL, et al. Relative contribution of type 1 and type 2 diabetes loci to the genetic etiology of adult-onset, non-insulin-requiring autoimmune diabetes. BMC Med. 2017;15(1):88. doi:10.1186/s12916-017-0846-0 [PMC free article: PMC5404312] [PubMed: 28438156] [CrossRef]
- 199.
- Cousminer DL, Ahlqvist E, Mishra R, et al. First genome-wide association study of latent autoimmune diabetes in adults reveals novel insights linking immune and metabolic diabetes. Diabetes Care. 2018;41(11):2396-2403. doi:10.2337/dc18-1032 [PMC free article: PMC6196829] [PubMed: 30254083] [CrossRef]
- 200.
- Redondo MJ, Nathan BM, Jacobsen LM, et al. Index60 as an additional diagnostic criterion for type 1 diabetes. Diabetologia. 2021;64(4):836-844. doi:10.1007/s00125-020-05365-4 [PMC free article: PMC7940596] [PubMed: 33496819] [CrossRef]
- 201.
- Battaglia M, Ahmed S, Anderson MS, et al. Introducing the endotype concept to address the challenge of disease heterogeneity in type 1 diabetes. Diabetes Care. 2020;43(1):5-12. doi:10.2337/dc19-0880 [PMC free article: PMC6925574] [PubMed: 31753960] [CrossRef]
- 202.
- Cefalu WT, Andersen DK, Arreaza-Rubin G, et al. Heterogeneity of diabetes: beta-cells, phenotypes, and precision medicine: proceedings of an international symposium of the Canadian Institutes of Health Research’s Institute of Nutrition, Metabolism and Diabetes and the U.S. National Institutes of Health’s National Institute of Diabetes and Digestive and Kidney Diseases. Diabetes Care. 2022;45(1):3-22. doi:10.2337/dci21-0051 [PMC free article: PMC8753760] [PubMed: 34782355] [CrossRef]
- 203.
- Elding Larsson H, Vehik K, Bell R, et al. Reduced prevalence of diabetic ketoacidosis at diagnosis of type 1 diabetes in young children participating in longitudinal follow-up. Diabetes Care. 2011;34(11):2347-2352. doi:10.2337/dc11-1026 [PMC free article: PMC3198296] [PubMed: 21972409] [CrossRef]
- 204.
- Bingley PJ, Wherrett DK, Shultz A, Rafkin LE, Atkinson MA, Greenbaum CJ. Type 1 Diabetes TrialNet: a multifaceted approach to bringing disease-modifying therapy to clinical use in type 1 diabetes. Diabetes Care. 2018;41(4):653-661. doi:10.2337/dc17-0806 [PMC free article: PMC5860837] [PubMed: 29559451] [CrossRef]
- 205.
- Herold KC, Bundy BN, Long SA, et al. An anti-CD3 antibody, teplizumab, in relatives at risk for type 1 diabetes. N Engl J Med. 2019;381(7):603-613. doi:10.1056/NEJMoa1902226 [PMC free article: PMC6776880] [PubMed: 31180194] [CrossRef]
- 206.
- Bruining GJ, Molenaar JL, de Jongh BM, Aarsen RS, Visser HK. Prediction of type 1 diabetes mellitus—a report on three cases. Eur J Pediatr. 1985;143(3):175-178. doi:10.1007/BF00442131 [PubMed: 3987708] [CrossRef]
- 207.
- Tarn AC, Thomas JM, Dean BM, et al. Predicting insulin-dependent diabetes. Lancet. 1988;1(8590):845-850. doi:10.1016/s0140-6736(88)91601-7 [PubMed: 2895363] [CrossRef]
- 208.
- Ismail HM, Cuthbertson D, Gitelman SE, et al. The transition from a compensatory increase to a decrease in C-peptide during the progression to type 1 diabetes and its relation to risk. Diabetes Care. 2022;45(10):2264-2270. doi:10.2337/dc22-0167 [PMC free article: PMC9643141] [PubMed: 35998266] [CrossRef]
- 209.
- Ziegler AG, Rewers M, Simell O, et al. Seroconversion to multiple islet autoantibodies and risk of progression to diabetes in children. JAMA. 2013;309(23):2473-2479. doi:10.1001/jama.2013.6285 [PMC free article: PMC4878912] [PubMed: 23780460] [CrossRef]
- 210.
- Insel RA, Dunne JL, Atkinson MA, et al. Staging presymptomatic type 1 diabetes: a scientific statement of JDRF, the Endocrine Society, and the American Diabetes Association. Diabetes Care. 2015;38(10):1964-1974. doi:10.2337/dc15-1419 [PMC free article: PMC5321245] [PubMed: 26404926] [CrossRef]
- 211.
- Aly TA, Ide A, Humphrey K, et al. Genetic prediction of autoimmunity: initial oligogenic prediction of anti-islet autoimmunity amongst DR3/DR4-DQ8 relatives of patients with type 1A diabetes. J Autoimmun. 2005;25(Suppl):40-45. doi:10.1016/j.jaut.2005.09.002 [PubMed: 16242305] [CrossRef]
- 212.
- Ghalwash M, Dunne JL, Lundgren M, et al. Two-age islet-autoantibody screening for childhood type 1 diabetes: a prospective cohort study. Lancet Diabetes Endocrinol. 2022;10(8):589-596. doi:10.1016/S2213-8587(22)00141-3 [PMC free article: PMC10040253] [PubMed: 35803296] [CrossRef]
- 213.
- Stankov K, Benc D, Draskovic D. Genetic and epigenetic factors in etiology of diabetes mellitus type 1. Pediatrics. 2013;132(6):1112-1122. doi:10.1542/peds.2013-1652 [PubMed: 24190679] [CrossRef]
- 214.
- Levescot A, Malamut G, Cerf-Bensussan N. Immunopathogenesis and environmental triggers in coeliac disease. Gut. 2022;71(11):2337-2349. doi:10.1136/gutjnl-2021-326257 [PMC free article: PMC9554150] [PubMed: 35879049] [CrossRef]
- 215.
- Sollid LM, Lie BA. Celiac disease genetics: current concepts and practical applications. Clin Gastroenterol Hepatol. 2005;3(9):843-851. doi:10.1016/s1542-3565(05)00532-x [PubMed: 16234020] [CrossRef]
- 216.
- Dedmon LE. The genetics of rheumatoid arthritis. Rheumatology (Oxford). 2020;59(10):2661-2670. doi:10.1093/rheumatology/keaa232 [PubMed: 32638005] [CrossRef]
- 217.
- Tomer Y, Menconi F. Type 1 diabetes and autoimmune thyroiditis: the genetic connection. Thyroid. 2009;19(2):99-102. doi:10.1089/thy.2008.1565 [PMC free article: PMC2729687] [PubMed: 19191741] [CrossRef]
- 218.
- McKeigue PM, Spiliopoulou A, McGurnaghan S, et al. Persistent C-peptide secretion in type 1 diabetes and its relationship to the genetic architecture of diabetes. BMC Med. 2019;17(1):165. doi:10.1186/s12916-019-1392-8 [PMC free article: PMC6706940] [PubMed: 31438962] [CrossRef]
- 219.
- Leete P, Mallone R, Richardson SJ, Sosenko JM, Redondo MJ, Evans-Molina C. The effect of age on the progression and severity of type 1 diabetes: potential effects on disease mechanisms. Curr Diab Rep. 2018;18(11):115. doi:10.1007/s11892-018-1083-4 [PMC free article: PMC10043737] [PubMed: 30259209] [CrossRef]
- 220.
- McQueen RB, Geno Rasmussen C, Waugh K, et al. Cost and cost-effectiveness of large-scale screening for type 1 diabetes in Colorado. Diabetes Care. 2020;43(7):1496-1503. doi:10.2337/dc19-2003 [PMC free article: PMC7305000] [PubMed: 32327420] [CrossRef]
- 221.
- Redondo MJ, Oram RA, Steck AK. Genetic risk scores for type 1 diabetes prediction and diagnosis. Curr Diab Rep. 2017;17(12):129. doi:10.1007/s11892-017-0961-5 [PubMed: 29080981] [CrossRef]
- 222.
- Carr ALJ, Perry DJ, Lynam AL, et al. Histological validation of a type 1 diabetes clinical diagnostic model for classification of diabetes. Diabet Med. 2020;37(12):2160-2168. doi:10.1111/dme.14361 [PMC free article: PMC8086995] [PubMed: 32634859] [CrossRef]
- 223.
- Oram RA, Patel K, Hill A, et al. A type 1 diabetes genetic risk score can aid discrimination between type 1 and type 2 diabetes in young adults. Diabetes Care. 2016;39(3):337-344. doi:10.2337/dc15-1111 [PMC free article: PMC5642867] [PubMed: 26577414] [CrossRef]
- 224.
- Oram RA, Sharp SA, Pihoker C, et al. Utility of diabetes type-specific genetic risk scores for the classification of diabetes type among multiethnic youth. Diabetes Care. 2022;45(5):1124-1131. doi:10.2337/dc20-2872 [PMC free article: PMC9174964] [PubMed: 35312757] [CrossRef]
- 225.
- Grubb AL, McDonald TJ, Rutters F, et al. A type 1 diabetes genetic risk score can identify patients with GAD65 autoantibody-positive type 2 diabetes who rapidly progress to insulin therapy. Diabetes Care. 2019;42(2):208-214. doi:10.2337/dc18-0431 [PMC free article: PMC6828553] [PubMed: 30352895] [CrossRef]
- 226.
- Patel KA, Oram RA, Flanagan SE, et al. Type 1 diabetes genetic risk score: a novel tool to discriminate monogenic and type 1 diabetes. Diabetes. 2016;65(7):2094-2099. doi:10.2337/db15-1690 [PMC free article: PMC4920219] [PubMed: 27207547] [CrossRef]
- 227.
- Deutsch AJ, Stalbow L, Majarian TD, et al. Polygenic scores help reduce racial disparities in predictive accuracy of automated type 1 diabetes classification algorithms. Diabetes Care. 2023;46(4):794-800. doi:10.2337/dc22-1833 [PMC free article: PMC10090893] [PubMed: 36745605] [CrossRef]
- 228.
- Bonifacio E, Beyerlein A, Hippich M, et al. Genetic scores to stratify risk of developing multiple islet autoantibodies and type 1 diabetes: a prospective study in children. PLoS Med. 2018;15(4):e1002548. doi:10.1371/journal.pmed.1002548 [PMC free article: PMC5882115] [PubMed: 29614081] [CrossRef]
- 229.
- Yamashita H, Awata T, Kawasaki E, et al. Analysis of the HLA and non-HLA susceptibility loci in Japanese type 1 diabetes. Diabetes Metab Res Rev. 2011;27(8):844-848. doi:10.1002/dmrr.1234 [PubMed: 22069271] [CrossRef]
- 230.
- Allis CD, Jenuwein T. The molecular hallmarks of epigenetic control. Nat Rev Genet. 2016;17(8):487-500. doi:10.1038/nrg.2016.59 [PubMed: 27346641] [CrossRef]
- 231.
- Greenberg MVC, Bourc’his D. The diverse roles of DNA methylation in mammalian development and disease. Nat Rev Mol Cell Biol. 2019;20(10):590-607. doi:10.1038/s41580-019-0159-6 [PubMed: 31399642] [CrossRef]
- 232.
- Bannister AJ, Kouzarides T. Regulation of chromatin by histone modifications. Cell Res. 2011;21(3):381-395. doi:10.1038/cr.2011.22 [PMC free article: PMC3193420] [PubMed: 21321607] [CrossRef]
- 233.
- Jerram ST, Dang MN, Leslie RD. The role of epigenetics in type 1 diabetes. Curr Diab Rep. 2017;17(10):89. doi:10.1007/s11892-017-0916-x [PMC free article: PMC5559569] [PubMed: 28815391] [CrossRef]
- 234.
- Rakyan VK, Beyan H, Down TA, et al. Identification of type 1 diabetes-associated DNA methylation variable positions that precede disease diagnosis. PLoS Genet. 2011;7(9):e1002300. doi:10.1371/journal.pgen.1002300 [PMC free article: PMC3183089] [PubMed: 21980303] [CrossRef]
- 235.
- Stefan M, Zhang W, Concepcion E, Yi Z, Tomer Y. DNA methylation profiles in type 1 diabetes twins point to strong epigenetic effects on etiology. J Autoimmun. 2014;50:33-37. doi:10.1016/j.jaut.2013.10.001 [PMC free article: PMC3995844] [PubMed: 24210274] [CrossRef]
- 236.
- Disanto G, Vcelakova J, Pakpoor J, et al. DNA methylation in monozygotic quadruplets affected by type 1 diabetes. Diabetologia. 2013;56(9):2093-2095. doi:10.1007/s00125-013-2972-3 [PubMed: 23793775] [CrossRef]
- 237.
- Elboudwarej E, Cole M, Briggs FB, et al. Hypomethylation within gene promoter regions and type 1 diabetes in discordant monozygotic twins. J Autoimmun. 2016;68:23-29. doi:10.1016/j.jaut.2015.12.003 [PMC free article: PMC4792657] [PubMed: 26782299] [CrossRef]
- 238.
- Paul DS, Teschendorff AE, Dang MA, et al. Increased DNA methylation variability in type 1 diabetes across three immune effector cell types. Nat Commun. 2016;7:13555. doi:10.1038/ncomms13555 [PMC free article: PMC5141286] [PubMed: 27898055] [CrossRef]
- 239.
- Starskaia I, Laajala E, Grönroos T, et al. Early DNA methylation changes in children developing beta cell autoimmunity at a young age. Diabetologia. 2022;65(5):844-860. doi:10.1007/s00125-022-05657-x [PMC free article: PMC8960578] [PubMed: 35142878] [CrossRef]
- 240.
- Johnson RK, Vanderlinden LA, Dong F, et al. Longitudinal DNA methylation differences precede type 1 diabetes. Sci Rep. 2020;10(1):3721. doi:10.1038/s41598-020-60758-0 [PMC free article: PMC7048736] [PubMed: 32111940] [CrossRef]
- 241.
- Fisher MM, Watkins RA, Blum J, et al. Elevations in circulating methylated and unmethylated preproinsulin DNA in new-onset type 1 diabetes. Diabetes. 2015;64(11):3867-3872. doi:10.2337/db15-0430 [PMC free article: PMC4613977] [PubMed: 26216854] [CrossRef]
- 242.
- Olsen JA, Kenna LA, Spelios MG, Hessner MJ, Akirav EM. Circulating differentially methylated amylin DNA as a biomarker of beta-cell loss in type 1 diabetes. PLoS One. 2016;11(4):e0152662. doi:10.1371/journal.pone.0152662 [PMC free article: PMC4844136] [PubMed: 27111653] [CrossRef]
- 243.
- Akirav EM, Lebastchi J, Galvan EM, et al. Detection of beta cell death in diabetes using differentially methylated circulating DNA. Proc Natl Acad Sci U S A. 2011;108(47):19018-19023. doi:10.1073/pnas.1111008108 [PMC free article: PMC3223447] [PubMed: 22074781] [CrossRef]
- 244.
- Miao F, Smith DD, Zhang L, Min A, Feng W, Natarajan R. Lymphocytes from patients with type 1 diabetes display a distinct profile of chromatin histone H3 lysine 9 dimethylation: an epigenetic study in diabetes. Diabetes. 2008;57(12):3189-3198. doi:10.2337/db08-0645 [PMC free article: PMC2584123] [PubMed: 18776137] [CrossRef]
- 245.
- Miao F, Chen Z, Zhang L, et al. Profiles of epigenetic histone post-translational modifications at type 1 diabetes susceptible genes. J Biol Chem. 2012;287(20):16335-16345. doi:10.1074/jbc.M111.330373 [PMC free article: PMC3351309] [PubMed: 22431725] [CrossRef]
- 246.
- Miao F, Gonzalo IG, Lanting L, Natarajan R. In vivo chromatin remodeling events leading to inflammatory gene transcription under diabetic conditions. J Biol Chem. 2004;279(17):18091-18097. doi:10.1074/jbc.M311786200 [PubMed: 14976218] [CrossRef]
- 247.
- Miao F, Wu X, Zhang L, Yuan YC, Riggs AD, Natarajan R. Genome-wide analysis of histone lysine methylation variations caused by diabetic conditions in human monocytes. J Biol Chem. 2007;282(18):13854-13863. doi:10.1074/jbc.M609446200 [PubMed: 17339327] [CrossRef]
- 248.
- Ye J, Stefan-Lifshitz M, Tomer Y. Genetic and environmental factors regulate the type 1 diabetes gene CTSH via differential DNA methylation. J Biol Chem. 2021;296:100774. doi:10.1016/j.jbc.2021.100774 [PMC free article: PMC8191311] [PubMed: 33992646] [CrossRef]
Drs. Redondo and Onengut-Gumuscu reported no conflicts of interest. Dr. Gaulton consults for Genentech and holds stock in Neurocrine Biosciences.
- Summary
- Introduction
- Major Histocompatibility Complex and Type 1 Diabetes Risk
- Non-Major Histocompatibility Complex Risk Loci
- Type 1 Diabetes Risk Loci in Diverse Populations
- From Variant to Function
- Genes and Initiation of Type 1 Diabetes
- Genetics and Prediction of Type 1 Diabetes Risk
- Epigenetics of Type 1 Diabetes
- Conclusions
- List of Abbreviations
- Funding
- Acknowledgment
- Article History
- References
- Review Genetics of Type 1 Diabetes.[Diabetes in America. 2018]Review Genetics of Type 1 Diabetes.Rich SS, Erlich H, Concannon P. Diabetes in America. 2018 Aug
- Association of MHC SNP genotype with susceptibility to type 1 diabetes: a modified survival approach.[Diabetes Obes Metab. 2009]Association of MHC SNP genotype with susceptibility to type 1 diabetes: a modified survival approach.McKinnon E, Morahan G, Nolan D, James I, Diabetes Genetics Consortium. Diabetes Obes Metab. 2009 Feb; 11 Suppl 1(Suppl 1):92-100.
- Type 1 diabetes: evidence for susceptibility loci from four genome-wide linkage scans in 1,435 multiplex families.[Diabetes. 2005]Type 1 diabetes: evidence for susceptibility loci from four genome-wide linkage scans in 1,435 multiplex families.Concannon P, Erlich HA, Julier C, Morahan G, Nerup J, Pociot F, Todd JA, Rich SS, Type 1 Diabetes Genetics Consortium. Diabetes. 2005 Oct; 54(10):2995-3001.
- Localization of type 1 diabetes susceptibility to the MHC class I genes HLA-B and HLA-A.[Nature. 2007]Localization of type 1 diabetes susceptibility to the MHC class I genes HLA-B and HLA-A.Nejentsev S, Howson JM, Walker NM, Szeszko J, Field SF, Stevens HE, Reynolds P, Hardy M, King E, Masters J, et al. Nature. 2007 Dec 6; 450(7171):887-92. Epub 2007 Nov 14.
- Review Genetics of type 1 diabetes.[Clin Chem. 2011]Review Genetics of type 1 diabetes.Steck AK, Rewers MJ. Clin Chem. 2011 Feb; 57(2):176-85. Epub 2011 Jan 4.
- Genetics of Type 1 Diabetes - Diabetes in AmericaGenetics of Type 1 Diabetes - Diabetes in America
Your browsing activity is empty.
Activity recording is turned off.
See more...