NCBI Bookshelf. A service of the National Library of Medicine, National Institutes of Health.
Ranji SR, Steinman MA, Shojania KG, et al. Closing the Quality Gap: A Critical Analysis of Quality Improvement Strategies (Vol. 4: Antibiotic Prescribing Behavior). Rockville (MD): Agency for Healthcare Research and Quality (US); 2006 Jan. (Technical Reviews, No. 9.4.)
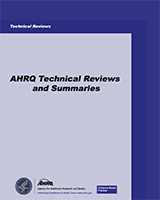
Closing the Quality Gap: A Critical Analysis of Quality Improvement Strategies (Vol. 4: Antibiotic Prescribing Behavior).
Show detailsScope
This report focuses on QI strategies to reduce unnecessary or inappropriate antibiotic treatment in the outpatient setting. Changes in outpatient antibiotic use are likely to have the greatest impact on efforts to arrest the development and spread of antibiotic-resistant bacteria that cause community-acquired infections. We restricted our focus to antibiotic treatment of acute illnesses, excluding studies of prophylactic antibiotic use and antibiotic therapy for chronic illnesses, both to minimize the conceptual heterogeneity of our approach and because chronic and prophylactic antibiotic use account for a small fraction of total outpatient antibiotic use.
As noted earlier, we reviewed strategies to affect antibiotic use in two realms: the antibiotic treatment decision, and the antibiotic selection decision. Studies in the “treatment decision” group addressed the dichotomous decision of whether or not to treat with antibiotics at all. These studies evaluated QI strategies to reduce the prescribing of unwarranted antibiotics, either overall or for a specific condition or patient population. Studies in the “antibiotic selection” group evaluated QI strategies to increase adherence to recommended choices of antibiotics in situations where antibiotic treatment is warranted.
Definitions of Quality Improvement Terms Used in This Report
For the purposes of this report, we used quality improvement terminology in accordance with the Institute of Medicine report described in previous volumes of the Closing the Quality Gap series 1 as follows:
- Quality gap: the difference between health care processes or outcomes observed in practice, and those potentially achievable on the basis of current professional knowledge. The difference must be attributable in whole or in part to a deficiency that could be addressed by the health care system.
- Quality improvement strategy: any intervention strategy aimed at reducing the quality gap for a group of patients representative of those seen in routine practice.
- Quality improvement target: the outcome, process or structure that the QI strategy targets, with the goal of reducing the quality gap. For this report, the main QI target was clinician prescribing behavior, either in terms of the decision to prescribe an antibiotic or the selection of an antibiotic.
Classification of Interventions
A single study may include different study arms (groups of subjects), and each arm may receive a different QI intervention. For the purposes of this report, each intervention type was abstracted separately and evaluated as a separate comparison. For example, a single study could contain three arms: a control or comparison group (depending on whether the study was an RCT or not), a group receiving clinician and patient education, and a group receiving clinician education only. We would consider such a study to contain two trials (two separate comparisons against the comparison group).
The intervention(s) used in a study sometimes included more than one QI strategy. Each type of QI strategy was abstracted separately, although considered part of the same intervention. Interventions containing two or more different QI strategies (as defined by the categorization listed below) were considered multifaceted interventions. For example, an intervention using (a) audit and feedback and (b) clinician education was defined as a multifaceted intervention, using two QI strategies. Finally, a study could contribute to both “treatment” and “selection” groups if the intervention clearly attempted to influence both the decision to prescribe and the selection of antibiotic, and reported these outcomes appropriately.
Classification of Quality Improvement Strategies
We classified QI strategies targeted at improving antibiotic prescribing in the following manner:
QUALITY IMPROVEMENT STRATEGIES 4 |
---|
Clinician education |
Interventions designed to increase understanding of clinical care principles, or awareness of specific practice recommendations. Specific subtypes of clinician education included passive strategies, where clinicians are not actively engaged in the learning process (e.g., distribution of educational materials, lectures, and meetings such as traditional CME); and active strategies, where clinicians interact and actively participate in their education in one-on-one or small group settings (e.g., workshops, consensus-building sessions, and educational outreach visits). In active strategies, the teacher can actively assess comprehension, and tailor information appropriately; whereas, in passive strategies there is little or no real-time monitoring of whether the information was received and understood by the learner. |
Patient education |
Interventions designed to promote increased understanding of target conditions or to teach specific prevention or treatment strategies. As with clinician education, patient education can also be subdivided into passive strategies (distribution of educational materials) and active strategies (one-on-one or group educational workshops). Mass media efforts (e.g., television announcements or billboards) are a type of passive education usually targeted at patients, but potentially exerting influence on clinicians as well. |
Provision of delayed prescriptions |
In this strategy, patients are given an antibiotic prescription, but instructed to fill the prescription only if their condition does not improve with supportive measures after a specified period of time. We considered delayed prescriptions to be a unique category. This strategy does not reduce antibiotic prescribing by the clinician per se , and it assumes that the “default” practice is to uniformly administer antibiotics (even when they might not be indicated, as in acute cough illness). Delayed prescriptions incorporate elements of patient self-management and patient incentives (e.g., guarantee of a “back-up” medication—antibiotics) to attempt to reduce actual antibiotic use. |
Audit and feedback |
Summary of clinical performance of health care delivery over a specified period of time, provided to the target clinicians by an individual or organization (e.g., the proportion of a clinician's patients with acute bronchitis for whom the clinician prescribed an antibiotic). Profiling, in which the performance of an individual, clinic or health system is compared with peers, and benchmarking, when the reference point is some established quality standard, would also be considered audit and feedback. |
Clinician reminders and decision support systems |
A paper-based or electronic system, intended to help a clinician incorporate specific information into a clinical decision. Decision support systems combine reminders with a prompt to follow a recommended pathway of clinical care. |
Financial and regulatory incentives or disincentives |
Inducements or disincentives that influence individuals to take a desired course of action. We considered incentives for patients and clinicians separately. Common types of financial incentives to patients to reduce unnecessary health care services include office-visit and prescription drug co-payments; capitated insurance plans are an example of financial incentives to clinicians to promote efficient care. A common regulatory disincentive targeted at clinicians is the requirement to submit extra documentation to receive approval to prescribe a restricted drug. |
- 4
Other commonly used QI strategies include patient reminders (efforts to improve adherence to self-care or keeping appointments), facilitated relay of clinical data to providers (provision of data outside of normal chart-based means), promotion of patient self-management (distribution of materials or access to a resource that enhances self-care), and organizational change (changes in the structure or delivery of care to improve efficiency or breadth of care). These strategies are commonly applied in chronic disease management, but are less applicable to an acute illness model, and were only featured in one included study. 87
Inclusion and Exclusion Criteria
The inclusion criteria were adapted from previous volumes of the Closing the Quality Gap series. 1, 2 Included studies were required to:
- Evaluate an intervention incorporating one of the QI strategies defined above to improve the quality of outpatient antibiotic prescribing for acute illnesses in the outpatient setting (clinic, urgent care, or emergency department).
- Use either (1) an experimental design with a comparison group assigned by the investigators, including patient- or cluster-randomized controlled trials (RCT), quasi-RCT 5 design, and controlled before-after (CBA) 6 studies; or (2) when a comparison group was not employed, use of an interrupted time series (ITS) with a clearly defined intervention time period, and at least three measurements before and after the intervention.
- Report at least one measure of antimicrobial agent use.
Trials that reported outcomes related to antimicrobial use, such as antimicrobial resistance rates, prescribing costs, health services utilization, or satisfaction with care, were included only if they also measured antimicrobial prescribing. For example, a study of antimicrobial costs to a health care system would be excluded if costs were the only outcome measured; however, if the study also measured antimicrobial prescribing (and met the other methodologic criteria above) the study would be included. We also included studies that assessed the effect of QI strategies on the dose or duration of antimicrobial therapy, where these outcomes were targets of the intervention.
We anticipated that some interventions would give rise to multiple publications. In these situations, we regarded the first publication as the primary study, but included additional information on the intervention or results from the other publications as warranted. In cases where results of an intervention were described at multiple time points in multiple studies, we abstracted data from the short-term followup article, judging that longer-term followup might address the sustainability of an intervention rather than its effectiveness, and in an attempt to maintain consistency of intervention time periods to maximize our ability to pool results across studies.
Literature Search and Review Process
To identify studies for potential inclusion, we searched the electronic database of the Cochrane Registry Effective Practice and Organisation of Care (EPOC) group ( Appendix C * ). The EPOC database catalogs studies that attempt to “improve professional practice and the delivery of effective health care services.” The database includes the results of extensive periodic searches of MEDLINE® (from 1966-present), EMBASE (1980-present), and CINAHL® (1982-present), as well as hand searches of specific journals and article bibliographies. 88 The strategy for identification of appropriate studies has a sensitivity of 92.4%. 88 The main EPOC registry primarily includes studies of clinician and system-targeted interventions, identified from a larger database of quality-improvement related studies compiled by Cochrane search experts. To maximize our search yield, we searched the main registry as well as the larger database of QI articles identified for possible inclusion but not meeting criteria for entry in the main registry. We searched for studies relating to antimicrobial prescribing, using terms specific to antimicrobials and prescribing practices as well as specific target conditions (e.g., pharyngitis, urinary tract infection) ( Appendix C ). We searched the EPOC registry through October 2004. To identify more recent studies, we performed a targeted search of MEDLINE® from June 2004 to November 2004 using similar search terms ( Appendix C ). In addition, we hand-searched the reference lists of each article that met all inclusion criteria. We included only English-language studies.
A trained research assistant and a physician-investigator (SRR) screened titles and abstracts of the retrieved citations for relevance. If reviewers could not make a decision based on the title and abstract alone, the article automatically moved to full-text review. All full-text reviews were performed by a trained research assistant and at least one core investigator (SRR, MAS, RG). In that stage, reviewers abstracted detailed information on study characteristics, study design, measures of study quality, and outcomes, which were recorded on standardized abstraction forms ( Appendix D * ). Disagreements on the abstracted fields were resolved by consensus, occasionally involving discussion with another core investigator.
Outcome Measures
For studies evaluating the antibiotic treatment decision, the principal outcome measure was the proportion of office visits in which a clinician prescribed an antibiotic. For studies evaluating the antibiotic selection decision, the primary outcome measures were proportion or volume of total antibiotic prescriptions written for a recommended antibiotic, or written in compliance with a specific guideline. Other forms of antibiotic utilization outcomes (e.g., antimicrobial prescriptions per person-year, antimicrobial prescriptions per clinician-year) were abstracted as well, including studies that did not report data in the form of our primary outcome measures.
Secondary outcome measures (in articles that also reported a measurement of antimicrobial prescribing) included clinical and health system effects of antibiotic prescribing, including intervention effects on adverse drug events, clinical outcomes, return visits or illness-related hospitalizations, antimicrobial resistance, costs of prescribing, patient satisfaction, and duration of office visits.
Assessment of Study Quality
In order to assess the overall quality of the literature, we assessed studies based on key elements that increase internal validity and translatability. However, once studies met our inclusion criteria, we did not exclude any on the basis of study quality, nor did we weight statistical analyses by study quality, as this process may unduly influence the results of meta-analysis. 89 Study factors that influence internal validity were chosen based on the methodology of the Cochrane Collaboration, and factors influencing translatability were chosen based on prior literature in the field. They were:
- Factors affecting study internal validity
- Method of treatment assignment
- Were study subjects randomized, and if so, was the randomization process described?
- For non-randomized studies, was the rationale for selection of the comparison group explained, and a baseline observation period included (to assess selection bias)?
- Blinding
- Were the outcome assessors blinded to treatment group assignment?
- Statistical analyses
- Was a unit-of-analysis error present? If so, were appropriate statistical methods used for correction?
- Factors affecting study translatability
- Study design
- Did the study document a quality gap in antibiotic prescribing in the study population?
- Did the study address prescribing for a specific condition (as opposed to prescribing in general)?
- Did the comparison group receive usual care (no intervention) or a low-intensity intervention?
- Did the study measure prescribing by chart review, or through use of administrative claims data? (Chart review is a more accurate reflection of the clinician's prescribing patterns, since administrative claims data captures only when a patient fills a prescription. Prior studies suggest that about 10 – 15% of antibiotic prescriptions identified on chart review are missed on searches of administrative claims. 90 )
An Explanatory Model for Evaluating the Different QI Strategies To Improve Antibiotic Prescribing Behavior
A comparison of effects for QI strategies with different targets must also take into account the possibility that contextual factors, such as intervention delivery, setting, and population factors, could confound (or be responsible for) the observed associations between QI strategies and behavior change. We have constructed the following model for guiding evaluation of QI strategies in light of these possible confounding and moderating factors ( Figure 2 ). Some of these factors may bias the results of an individual study—for example, sample size or the type of intervention delivered to the comparison group. They may also act as effect modifiers or confounders of the observed association between a QI strategy and median effect size. We also examined certain intervention and population factors, which are not typically considered “confounders” at the individual study level (e.g., study design), but which could influence the overall association between QI strategy and median effect size at the summary level.

Figure
Figure 2. Evaluation of QI strategies for confounders and moderators.
Statistical Analysis
We expected that the identified studies would exhibit significant heterogeneity, due to variations in study populations (e.g., by age or condition), methodologic features, and characteristics of the interventions (e.g., intensity) or the context in which they were delivered (e.g., magnitude of quality problem, alignment with attitudes of patients and clinicians). Our conventional random-effects meta-analysis confirms the existence of marked heterogeneity, which persisted even after stratifying studies by various design and intervention features ( Appendix E * ).
Therefore, as our primary approach to the analysis, we chose a framework based on the median effect size, comparing groups of studies that shared specific features of interest. This approach was first developed in a large review of strategies to foster the implementation of clinical practice guidelines 91 and subsequently applied to the reviews of QI strategies for diabetes and hypertension care in previous volumes in this series. 1, 2
To calculate the median effect size, we calculated the net effect size for each study reporting dichotomous outcomes by subtracting the difference between post-intervention and pre-intervention rates in the comparison group from the difference between post-intervention and pre-intervention rates in the intervention group. Thus, in the treatment group, negative effect sizes indicate a reduction in antibiotic prescribing (lower rate of prescribing in the intervention group, post-intervention); in the selection group, positive effect sizes indicate an increase in prescribing of a recommended antibiotic in the intervention arm compared with the comparison arm.
The median effect for a group of studies is simply the median of the individual effect sizes of individual studies. Using the median effect approach allows preservation of the natural study units, and allows comparisons across different methodologic features or effect modifiers (e.g., baseline adherence).
In order to minimize multiple comparisons and spurious associations, we followed a structured approach to the evaluation of each research question based on our model above. In the first step, we assessed whether the crude median effect size varied between different QI strategies using non-parametric rank-sum tests (Wilcoxon or Kruskal-Wallis, as appropriate). We next sought to determine if other study characteristics could potentially act as effect modifiers or confounders of the observed relationship between QI strategy and median effect size. To do this, we established two criteria that had to be met for a variable to be considered a confounder or effect modifier: (1) the distribution of QI studies differed across strata of the study characteristic, and (2) the median effect size of studies varied across the study characteristic. For the first step, we analyzed contingency tables comparing the distribution of the number of studies for each QI strategy across dichotomized levels of each study factor (study factors were dichotomized due to the limited number of studies in our sample). The variables analyzed in this fashion included those we used to assess study translatability, along with other intervention characteristics and study population factors. For this purpose, each group of studies (treatment and selection), was examined for the following factors: country of study (US vs. other), effective sample size (above vs. below median sample size), baseline prescription or compliance rate (above vs. below median prescribing rate), intervention characteristics (repeated vs. one-time intervention, multifaceted vs. single QI strategy, use of passive vs. active QI strategies), type of comparison group (no intervention vs. low-intensity intervention), and target populations (specific disease target vs. no disease target; children targeted vs. children not targeted). To preserve power, we only included QI strategies represented by more than three interventions. A p-value of < 0.20 (by Fisher's exact test) was used as the threshold to consider the variable a potential effect modifier or confounder. We chose this liberal threshold to reduce the chance of missing potential confounders simply due to relatively small numbers of studies. When a factor was identified as a potential effect modifier or confounder through its association with the QI strategy, for the second step we then tested whether the factor was associated with the outcome (median effect size) using the non-parametric rank-sum tests noted above.
In addition to this analysis, we also performed stratified analyses to assess for differences in median effect sizes based on presence or absence of our prespecified quality criteria for internal validity, as these factors could bias individual study results. All statistical analyses were performed using STATA version 8.2 statistical software (Stata Corp., College Station, TX).
Calculation of Effective Sample Sizes
Cluster randomized trials, in which treatment allocation occurs at the level of clinicians or groups (e.g., randomization by clinic), are an increasingly encountered form of evaluation in QI research. 92 Allocating treatment in this manner is sometimes done out of convenience, but sometimes reflects the investigator's desire to avoid contamination. If treatment were assigned randomly at the patient level, clinicians would have some patients in each group and might therefore change their behavior for control and intervention patients. 93, 94 In cluster-randomized trials, some of the care that patients within each group receive is similar, and thus the two groups cannot be considered truly independent. Correction for this non-independence results in a smaller effective patient sample size for each study. 95 – 99 The effective sample size N is equal to: N Effective = (k*m) / (1 + (m-1)*ICC) where ‘k’; represents the number of clusters, ‘m’ denotes the number of observations per cluster, and ‘ICC’ equals the intra-cluster correlation coefficient. We used ICCs calculated from an existing database of prescriptions and clinician characteristics collected as part of the Minimizing Antibiotic Resistance in Colorado Project (AHRQ R01 HS13001). This database consists of all office visits and antibiotic prescription claims related to ARIs in Denver and Colorado Springs Metropolitan Statistical Areas from four large managed care organizations. This analysis showed an ICC of 0.055 for clustering at the clinician level, and 0.033 for clustering at the clinic level. The effective sample sizes calculated in this fashion were used for stratified analyses, as described above. These numbers fall within the range of values for ICCs found in quality improvement trials in primary care settings. 98
Calculation of the Population Effect Size
Because certain patient populations and conditions are much more common than others, the median effects approach does not identify which interventions would have the greatest effect on total antibiotic use at the population level. Thus, we took a payor and public health perspective in standardizing changes in antibiotic prescriptions to the community level. An additional advantage of this approach is that it also allows inclusion of studies that were not eligible for the median effects analysis due to differences in how outcomes were measured. In order to standardize the observed median effects at the community level, we used data from the 2002 National Ambulatory Medical Care Survey (NAMCS) 100 ( Figure 3 ) to extrapolate the relative reductions in antibiotic prescribing for specific conditions and age groups to absolute reductions in antibiotic prescribing in the general population, expressing results across studies as antibiotic prescriptions saved per 1000 person-years. This model assumes that the observed effect in each study lasted for 1 year. Using US Census estimates and NAMCS for 2002, we estimated that the US population of 283.1 million persons yielded 83.17 million ARI office visits in 2002. When interventions did not target a specific condition or age group, we multiplied total number of ARI office visits by the absolute net change in proportion of visits at which antibiotics were prescribed as a result of the intervention, then divided by the US population to estimate antibiotics saved per person-year. When studies focused on specific conditions and age groups, we used data from NAMCS to estimate the number of condition-specific office visits for the relevant age group(s), and divided by 283.1 million to standardize this effect to the general population. For studies that expressed results as antibiotic prescriptions per person-year, we multiplied the absolute change in prescriptions per person-year by the number of (condition-specific) office visits to arrive at the population effect.
Table
Figure 3. Ambulatory antibiotic prescriptions for various ARIs, by age group.
To estimate the cost of antibiotic prescriptions, we used the average wholesale price for a 7-day supply (except for azithromycin, which was 5 days) published by Red Book in January 2002. Because we did not have data on specific antibiotic prescriptions, we calculated the weighted average cost for a single prescription within each antibiotic class based on the distribution of specific antibiotic prescriptions within each class derived from the National Ambulatory Medical Care Survey in 2002. Based on this methodology, the weighted average costs for a single prescription within each antibiotic class in 2002 was the following: tetracyclines ($5.24), cephalosporins ($46.94), macrolides ($41.91), penicillins ($28.36), quinolones ($52.97), other ($37.18). These costs do not include the cost of dispensing the prescription.
Particularly Salient Studies
Although the median effects analysis and systematic review outlined above provides the most comprehensive summary of the body of evidence, we recognize the limitations of such analyses in providing concrete examples for stakeholders. We have thus identified three particularly salient studies that illustrate the following three virtues: first, studies that met a high number of our prespecified quality criteria; second, studies that would be easily translatable to other settings; third, studies incorporating representative types of quality improvement strategies. The final studies chosen for highlighting were identified by discussion among the core investigators. These studies are summarized in Appendix A * .
Footnotes
- 5
“Quasi-RCT” refers to studies described as randomized where randomization was altered by investigators, or where a method of patient assignment was used that is not truly random (e.g. assignment by even/odd medical record number or date of clinic visit.)
- 6
Controlled before-after studies are non-randomized trials with a contemporaneous comparison group.
* Appendixes cited in this report are provided electronically at http://www
.ahrq .gov/downloads/pub /evidence/pdf/medigap/medigap.pdf
- Scope
- Definitions of Quality Improvement Terms Used in This Report
- Classification of Interventions
- Classification of Quality Improvement Strategies
- Inclusion and Exclusion Criteria
- Literature Search and Review Process
- Outcome Measures
- Assessment of Study Quality
- An Explanatory Model for Evaluating the Different QI Strategies To Improve Antibiotic Prescribing Behavior
- Statistical Analysis
- Calculation of Effective Sample Sizes
- Calculation of the Population Effect Size
- Particularly Salient Studies
- Methods - Closing the Quality Gap: A Critical Analysis of Quality Improvement St...Methods - Closing the Quality Gap: A Critical Analysis of Quality Improvement Strategies (Vol. 4: Antibiotic Prescribing Behavior)
Your browsing activity is empty.
Activity recording is turned off.
See more...