This book is distributed under the terms of the Creative Commons Attribution-NonCommercial-NoDerivs License which permits noncommercial use and distribution provided the original author(s) and source are credited. (See https://creativecommons.org/licenses/by-nc-nd/4.0/
NCBI Bookshelf. A service of the National Library of Medicine, National Institutes of Health.
Structured Abstract
Background:
All data sets are flawed. Values may be missing where they are expected to be present, be present but contain values that do not represent reality, or be inconsistent compared with other values (eg, sex = male; diagnosis = pregnant). Currently, no standard practices and metrics exits to describe data quality (DQ). As a result, current DQ processes are ad hoc and nontransparent to data users and consumers.
Objectives:
The goal of this project was to address the question, What aspects of DQ help users (eg, health researchers, patient advocates, and policy makers) and consumers (eg, patients and policy makers) have confidence in the results that are generated from a data set? This project focused on creating an agreed-on set of terminology definitions and recommendations to guide assessment and reporting of DQ findings.
Specific Aims
- To develop community-driven recommendations for DQ measurement and reporting
- To define a DQ common data model (CDM) for storing DQ measures and to assess its viability within several large comparative effectiveness research (CER) data networks
- To create prototype DQ reports and visualizations that present results in an intuitive, informative, and understandable format to multiple patient-centered outcomes research (PCOR) stakeholders.
- To understand technical, professional, and policy barriers to increased DQ transparency
Methods:
For specific aims 1 and 4, four stakeholder all-day face-to-face meetings plus monthly webinars and more than 10 national presentations allowed for continuous project engagement and assessment. We created 2 stakeholder communities: (1) patients, patient advocates, and health care policy makers; and (2) data stewards, informatics professionals, and clinical investigators. For specific aims 2 and 3, a 2.5-day in-person DQ Code-A-Thon brought together programmers and data users to create DQ visualization prototypes, which we used during the second set of stakeholder meetings.
Results:
Four separate publications capture the conclusions produced by the community (Section I): (1) a harmonized terminology for describing DQ dimensions, (2) recommendations for reporting DQ results, (3) an evaluation of more than 11 000 DQ checks from 8 networks, and (4) a survey exploring professional and organizational barriers to performing DQ assessment and reporting results. In addition, we created multiple DQ visualizations and a technical specification for a CDM for storing DQ results. All publications and technical materials are freely available through links on the Data Quality Collaborative website (http://repository.edm-forum.org/dqc/). [link inactive]
Conclusions:
Data quality assessment remains a complex set of concepts, activities, computations, and reporting methods. A harmonized terminology unifies these disparate threads; a CDM for DQ measures brings technical computations under a single representation. Yet, the challenge of creating intuitive visualizations and easily interpreted reporting structures for technical and nontechnical stakeholders remains unsolved.
Limitations:
This work focused on general measures of DQ (“intrinsic DQ”). Yet most data users are interested in the fitness of a subset of elements needed for a specific study. The next phase of this work must include “fitness-for-use” DQ assessment and reporting methods.
Background
Patient-centered outcomes research is being enhanced by explosive growth in the diversity and depth of patient-specific electronic data sources. Electronic health records (EHRs), personal health records, internet blog postings, social media sites, and wearable electronic sensors are examples of new sources that provide a more intimate and complete view of an individual's personal experiences with his or her health and the health care system.1-4 Further, advances in the operation and function of disease-monitoring sensors, such as glucose monitors that automatically upload an individual's blood glucose levels to his or her doctor's office, have expanded patient interest in these data. While large administrative claims databases have long been used for retrospective observational studies, limitations have led to heightened interest in other electronic sources, such as EHRs.5-7 Studies using EHR data have enabled investigators to examine the impact of diagnostic and therapeutic interventions in diverse real-world clinical settings.2,8-12
Comparative effectiveness studies, patient-centered outcomes research, and pragmatic trials using EHR data captured during routine clinical care from 1 or more practice settings are becoming important complements to prospective randomized trials for generating new insights and knowledge. National and international EHR-based clinical research networks are expanding the scope and depth of real-world data to answer critical questions about care decisions and outcomes important to patients and families.13-20
Detailed clinical data are generated mostly by electronic transactions in operational systems that are not primarily intended for research and secondary analysis. Secondary data use refers to the use of data for purposes other than those for which the data were originally collected. Examples include operational performance dashboards, quality improvement measures, and research analytics. Access to large quantities of clinical data from operational EHRs holds much promise.
However, a major concern is that data not collected systematically for research will be of poorer quality, which could negatively impact findings generated from these data and hamper patient-driven interest in access to and use of their health data. Transaction-oriented systems rarely include prespecified, unambiguous data definitions or uniform (unbiased) data collection procedures. A substantial body of research suggests that data collected in EHRs and other operational systems may not be of sufficient quality for research.21-30 EHRs typically are optimized for efficient patient care and nonclinical administrative requirements, resulting in great variation in clinical documentation practices even among users of the same system.31-33
Legitimate concerns about the DQ from these sources and the impact on study validity need to be addressed. Current data quality assessment (DQA) and data “cleaning” methods are ad hoc, rarely reported in publications, and, if reported, not described in a uniform manner that can be easily understood by patients, providers, policy makers, or researchers. As clinical warehouses and large-scale EHR-based networks become established repositories of electronic health data, consistent methods for describing, assessing, and reporting DQ findings could be a way to help secondary data users and consumers understand the potential impact of DQ on reusing data and interpreting findings.29,34
Exposing the strengths and weaknesses of a data set enables a scientist to determine if these flaws impact the intended use. Flaws in a data set may make it unusable for 1 use case but acceptable for a different use case. Making DQA results easily accessible to all users (including patient advocacy groups and policy makers) increases transparency and trust in the findings derived from the data and facilitates the opportunity for patients, their representatives, and policy makers to be actively engaged in defining what is studied and how. Without facilitating access to large secondary data sets by stakeholders outside of the owners of the data, opportunities to obtain maximum benefit and insights stemming from these data may be reduced. Thus, having direct access to well-described DQ measures is critical for ensuring data are of sufficient quality to proceed with any type of analysis. The Equator Network35,36 is an initiative in place to promote transparent and accurate health research reporting to enhance the reliability of medical research literature. Currently, there are no DQ reporting guidelines consistent with these goals to ensure DQ transparency for data users (investigators) and results consumers (patients, providers, policy makers).
The overall goal of this project was to address the following question: What aspects of DQ help data users (health researchers) and data consumers (patients, patient advocates, and policy makers) have confidence in the results that are generated by a data set? No standard practices or metrics currently exist to describe DQ findings and, as a result, the current process is not transparent to users and consumers. This project focused on creating a consensus set of terminology definitions and recommendations to guide DQA, presentation of results, and visualization of those results in understandable formats through 4 specific aims:
- To develop community-driven recommendations for DQ measurement and reporting
- –
This engagement-focused aim matters to patients and policy makers because it will help improve understanding and facilitate use of secondary data by entities beyond the owners of the data, which affords the potential for unlimited uses of these data.
- a.
To define a DQ common data model (DQA-CDM) for storing DQ measures and to assess its viability within several CER data systems
- –
This technical-focused aim is a key first step to sharing and reusing DQ tools built by existing DQ teams to reduce barriers to performing DQA and to increase comparability of DQ findings.
- b.
To create prototype DQ reports and visualizations that present DQ results in an intuitive, informative, and understandable format to multiple PCOR stakeholders
- –
This technical-focused aim seeks to facilitate understanding of DQ findings by nonscientists and, in turn, promote the usability of secondary data sets.
- c.
To understand technical, professional, and policy barriers to increased DQ transparency
- –
This engagement-focused aim seeks to identify barriers that may inhibit opportunities for broader reporting of DQ findings by all data owners.
Participation of Patients and Other Stakeholders
Types and Number of Stakeholders Involved
We engaged stakeholders in various activities that included 2 face-to-face stakeholder engagement meetings to review DQ reporting recommendations, reports, visualizations and barriers to DQ reporting (N = 92 stakeholders); online participation in feedback on a public website/wiki about the recommendations developed at the face-to-face meetings (N = 138 comments); a survey exploring professional and organizational barriers to performing DQ assessment and reporting results (N = 141 respondents); and a DQ Code-A-Thon designed to develop visualizations that could present complex DQ results in a consumable format for data users and PCOR stakeholders (N = 20 participants). Participants were patient advocates, policy makers, data project managers, data analysts and programmers, comparative effectiveness researchers, and technical professionals who manage and analyze large data sets or are otherwise interested in DQ methods and standards.
How the Balance of Stakeholder Perspectives Was Conceived and Achieved
Our study engaged a wide range of stakeholders across the PCOR community to obtain balanced perspectives on the critical issues of defining, measuring, and reporting DQ across diverse sources. We assembled a broad community of PCOR consumers. One important way this balance was achieved was through the recruitment method. Lead team members leveraged connections across educational, community, governmental, and public institutions to reach and recruit a varied group of community members interested and involved in DQ issues. A second way we achieved a balanced perspective was by holding face-to-face meetings among groups of stakeholders. A patient advocate and policy maker group and a data analyst and technical professional group met on 2 separate days. This allowed us to engage each group during its own all-day meeting to ensure thorough data collection on both sets of perspectives.
Additionally, our DQ Code-A-Thon brought together coders, visualization experts, and data managers who formed interdisciplinary teams to develop prototype DQ reports and visualizations, which facilitated balanced engagement across various types of stakeholders. Finally, because we partnered with the Agency for Healthcare Research and Quality–funded Evidence, Data, & Methods (EDM) Forum through its network of data-interested professionals, we were able to post project products on the Forum's wiki page to highlight outcomes from the community stakeholder engagement meetings. The wiki facilitated outreach to an even broader community of DQ-interested people.
Methods Used to Identify and Recruit Stakeholder Partners
The project investigator (PI) and our lead community partner collaborator both lead groups focused on the DQ of large administrative data sets. They also are engaged with multiple individuals from many educational, community, governmental, and public institutions in health data and DQ content areas. Additionally, the PI engaged the team leads at numerous influential data warehouses within health care, the pharmaceutical industry, and educational institutions, as well as lead individuals within private industry both nationally and internationally. We also recruited through working groups formed to generate analyses and monitor data to promote better health care decisions, including Observational Health Data Sciences and Informatics (OHDSI), PCORnet, and Sentinel, which are the leading examples of large-scale national data sharing networks with heterogeneous data partners. Through these contacts, we approached the leads and asked for referrals of individuals we could recruit to participate in our DQ Code-A-Thon and other study activities. This “snowball” recruitment method successfully exceeded the a priori target recruitment goals.
Methods, Modes, and Intensity of Engagement
Our study employed multiple modes of engagement that varied in intensity. Specifically, the DQ Code-A-Thon required the most intense engagement, followed by the face-to-face meetings, survey participation, and e-community engagement. Methods that worked particularly well for engaging with patients and other stakeholders included presenting a problem to be solved, or presenting an interactive product (such as the DQ visualizations) for review and discussion. Additionally, approaching engagement exercises by asking stakeholders to assess how issues or products would impact their own organizations or jobs was an effective way to increase discussion and drive recommendations and the generation of solutions. This worked well because it made information personally salient to stakeholders; they could visualize and think about how issues or products could impact their own lives, and thus could generate more specific suggestions. In fact, it was through our community stakeholder engagements at the face-to-face meetings that a large amount of constructive information flowed from our stakeholders to the study team, which in turn modified project products (DQ concepts, terms, and visualizations). Although our online survey and e-community engagement activities were less intensive, these methods were effective in garnering feedback about study progress, as well as important information about barriers to DQ reporting, that could be summarized and reported at subsequent meetings as well as shared with the larger research community via web-based teleconferences and manuscripts.
Perceived or Measured Impact of Engagement on Study Relevance, Processes and Quality, Transparency, and Adoption of Findings
Engagement in our study impacted the relevance of the research question, study quality, and usefulness and uptake of findings. After our first face-to-face meeting in July 2014, stakeholders expressed a strong interest in DQA and reporting, demonstrating the strong relevance of our study. They reported that they desired significant engagement at initial data collection and analysis stages as well as at later-stage categorizing, analyzing, and reporting of DQA findings. Patient advocacy stakeholders believed that engagement at data point of capture is critical to improved accuracy and clinical decision making. Policymakers identified medical providers' distrust of accuracy in EHRs as a significant barrier to secondary use of data sets (for CER, benchmarking, meaningful use). All stakeholders believed they have unique and important roles in promoting the implementation and dissemination of DQA results.
Our monthly DQ webinars among patient advocates, policy makers, and CER researchers have had positive impacts insofar as they have provided discussion about the study progress and quality, as well as idea sharing that applies to the current project's deliverables.
For example, 1 meeting highlighted another researcher's early-stage metadata tool currently being built to support a multi-institutional data network. This topic generated in-depth discussion and ideas about current and future projects' study quality and approach.
An important topic covered at the second set of stakeholder engagement meetings in June 2016 that demonstrates the impact of engagement on our study's adoption and usefulness was how stakeholders thought they could be change agents for DQA and reporting to their own communities, and how they thought the current project could support communication of DQA and visualization standards through community-appropriate communication channels (a manuscript presenting these findings is in preparation). Discussion among stakeholders produced several recommendations on how they could envision themselves as change agents and what resources would be needed, including (1) access to a compilation of examples of what kinds of DQ issues exist and how they typically arise, (2) a set of talking points for use in their own organizations/communities, (3) a better understanding of the business side/staffing hours necessary for implementation of DQ checks, and (4) access to a DQA tool that is championed and maintained by a supporting entity. The most positive impact of these discussions and engagement was the formation of a reciprocal relationship between the project team and our stakeholders, and the resulting identification of the strengths and weaknesses of the project's overall deliverables.
One way our study facilitated transparency was our DQ Code-A-Thon, which engaged key stakeholders that will ultimately use the primary outcome of this project: an industry standard for DQA and reporting. Through the detailed review and application of a nascent CDM for storing DQ measures (DQA-CDM), the coders, visualization experts, and data managers at the DQ Code-A-Thon provided real-world testing of DQAs and identified limitations in Version 1 of the DQA-CDM. One of the most notable impacts of this process was the improvement in the DQA-CDM that resulted from stakeholders' pilot-testing the CDM and identifying needed changes and feedback. Additionally, in support of transparency and to stimulate interest in replication of this work by other health researchers, we presented results from the first set of stakeholder engagement meetings to public health and comparative effectiveness researchers in a session focused on the role of “big data” and health care analytics at the 2015 national meeting of the American Public Health Association in Chicago.
Engagement with stakeholders during the second face-to-face meeting included a discussion about the results of a survey of professional and organizational barriers to performing DQA and reporting DQ results. The face-to-face discussions both confirmed and advanced interpretation of these findings and the impact of DQA on perceptions of data quality and the credibility of study findings, which were included in a final published manuscript.
Methods
Study Design
The core methodology we used to develop the project work products was based on continuous community engagement with 4 face-to-face workshops—2 workshops in the first and the last project years as described in detail in Section D. Figure 1 shows 2 timelines of events from 2 of the publications (details in Findings 1.1 and 3.1 below) from the current project. These timelines illustrate how workshops and webinars/conference calls (as community engagement activities) formed the key study design to elucidate the core findings for DQ terminology harmonization, DQA measures standardization, barriers to use of DQAs, and DQA findings reporting and visualizations.
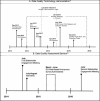
Figure 1
Study Designs for DQ Work Products 1.1 and 2.2. Key Activities Involved Continuous Stakeholder Engagement With 4 Stakeholder Engagement Sessions—2 in July 2014 and 2 in June 2016.
Three work products included additional methods; we solicited participants as described in Section D:
- The investigation of individual and organizational barriers to performing DQA and reporting findings (Figure 1B) for aim 4 included an anonymous online survey.
- The development and initial testing of a CDM for storing DQA findings (DQA-CDM) for aims 2 and 3 created a HIPAA-compliant deidentified database, and included executing a 2.5-day in-person DQ Code-A-Thon held in Denver, Colorado, in November 2015.
- The evaluation of more than 11 000 existing DQ checks acquired from 6 existing data networks against the harmonized DQ terminology for validating aim 1. The 6 networks were a convenience sample suggested by the DQ community participants.
Study Cohort
Section D provides details on how we identified, selected, and engaged participants for this community-based project. We identified participants as members of the “patient, patient advocate, policy maker” or “informatics, statistics, investigator” community. The study team members were facilitators in interactions with both communities. Study team members were participants in the development of the DQ harmonized terminology and reporting recommendations (aim 1) and the DQ Code-A-Thon (aim 3).
Study Settings
We used multiple environments to solicit community input and engagement. Where appropriate, we chose an online or webinar format as a matter of convenience to participants and also to encourage response and engagement among a broad base. We selected meeting sites based on meeting host resources and nearness to potential participants:
- The core mechanism was a weekly, then monthly, online webinar focused on key project topics—initially DQ terminology harmonization and reporting, input on the DQA-CDM, and input on draft versions of the DQ barriers survey. The webinar was open to the DQ community, including earlier participants in the EDM Forum Data Quality Collaborative. We encouraged additional participation in all public presentations. Over time, the participation list for the webinar has grown to more than 25 individuals.
- EDM Forum hosted 4 all-day face-to-face meetings in Washington, DC. The project paid all travel expenses for participants.
- A 2.5-day in-person DQ Code-A-Thon event held in Denver engaged end-users, technical programmers, and data visualization experts. The project paid all travel expenses for participants.
- An anonymous online survey solicited barriers to performing and reporting DQA results.
Data Collection
All project work products were created with community consensus. We reproduce here the detailed methods section from the 4 publications. Since we used workshops and webinars to create multiple work products, there is redundancy in the descriptions of these methods across publications.
1. To create the harmonized DQ assessment terminology37
We enlisted investigators currently engaged in DQ-related work as well as all members of a panel of experts who participated in an earlier effort on DQA reporting sponsored by the EDM Forum38 to provide expertise on identifying existing DQA models, terms, and assessment methods. To obtain a broad representation of DQ terms in current use, we used materials from the previous EDM Forum project,34 such as the Sentinel data characterization routines39 and DQ rules embedded in the Observational Medical Outcomes Partnership (OMOP) and the OHDSI open-source DQ tools.40,41 We also included standard operating procedures for DQA used in past or current projects, published best practices, and DQ publications from both the clinical research and information sciences literature.29,42-48 In total, representatives from approximately 20 of the largest US distributed research networks and large data owners, along with international engagement, either participated directly or were represented in the assessment materials. Collectively, participants represent clinical data networks that contain data on more than 540 million patient records. Based on iterative discussions, an initial draft set of DQ terms, categories, and definitions was developed over 9 months and revised as detailed below.
In July 2014, the EDM Forum hosted two 1-day workshops to review and critique the draft DQ terms and definitions. One workshop enlisted participants representing patients, patient advocacy groups, and policy makers interested in DQ. The second workshop enlisted members of the informatics and CER community. Both workshops explored the need for clarity and transparency in DQ concepts to the specific community and participants. The patients and policy makers workshop also focused on preferred methods for communicating DQ findings and engagement methods to ensure DQ efforts are inclusive of stakeholder perspectives. The informatics and CER community workshop participants reviewed and critiqued the draft DQ terms and definitions. Significant portions of the discussions during each workshop were recorded, and later transcribed, imported into ATLAS.ti (qualitative data analysis software), and reviewed to identify themes and subthemes expressed by participants during each workshop. We incorporated recommended changes to the DQ terms, categories, and definitions identified by workshop participants into the next version of the terminology. We performed a second round of iterative expert consensus development by incorporating comments from a wiki page dedicated to the DQ terminology as well as introducing the revised harmonized draft terminology by webinars to national audiences.
It is estimated that through all outreach efforts, approximately 100 unique individuals from diverse disciplines, as well as US and international networks and projects, contributed to the development and review of the harmonized DQ terminology. We integrated recommendations that had strong consensus throughout the process. We merged and grouped into categories and subcategories DQ terms that addressed similar issues. We included in the terminology terms that focused on DQ features intrinsic to data elements, such as their presence/absence, format, values, and distributions. We did not include terms that focused on DQ features extrinsic to data elements—such as data access, system availability, and security and privacy concerns—and for determining fitness for a specific analysis (fitness for use). We examine this scoping decision in the Discussion. The final set of DQ terms were developed by the primary authors and organized into a logical framework with 3 major categories, which were further separated into 2 evaluation contexts, Verification and Validation.
2. To create the consensus DQA reporting recommendations38
The EDM Forum sponsored the creation of the Data Quality Collaborative (DQC), which provided multiple convening activities (meetings and webinars) and an open-access web-based information sharing environment, to bring together members across informatics, investigator, and methodology communities. The collaborative focused on identifying a set of DQA reporting recommendations that should be included as additional metadata to be associated with observational data. Metadata is data about data. In this case, DQ measures are metadata that provide insights into the quality of the underlying database, data set, or data elements. Envisioned users of these DQ reporting recommendations include data management staff responsible for releasing data for internal or external use; data analysts responsible for combining data across a Learning Health System or research network; clinical investigators using a data set for an analysis; and consumers, both scientific and lay public, of the inferences derived from analytic results.
The DQC conceptualized its work by defining key features that should be contained in a Table 1 for DQA reporting. Table 1 is intended to be analogous to the Table 1 commonly found in publications on clinical studies. Table 1 describes the key characteristics of a study population before presenting analytic findings, such as the distribution of age, sex, gender, race, socioeconomic class, and significant risk factors in the study population. In our context, rather than describing clinical characteristics of a population, Table 1 for DQ reports the key data quality characteristics of a data set or data source (or metadata) that might be used for multiple research and nonresearch purposes.
Table 1
Finding 1.1: Summary Table Describing Harmonized Terminology for DQ Categories. Crosswalks With 10 Previously Published Categories and Frameworks Were Included.
The DQC members (the authors) developed and revised an initial draft set of recommendations at weekly teleconference calls. The initial recommendations were derived by inspecting existing DQ profiling methods used by DQC members, such as the Sentinel data characterization routines39 and DQ rules embedded in the OMOP Observational Source Characteristics Analysis Report (OSCAR) and Generalized Review of OSCAR Unified Checking tools,40,41 standard operating procedures for DQA used in past or current projects or programs, published best practices, and DQ publications from both the clinical research and information sciences literatures.29,42-48 This internal effort elicited approximately 50 initial potential recommendations. In December 2012 and June 2013, EDM Forum's DQC convened 2 face-to-face workshops, held 8 months apart, that focused on reviewing the current draft DQA reporting recommendations. Workshop participants included the DQC, EDM Forum members, and approximately 25 invited contributors who were identified through professional networks, publication authorship, and stakeholder recommendations to represent a broad range of data stakeholders, including data owners, data analysts, clinical investigators, statisticians, and policy makers.
Approximately 50% of attendees attended both workshops. In addition, EDM Forum disseminated a broad-based call for comments to the CER community via sponsored workgroups, a CER-related listserv, electronic newsletters, and personal outreach. EDM Forum provided online access to the evolving set of recommendations and invited comments to be posted. In 2012 and 2013, DQC members presented in 2 national webinars, attended by more than 100 participants, and presented panels at 2 national conferences describing multiple activities in DQA, including draft DQ reporting recommendations. All webinar and meeting participants were directed to the website for reviewing the draft recommendations and for posting comments or were encouraged to directly correspond with the lead author or EDM Forum staff. In June 2014, an updated version of the recommendations was again presented to relevant stakeholders at 2 EDM Forum–hosted workshops, where additional input was solicited.
In response to multiple DQC meetings, public webinars and presentations, email outreach, and targeted solicitations, more than 200 individuals were exposed to the DQ reporting recommendations. In addition to in-meeting and webinar-based comments and discussion, approximately 20 responses were obtained either via the public-facing webpage or via direct email to a DQC member. In total, approximately 50 individual recommendations were obtained by the various stakeholder outreach efforts.
We did not employ Delphi methods and other formal consensus methods to develop the initial or final recommendations. We added or removed from the evolving set recommendations that had strong consensus. We used no formal voting process to determine the degree of consensus.
We continuously revised recommendations in response to stakeholder input and reposted these to the public website. Input was divided roughly evenly between requests for clarification and requests for simplification. We identified no major additional categories via public comments. We posted for review and comment 4 versions of the recommendations. Using informal group consensus, we merged recommendations that addressed similar issues and eliminated recommendations that addressed issues beyond DQ, such as data access, security, and privacy concerns. The final recommendations reflect a compromise between an extensive list and the practical realities of implementation. For example, while it might be desirable to validate data elements against independent, external data sources, such as using US Census data as an external validation source for assessing demographic distributions in an observational data set, we considered these DQ checks out of scope for the DQ reporting recommendations. We reduced the final set of recommendations to 20 DQ features for reporting.
3. To create the survey to elicit individual and organizational barriers to performing DQA and reporting results49
During July 2014 and June 2016, stakeholder engagement meetings were held in Washington, District of Columbia, with CER/informatics professionals from universities, research institutions, professional organizations, federal government agencies, an insurance company, and health care institutions.
Phase 1: Stakeholder Engagement Meetings
2014 Stakeholder Engagement Meeting
We used qualitative methods to moderate both stakeholder engagement meetings. The study team designed stakeholder community–specific discussion guides and led the group discussions. The discussion guides included broad, open-ended questions and prompts to elicit detailed descriptions of stakeholders' views and experiences. The 2014 stakeholder meetings collected recommended additions and changes to the proposed harmonized DQ terminology framework and guidelines specific to the CER community, and limitations and implications of conducting DQA and reporting. Three established models of DQA systems (National Patient-Centered Clinical Research Network, the OMOP ACHILLES Heel, and University of Washington's Find It) were showcased to query recommended additions to a revised version of the DQ terminology framework.
2016 Stakeholder Engagement Meeting
As part of the 2016 meeting agenda, attendees received a summary of results from the DQ Barriers Survey and the research team led a discussion asking stakeholders to report if the survey results were consistent or conflicted with their individual and organizational work as data users and/or analysts. The first part of the discussion was group-based, and the second part took place as part of a “gallery walk,” where attendees could circulate; view survey-identified barriers and solutions on a whiteboard; and validate, comment on, or add to those barriers and solutions based on their own work experiences.
Data Collection
Discussion among stakeholder meeting attendees was digitally recorded following consent from all meeting participants, and the recordings were professionally transcribed verbatim then imported into ATLAS.ti. We analyzed data using qualitative content methods50 and reflexive team analysis, which emphasizes inclusion of emergent rather than a priori themes. We utilized the broader study team to discuss emergent understandings of the data and to check on analysts' preconceived assumptions and biases about the data, as well as to identify themes and subthemes from each meeting's discussions.51
Phase 2: Key Informant Interviews
To provide further insight into the practice of conducting DQAs, during July and August 2014, we contacted several sites and departments engaged in the DQA and reporting and asked them if they would be willing to host a site visit and be interviewed regarding their current practices. The purpose of these interviews was to elicit information regarding each site's current DQA and reporting efforts and investments.
Recruitment
We contacted a convenience sample of 6 sites; 4 sites agreed to host a face-to-face visit and be interviewed. We contacted individuals from both private-sector and academic settings. We offered interview participation to all staff at each institution working in DQA and reporting.
Interview Questions
Using a previously established DQA framework,52 we developed a semi-structured interview instrument consisting of 30 questions to elicit information regarding each site's current DQA and reporting efforts and investments. The interview contained questions about current practices and regulations within the interviewee's organization, DQA requirements, DQA strategies, and remediation plans.
Data Collection
All interviews were conducted one-on-one and took approximately 30 minutes to complete. Detailed notes were taken during interviews. All participants were contacted after the initial interview, reviewed the interview notes, and were offered the opportunity to clarify their responses. To protect the privacy of the participating personnel and sites, names and locations were anonymized.
Data Analysis
Once the site visits and interviews were completed, we aggregated responses within each site; we did not compare or contrast individual responses within or across sites. We evaluated descriptively current DQA and reporting practices conducted at each of the 4 sites. We performed iterative thematic content analysis on the interview notes.
Phase 3: Data Quality Barriers Survey
The final version of the anonymous web-based survey contained 44 self-report items organized within 6 separate subsections and was administered between March and June 2015.
Survey Questions
To ensure participants felt comfortable completing the survey, we included a response option of “I do not feel comfortable answering this question” for every item. In addition to questions about individual and organizational barriers and DQA solutions described below, we asked participants 4 questions regarding demographics, 5 questions regarding current employment, and 8 questions about current DQA practices.
DQA and reporting individual and organization barriers
We used findings from the stakeholder engagement meetings and the site interviews to develop questions about individual and organizational barriers to conducting data quality assessments and reporting DQ results as well as potential solutions to these barriers. We used a 5-point Likert scale (Strongly Disagree to Strongly Agree) to examine agreement to 11 potential individual barriers. We created the organizational barriers survey items by modifying items from a questionnaire created to assess the barriers of implementing quality management in service organizations in Pakistan.53 We used the same 5-point Likert scale to examine agreement to 9 potential organizational barriers. Higher scores indicated a greater perceived individual or organizational barrier.
DQA solutions
We used a 4-point scale (“none” to “a lot”) to assess 7 potential DQA solutions. Higher scores indicated greater perceived organizational support for conducting DQAs. Additionally, we asked respondents to provide any other solutions they felt would support the conduct and reporting of DQAs.
Recruitment
We recruited respondents between 18 and 89 years of age who reported current work with data as a producer (ie, someone who generates data) and/or consumer (ie, someone who uses data generated by a data producer). We collected no identifying information (ie, name, birthdate, social security number) from respondents and participation was completely voluntary. Following the Dillman method of survey research, participants received up to 5 reminder emails; respondents were emailed once a week for up to 5 weeks starting from the date the initial email was sent.54
4. To perform analysis of existing DQ checks against the harmonized DQ terminology56
We recruited project leaders from 4 organizations currently engaged in DQA (Kaiser Permanente's Center for Effectiveness and Safety Research [CESR],57 Sentinel,14,58 the Pediatric Learning Health System network [PEDSnet],59 and the Pediatric Health Information System [PHIS]60) to participate via emailed project proposal. We elicited additional participation from 2 organizations (Duke University School of Medicine's Measurement to Understand the Reclassification of Cabarrus/Kannapolis [MURDOCK]61 registry and the OHDSI program [formerly OMOP]19,62) via outreach to collaborators during monthly meetings held as part of a larger PCORI-funded project (ME-1308-5581).
As demonstrated in Table 6, the majority of participating organizations were part of a clinical research network founded within the past 10 years and had governance that focused on the requirements of external stakeholders (eg, funders). Most of the organizations utilized a distributed network composed of 7 to 50 network sites containing between 11 749 and 660M patient records. The primary analytical focus ranged from chronic disease surveillance (adult and pediatric) and comparative effectiveness and/or quality improvement to generalized large-scale analytics. Common data models were used by 4 of the 6 organizations. In addition to currently engaging in DQ work, we chose these organizations because they represented DQ efforts at varying levels of maturity (eg, from organizations just beginning to engage in DQ to those that currently set the standard for this type of work). As there was no prior work to compare against when developing this work, this approach offered the best opportunity to obtain a well-represented sample of organizations engaged in DQ and their associated DQ checks.
Table 6
Finding 3.1: Results of Mapping 11 000 DQ Checks Obtained From 6 Data Networks Against the Harmonized DQ Categories.
The organizations willing to participate in the project agreed to provide current DQ check documentation in a spreadsheet or PDF table; 2 organizations provided instructions on how to download DQ check information in the form of SQL or R code and 1 organization provided detailed information on the DQ checks applicable to all tables in their database with accompanying data model documentation.
DQ Check Mapping Procedures
DQ check documentation or code received from each organization was standardized (ie, DQ checks were labeled with a name and corresponding description) and stored in a Microsoft® Office Excel 2010 (Redmond, WA: Microsoft) spreadsheet. We created a separate spreadsheet tab for each organization; columns represented the harmonized DQA categories/subcategories and rows represented the DQ checks. We allocated 1 point to the corresponding cell of the harmonized DQA terminology category that each DQ check represented. For any DQ check represented by multiple harmonized DQA categories, a portion of 1 point was allocated—based on the number of represented categories—so that the total points for each row summed to 1. For example, if a DQ check mapped to 2 different categories, each corresponding category of the DQ check would be allocated 0.5 points.
To ensure a systematic approach when mapping the DQ checks to the harmonized DQA categories, we developed conventions to operationalize each of the individual categories within the data verification context of the harmonized DQA terminology. Examples of the conventions by DQ check type are provided below:
- Conformance
- –
Value: The DQ check examines the formatting of variables (eg, length, string/numeric variable typing).
- –
Relational: The DQ check examines the relational database constraints (eg, primary and foreign key relationships), as well constraints specified by the metadata (eg, table existence).
- –
Calculation: The DQ check examines computationally derived variables.
- Completeness
- –
Atemporal: The DQ check examines counts of missing or available variables at 1 time point.
- –
Temporal: The DQ check examines counts of missing or available variables across multiple time points.
- Plausibility
- –
Uniqueness: The DQ check tests for duplicated variables, variable values, and records.
- –
Atemporal: The DQ check examines the range or distribution of a single variable (eg, height or weight) or the relationship between multiple variables (eg, gender and procedure type) to determine if values are correct.
- –
Temporal: The DQ check examines the believability of data values over a certain period of time (eg, hours, days, years).
In addition to these conventions, we identified from each organization example DQ checks representative of each harmonized DQA category, such as those listed below. Each DQ check's correspondence to the harmonized DQ terminology is provided in parentheses.
- CESR
- –
Check variable type (conformance: value).
- –
For inpatient stays, compute the length of stay (conformance: calculation).
- MURDOCK
- –
Identify records with NULL date of birth (completeness: atemporal).
- –
Values for height are not between 36 and 84 (plausibility: atemporal).
- OHDSI
- –
The distribution of age at first observation period (plausibility: atemporal).
- –
The number of visit records with an end date occurring before a start date (plausibility: temporal).
- PEDSnet
- –
Compare the number of people in the person table against the number of people in the observation period table (conformance: relational).
- –
Describe missing values (completeness: atemporal).
- PHIS
- –
Length of Stay does not include time before admit order, ie, time in emergency department observation (conformance: calculation).
- –
Medical discharge values are missing for more than 8 consecutive hours (completeness: temporal).
- SENTINEL
- –
At least 1 PatID in the enrollment table is not in the demographics table (conformance: relational).
- –
Enrollment end occurs more than once in the file in combination with PatID, medical and drug coverage (plausibility: uniqueness).
Using these conventions and example DQ checks, we performed mapping of the full set of DQ checks 4 times. We discussed with members of the research team any check not able to be clearly mapped until a final mapping consensus was reached.
Analytical and Statistical Approaches
We had no formal hypothesis with associated analytical or statistical models. Numerous DQA measures include very simple statistical results, such as means, medians, extreme values, and percent missingness. We analyzed the Barriers to Data Quality Assessment and Reporting survey results using univariate and bivariate statistics as well as exploratory factor analysis. We performed factor analysis separately for the individual and organizational barriers scales. Data from the Likert scales were collapsed, primarily due to small sample sizes and to limited variability in responses for many items. We include the statistical analysis description from the Methods section of that manuscript49:
Statistical Analysis
Analysis was performed using SAS software (version 9.4). Univariate statistics were used to examine the frequencies of responses to survey questions. Chi-squared and Fisher exact tests were performed to investigate whether responses differed by job characteristics, including the numbers of hours per week spent working on DQ issues (0-9 hours/week versus 10 or more hours/week), the type of position (data producer, data consumer, or both), and the number of data sites participating in the respondent's network (small: 1-20 sites versus large: more than 20 sites).
For bivariate analyses, the response categories were collapsed into 2 categories, “Strongly Agree/Agree” versus “Strongly Disagree/Disagree/Neutral” for barriers items and “None/Some” versus “Mostly/A Lot” for solutions items, to allow for statistical testing given the limited sample size. Exploratory factor analysis, with principal components analysis as the method of factor extraction and varimax rotation, was used to explore the individual barriers and organization barriers scales for correlated variables that reflect an underlying factor structure in the data. The Kaiser-Meyer-Olkin (KMO) test and Bartlett's test of sphericity were used to determine factorability of the 2 sets of items. Items that loaded strongly on a factor were averaged into a subscale for each respondent; this method is preferred over using factor scores as it allows the subscale values to be interpreted using the Likert scale of the original item responses. Analysis of Variance (ANOVA) was used to compare the overall individual and organizational barriers scales, as well as the subscales derived from factor analysis.
Conduct of the Study
Two components of the project required institutional review board oversight/review. For the technical programming activities performed in aims 2 and 3, we required a deidentified database derived from actual electronic health records. We created a deidentified version of the OMOP CDM (partnership-established model informing the appropriate use of observational health care databases) using an existing fully identified version of an OMOP database at Children's Hospital Colorado (OMOP De-ID Repository). We obtained IRB approval for the deidentification procedures that led to a determination of compliance with statistical deidentification. The De-ID OMOP database enabled us to create realistic DQA statistics that could be used to populate an initial version of the DQA-CDM for aim 2 and for DQ visualizations for aim 3. Our second IRB submission focused on the conduct of the online anonymous Barriers to Data Quality Assessment and Reporting survey developed for aim 4. We include the overall IRB summary statements from both submissions.
Creating the OMOP De-ID Repository for aims 2 and 3:
This project seeks to utilize de-identified data from the Children's Hospital of Colorado (CHCO) that has been transformed into the Observational Medical Outcomes Partnership (OMOP) common data model (CDM). More specifically, we propose a system that will facilitate the continual transfer of data from the currently existing CHCO OMOP CDM limited data repository into a completely de-identified OMOP CDM data repository. Upon completion of a given data transfer, we will utilize the clinical pediatric information captured in the OMOP CDM de-identified data repository to learn from existing medical data by applying our current methods and tools for analyzing and visualizing trends and outcomes using observational EHR data, and then to improve upon these methods through evaluation and validation. Our ultimate aim is to fully utilize the de-identified information in medical records for use in guiding pediatric clinical care.
The proposal was submitted as an expedited protocol, but was determined by the IRB to be Exempt/Not Human Subjects Research.
Creating the DQ barriers survey for aim 4:
The goal of this study was to gain information regarding current data quality assessment practices as well as examine potential barriers to improving or expanding current data quality assessment activities/investments. Specifically, we designed a survey to examine data quality assessment and individual- and organizational level barriers to performing data quality assessment. We administered this survey to over 100 individuals aged 18-89 years, who worked with data, either as a data consumer or producer. Participants were recruited from local- and national-level conferences and meetings and were contacted via listservs and asked to complete the survey via REDCap. No information which could be used to identify participants was collected and all results from this study were presented in aggregate form. Further, all data is anonymous since participants did not provide any identifying information (name, birthdate, social security number) to the researchers at any point.
The proposal was submitted and approved as an Exempt/Non-Human Subject Research protocol.
Results
Traditional clinical studies often organize results using the PICOTS format (population, intervention, comparison, outcomes, time frame, and setting). Our work does not include a formal study so the PICOTS format is not used as suggested by PCORI. Instead, we have pulled the key results graphics from our published manuscripts or additional project deliverables that did not resulted in archival publications but were presented at academic informatics meetings or workshops.
We present 7 key findings generated by this project, which represent a comprehensive inventory of all work products. We have organized these findings into 3 Findings Categories: (1) 2 findings with broad impact on DQ policies and processes, (2) 2 findings related to investigator and patient engagement in understanding DQ transparency, and (3) 3 findings with narrower technical outcomes. Each finding is aligned with 1 or more specific aim. All publications and technical artifacts are freely available via an open-access journal and open-source websites.
Category 1: Findings With Broad Impact on Data Quality Policy and Processes
Finding 1.1: A Harmonized Terminology for Data Quality Assessment Categories (Aim 1)
Table 1 presents the final result of a 1.5-year effort to obtain community consensus of key DQ dimensions.37 We divided 3 major categories (conformance, completeness, and plausibility) with subcategories into 2 contexts (verification and validation) to form the final terminology. Each category builds on the previous category. Conformance focuses strictly on the agreement of values against technical specification without regard to the amount or believability of those values. Completeness focuses on the absence of data of a variable, again without regard to the believability of those values. Plausibility focuses on the believability or correctness of data that agree with technical specifications (conformance) and are present in the expected amount (completeness). We aligned 10 previously published DQ models with the harmonized DQ terminology to validate the coverage of the harmonized terminology.37 A subsequent publication (Finding 1.2 below) validated the coverage of the consensus terminology against more than 11 000 DQ checks.56
Finding 1.2: Guidelines for Transparent Reporting of Data Quality (Aim 1)
STROBE (Strengthening the Reporting of OBservational studies in Epidemiology) is an international collaborative that focuses on improving the quality of observational studies.63,64 Our work similarly focused on improving transparency, and therefore confidence, in the data sources underlying observation studies. We examined the current STROBE recommendations for statements regarding DQA when using observational studies. The current STROBE Statement includes 2 items directly relevant to DQ reporting:
- STROBE Item 8: Data sources/measurements: For each variable of interest, give sources of data and details of methods of assessment (measurement). Describe comparability of assessment methods if there is more than 1 group.
- STROBE Item 12(c): Explain how missing data were addressed.
We developed additional recommendations that extend the STROBE statements related to 2 existing DQ items with more detailed structured reporting requirements.38
Using the same format as the existing STROBE Statement, Table 2 presents 20 new recommendations for reporting results on DQ.63 Six recommendations apply to documentation of data capture systems. Three of the 6 recommendations describe features of the source data system(s); the remaining 3 describe features of the target data system. Five recommendations focus on data processing and data provenance. Data provenance is concerned with ensuring that all the processes applied to a data element from initial creation to final storage are made explicit. For example, a data element may be transformed, recoded, combined with other variables to create derived variables, removed as an outlier, replaced with a fixed value or flag, imputed when missing, or subject to other alterations. The characterization of data elements group lists only 4 reporting recommendations. Yet in actual implementations, this set of recommendations is likely to represent the component that consumes the largest amount of resources. The recommendations in this group are more computational and focus on describing distributional characteristics of the target data set. These recommendations are also the most technical and statistical, using methods such as goodness-of-fit testing for variables that have an expected distribution, state-transition checks for variables that are expected to exhibit a specific sequence of values over time (eg, inpatient admission event should be followed only by a discharge or death event; a death event should not be followed by a clinic encounter), or primary/foreign key constraints such as that the provider listed in a patient's record (a foreign key) must always be a provider already present in the provider table (the primary key).
Table 2
Finding 1.2: Twenty Recommendations, in STROBE Format, for Comprehensive DQ Reporting.
The fourth reporting recommendation group, “Analysis-specific Data Elements Characterization” is even less prescriptive. This grouping recognizes that is it not possible to anticipate all the ways in which data are to be used in a complex analytic context; therefore, highly specialized and specific DQ checks and reporting should be performed when the data are used in a focused analytic context. The reporting recommendations in this section highlight that additional DQA checks should be targeted to key variables that are used to identify cohorts, detect outcomes, define exposures, and participate as covariates. Where these variables may be affected by other related (perhaps causal) variables, these related variables should also be included.
Category 2: Findings Related to Investigator and Patient Engagement in Understanding Data Quality Transparency
Finding 2.1: Patient Engagement in Data Quality Assessment (Aims 1 and 4)
In July 2014, EDM Forum hosted the first face-to-face engagement with patients, patient advocates, and policy makers. While it was not an intended focus of the discussion on DQA results, the conversation took an unexpected turn toward the role of early and active patient engagement in ensuring electronic health record data are initially recorded correctly, which would ensure that subsequent secondary use of these data had accurate values. Engaging patients as an important “up-front data steward” would result in patients being:
“more engaged and ready to communicate with their doctor and engage in health care decision making as the result of this (DQ review) process.”
The desire to be engaged directly at the point of care extended to the entire data lifecycle, including the data collection methods:
“You bring them [patient/consumers] in at the data collection phase, and you get their input on how to collect the data from different populations, especially underserved populations that might not trust a clinical research system, right, so you bring them in and … that middle area of [data] cleaning, and analysis would be really be done by the researchers, and then would be reflected back to the beginning [patient/consumers] groups to say ‘This is what we did, and this is what we found, and yes our methods are sound; you can trust us.’”
An initial diagram of the data lifecycle created by the project team (Table 3A) was altered by the patient participants to include a new Level 0 point-of-care data review (Table 3B). One of the patient participants captured this sentiment in a published paper that was directly motivated by this discussion.65 These results were also presented as a case study in qualitative analysis by J. Barnard at the 2016 American Public Health Association meeting. A manuscript comparing the qualitative findings across the 4 face-to-face meetings is in early draft
Table 3
Finding 2.1: (A) Premeeting and (B) Postmeeting Representation of Data and DQ Life Cycle. A New Level 0 Was Added by the Patient Representatives.
Finding 2.2: Individual and Organizational Barriers to Data Quality Assessment (Aim 4)
Table 4 and Table 5 provide only the results of aggregated factor analysis from the online survey, which attracted 141 respondents (111 analyzable responses). Additional univariate and bivariate analyses are described in the published manuscript.49 Factor analysis revealed 3 individual barriers (personal consequences, process issues, and lack of resources) and 2 organizational factors (environment/support and practices). Performing DQA and reporting DQ results require significant resources. Attendees of the first stakeholder meeting and survey respondents agreed that a lack of funding and allocated time to conduct DQA as well as a lack of guidelines on definitions of desirable versus undesirable DQ results were barriers to DQA and reporting. Conversely, survey respondents were much less concerned than meeting attendees about potential personal, professional, or institutional reputation–related punitive consequences of poor DQA results. Meeting attendees and survey respondents agreed that organizational resources and support and established standards and processes for conducting DQAs were potential solutions to the identified DQA barriers. Verification of the identified DQA barriers and solutions at a second stakeholder meeting revealed additional barriers, including a lack of standardized guidelines and a feeling of powerlessness to impact the quality of the data sets received.
Table 4
Finding 2.2(a): Results of Factor Analysis From Anonymous Online Survey of Individual and Organizational Barriers to DQ Assessment and Reporting.
Table 5
Finding 2.2(b): Results of Factor Analysis From Anonymous Online Survey of Individual and Organizational Barriers to Data Quality Assessment and Reporting.
An important factor for encouraging the reporting of errors is the establishment of an infrastructure that facilitates DQA. Recent work to harmonize and standardize the reporting of health information found that 2 frameworks were needed: a conceptual framework for comparing content and a measurement framework, like the Rasch measurement model.66 Using these frameworks, the authors developed and tested an International Classification of Functioning architecture that allows patient information to be consistently documented when data have been collected using disparate instruments. There is no equivalent architecture in DQA.
Category 3: Findings With Narrower Technical Outcomes
Finding 3.1: Distribution of DQA Checks Across 6 Large Data Networks (Aims 1 and 4)
Using the harmonized DQ categories described in Category 1, DQ checks from 6 data networks were categorized.56 Of the 11 026 checks analyzed, only 3 did not fit into the harmonization framework (final N = 11 023). Table 6 (top) shows key descriptive features of the 6 participating networks, illustrating a wide range of network size, age, technologies, and depth of DQA. Table 6 (bottom) shows the distribution of DQ categories within each network (bottom left) and aggregated across all 11 000 DQ checks (bottom right). These findings provide validation for the harmonized DQA terminology, highlighting its ability to successfully represent a robust sample of DQ checks across highly diverse networks. We mapped provided DQ checks to all the harmonized DQ categories in the data verification context. We did not consider for DQ checks within the data validation context for mapping due to the low number of provided checks that mapped to this category. These types of checks are much harder to perform than those within the data verification context and thus are harder to standardize and compare.
As shown in Table 6, there was wide variability in the distribution of mapped DQ checks among the organizations. Three of the organizations (Sentinel, PEDSnet, and PHIS) had similar DQ check coverage distributions. These organizations were focused on meeting the DQ expectations of external stakeholders, had distributed network sites (≥ 8 sites) with more than 5 million patient records, and had extremely well documented DQ checks and procedures for evaluating their data. These organizations primarily evaluated atemporal plausibility, atemporal completeness, and value conformance.
CESR is also a distributed clinical research network like Sentinel, PEDSnet, and OHDSI, but it must meet the DQ expectation of both internal and external stakeholders. Like Sentinel and PEDSnet, CESR has developed its own tools for performing DQA. Unlike these organizations, CESR's DQ checks are not publicly documented and are not publicly available, but are freely shared upon request; CESR may be subject to internal restrictions regarding how DQA is performed. The bulk of its DQ checks focused on value conformance, relational conformance, and atemporal completeness.
MURDOCK is a registry and biorepository, which is managed by internal and external stakeholders. This organization collects data from participants at multiple sites and enrollment events (eg, health fairs coordinated by MURDOCK study office), but stores the fewest number of patient records (< 12 000) compared with the other organizations. Although it collects EHR data for some enrolled participants, the registry does not contain all patient records from any of the participating facilities' EHRs as the others do. Like OHDSI, most DQ checks in MURDOCK were focused on atemporal plausibility.
The OHDSI open science collaborative is focused on large-scale analytics for clinical characterization, population-level effect estimation, and patient-level prediction. OHDSI is the only organization that has distributed DQA coordination (ie, different individuals responsible for reviewing the DQ of sites in their network). This is a distinguishing characteristic in that all the other organizations are in the position to “reject” data before use on a routine basis, but as an open collaborative, OHDSI does not play that type of central role, leaving individual investigators/projects to make a fitness-for-use determination. OHDSI DQ checks were primarily focused on atemporal plausibility.
The key findings from this exercise were that the harmonized DQ categories successfully captured the DQ checks performed in real-world practice in large-scale data networks, and that the distribution of DQ checks varied greatly across the networks. The manuscript proposes a “data quality maturity model” to describe DQ programs that evolve from simple DQ checks to more complexity and diversity as the data network matures and more resources are devoted to establishing DQ evaluation procedures.
Finding 3.2: A Common Data Model (DQA-CDM) for Storing Data Quality Results (Aim 2)
Only a few well-funded, large projects have invested significant resources to develop formal DQA procedures and programs. Mature DQ programs have evolved over many years, reflecting substantial investments in developing DQA processes, measures, reports, and visualizations.67,68 Newer or smaller projects struggle to create (or re-create) similar DQA processes with fewer resources. Currently, DQA software is written by project-specific programmers against specific source data models. These programs generate DQ measures directly from a source data model or write into summary tables that are then used by DQA reporting or visualization tools. In either case, DQ routines are project specific. Innovative DQ routines or visualizations created by Project A are not available to Project Z (Figure 2A). To explore methods for sharing DQA tools, we hosted a 2.5-day DQ Code-A-Thon in Denver. For the Code-A-Thon, we implemented a prototype CDM for storing DQ results that were obtained from markedly different source databases: DQ summary measures obtained from Children's Hospital Colorado and the CMS 1000-patient SynPUF data in OMOP format, sample data from Sentinel data characterization programs, and results from the PHIS quarterly DQ report were transformed into a CDM for DQA results, called the DQA CDM (Figure 2B, Table 7). Four teams of DQ Code-A-Thon participants created a wide range of visual and analytic mock-ups.

Figure 2
(a) Current and (b) Future State for DQA-CDM for Sharing DQA Results.
Table 7
Finding 3.2: The Entity-Relationship Diagram of the CDM for Storing DQ Results (DQA-CDM) Used in the DQ Code-A-Thon.
Final products by those teams include a DQ platform, suggestions for DQ reporting to naïve users, impossible (ie, nonsensical or contradictory) data, and temporal anomaly detection. The DQ platform team worked on proposal for a framework to support DQ reporting based on summary statistics data stored in the DQA-CDM. The “naïve users” team came up with insights on presentations of DQ measures to make them understandable by naïve users such as patients. Using provided data in DQA-CDM format, the “impossible data” team suggested a top-down approach, which provides an overview of data with the ability to drill down to narrower levels of data aggregation. Finally, the “temporal anomaly detection” team successfully performed a lossless conversion of data stored in DQA-CDM structure to the OHDSI ACHILLES data model and conducted sophisticated temporal statistical analysis to classify normal and anomalies in the prevalence of influenza between 2010 and 2014.
A team of visualization experts who participated in the DQ Code-A-Thon was assembled to create illustrative visualizations that were used in a later stakeholder meeting. Table 7 shows the DQA-CDM developed for the DQ Code-A-Thon and sample visualizations that were created. Data sets created for the Code-A-Thon are freely available on a dedicated GitHub site (https://github.com/DQCode-A-Thon).
Experience with the prototype DQA-CDM revealed critical shortcomings:
- While the logical structure of the DQA-CDM was independent of the source data models (OMOP, Sentinel, and PHIS), the contents/semantics of the data elements were not. For example, a DQA measure that calculated the number of encounters per month would store “visit_occurrence” (OMOP) or “encounter” (Mini-Sentinel) or “visit” (PHIS) as its Dim_name_1 in the Dimension_Set table and “Month” in Dim_name_2. A DQA tool using this version of the DQA-CDM would need to know the terms used by all the source data models to express encounters (visit_occurrence, encounter, and visit) to display the number of encounters per month. Similarly, terminologies from different encoding systems are used in different source data models. For example, OMOP uses SNOMED as its standard for diagnosis terminologies while Mini-Sentinel uses ICD9 or ICD10. The use of different encoding systems makes it impossible to compare statistical results from different sources even though they are stored in the same data structure.
- Statistical results were represented using ad hoc terminology such as “Mean,” “Median,” “25th percentile,” “%Change,” and other labels based on DQA measures.
- Different relational database management systems (RDBMS), such as Oracle, SQL Server, MySQL, and Postgres, and SAS-based data sets, differ in how data are stored and queried. These differences cause tools that use these databases to become dependent on the specific underlying RDBMS. Flat-files such as comma-separated values or the more structured JavaScript Object Notation eliminate these dependencies but require generating and manipulating data files rather than tables within an RDBMS.
- While DQ CDM Version 1 was able to provide a generic structure for summary statistics, the convention for the metadata that describes the content of each DQ measure and their relationship must be defined. Inconsistent definitions of the measure metadata could create discrepancies between instances of the DQA-CDM, making it difficult to directly compare DQ results.
A manuscript describing our initial experiences and the limitations discovered during the DQ Code-A-Thon is in progress.
Finding 3.3: Visualizing Data Quality Results (Aims 2 and 3)
Data visualization is a powerful tool for quickly detecting complex multidimensional data features. Yet most DQ tools and reports use a large number of tables and simple histogram or timeline plots for categorical and time-varying parameters, respectively. More complex interactive visualizations, such as drill-down heat maps, radial plots, and temporal flow diagrams, are starting to appear in the scientific data visualization community but have not yet been applied to visualizing DQ results. Aim 3 brought together technical and nontechnical experts in DQ, informatics, CER, and data visualization for a 2.5-day DQ Code-A-Thon in Denver to explore new models for data visualization using DQ summary statistics stored in Version 1.0 of the DQA-CDM (Table 8). By purposeful selection, a balanced set of scientists, programmers, and health services investigators were invited to attend. In total, 22 individuals participated in the event. Instructions/context-setting for the event stated the following:
Table 8
Finding 3.3: Three Presentative Visualizations Created During and After the DQ Code-A-Thon and Used for Discussion in the Second Set of Face-to-Face Meetings.
Code-A-Thon Aim: The goal of this weekend will be to have small teams of programmers, visualization experts and DQ project leads compete to create visualizations of DQ results produced by the project's Data Quality Common Data Model (DQ CDM). Be creative, take risks, be as wacko as you want. We want to come out of this meeting with ideas and prototypes that go far beyond the current tools and graphs. Reach high to go after “new data characterizations/visualizations to summarize data, as well as better mechanisms to explore the summary data so that unusual patterns can be more easily detected, diagnosed, and resolved” (Pat Ryan).
Equally important, please have fun working with known and new colleagues who are equally passionate about data quality and data quality assessment. We are hoping that enduring collaborations will emerge from this long weekend together. The DQC Code-a-Thon will conclude with presentations of each team's DQ visualizations and code. Prizes will be awarded on Sunday to the team with the best and most creative visualizations.
There are different “customers” that we envision would benefit from the data quality assessment products that we will develop at the DQC Code-A-Thon:
- –
Data analysts who are responsible for understanding the strengths and weaknesses of data sets that they receive from data owners. These individuals tend to be “in the data”
- –
as part of their daily responsibilities. They are comfortable with data exploration tools and are used to looking at data quality results for anomalies that might indicate data quality issues
- –
Data users, typically (in our setting) clinical investigators, who are more focused on using data to answer a specific research question. While these individuals are involved in using data for their research, they tend to not be as well versed in the nuances of complex data sets nor do they tend to spend time digging into the nitty gritty features of data sets
- –
Data consumers, which for our work, are defined as patients, patient advocates, and health care policy makers. These individuals use the results of data analyses to understand who these results affect their care (patients, patient advocates) or may alter health policy (patient advocates, health care policy makers). Most in this group are used to working with highly “processed” data in aggregate form, usually as summarized by statistical models, plots and other high-level summary. Their interest in data quality is more indirect – what do I need to know about the underlying data quality that might affect how I interpret these findings for myself or for setting new policies. A brief perspective on data consumer needs is available from Erin MacKay of the National Partnership for Women and Families, a national consumer group.
Work products developed for these customers are likely to be very different as these groups have markedly different experience with and exposure to detailed large-scale clinical data. Telling the data quality “story” (“What do these findings mean to me?”) is the core challenge to be addressed by this DQC Code-A-Thon. You are free to pick whichever customer type (or more than 1 customer type if you are very brave), to frame your work.
The use cases below are combined/modified from more detailed use cases provided by Pat Ryan (OHDSI) and Meredith Nahm (Duke). Their original use cases will be posted on the EDM Forum wiki. These use cases are provided only as examples and are not intended to be directive or limiting—folks should feel free to wander down their own paths.
- –
Can we find anomalies in prevalence trends over time (eg by month/by year) with adequate sensitivity/specificity?
- –
Can we find improbable/implausible values for categorical distributions?
- –
Can we find implausible values (crazy lab measures, diagnoses in the wrong gender, physically impossible such as negative weights, etc.)? Can we drill down to which site, which data element, which encounter?
- –
Implausible health patterns, such as outpatient visits subsumed within inpatient visits, visits/procedures recorded after death date or before birth date
- –
How to describe missingness – amount and patterns
- –
Can we view results of comparisons between 2 independent data sets to identify areas of significant inconsistency? For example, comparison of Diagnoses from CMS claims data and Health Records, or between problem lists and Diagnoses, or Diagnoses for same patients between 2 different facilities (or in our setting, difference across 2 different DQ CDM instances)
- –
Can we use temporal disturbances (temporal gaps) to signal potential exit by population members? Ability to compare by facility
Table 8 provides 3 examples of the visualizations that were created at the DQ Code-A-Thon and presented at the 2 stakeholder meetings. Figures (A) and (B) in Table 8 present 2 different approaches for an overall dashboard of DQ measures based on the DQ harmonization framework (Finding 1.1). While both approaches categorize DQ measures into 3 main DQ measures—completeness, fidelity, and plausibility—the visualization teams envisioned a different end-user. Figure (A) is a more technically oriented comprehensive overview that combines DQ categories and subcategories on a single screen, allowing for direct comparisons between the outputs of the methods within subcategories (eg, density temporal versus density atemporal) but with much more “data density” for the user to assimilate.
Figure (B) presents a less dense but less comprehensive top-down approach, including an overview evaluation of each of the main DQ measures and the ability to drill down to investigate how DQ measures at the lower level (eg, patient data completeness) constitute the overall DQ measures. Figure (B) requires more “clicks” to reach more detailed displays. Figure (C) is an interactive tool that uses a multiaxial display method called parallel coordinates to compare aggregate data among different cohorts of patients. For example, this tool displays DQ features from 8 tables simultaneously and allows real-time filtering to display subsets of data that may be of interest to a specific question. The intended audience for Figure (C) is investigators seeking to understand fitness-for-use for their analytic needs. More visualizations, including the interactive version of the Parallel Coordinates tools, are available at https://github.com/DQCode-A-Thon.
The visualizations generated during the DQ Code-A-Thon were used to guide the discussions with the 2 face-to-face meetings held toward the close of the project with patients, patient advocates, and policy makers (June 23, 2016) and informatics and CER investigators (June 24, 2016). Summaries of themes and recommendations from 2 meetings are posted at http://www.edm-forum.org/collaborate/collaborativeprojects/dataquality/dqwiki. In brief, common themes included the following:
- Dashboards must provide visual cues regarding where DQ issues exist that require additional exploration, although what constitutes a relevant DQ issue may be context specific, varying for each use depending on the question being considered (fitness for use). Techniques for obtaining or inferring the most relevant variables and features for context-specific explorations are the focus of future work. Initial thoughts include highlighting all variables used to define the study cohorts and strata, exposures, possible covariates, interventions, and outcomes, perhaps by tying the DQ visualizations with a structured representation of the study analytic plans.
- Users should be allowed to set their own thresholds for alerting on DQ based on the need for higher- or lower-quality data depending on the intended conclusions to be drawn (high-level broad question versus detailed hypothesis-driven research question).
- The completeness dimension must have a denominator—a variable is complete (not missing more values than expected) compared with what? The reasons data are incomplete are important to determine if there is significant correlated missingness (missing not at random).
- Error, bias, and accuracy are critical components of data plausibility.
- Completeness and fidelity (assumptions of the data, given the problem or task, are not violated) can be used as a signal to explore and assess the plausibility of the data.
- A visualization environment must allow for end-user customization, including DQ thresholds, relevant data domains, subsets (cohorts, time periods), and relationships.
- Dynamic data exploration capabilities, such as drill-down and cross-correlations, are important features because they allow the user to see the data from different perspectives (eg, counts of visits by satellite clinic, over time) and gather information about the quality that wouldn't otherwise be accessible.
- While the high-level summary visualizations were easily understood, they also provided very limited insights into the specific DQ features of the data.
- Assessing a data source's fitness for use (highly specific DQ use cases) has been the most common way the DQ visualization tool was used.
- The lack of known “gold standards” for the quality of a given data source can make interpreting DQA results difficult.
- Fitness-for-use scenarios often blurred the distinction between exploring DQA results to assess DQ and exploratory data analysis of a research hypothesis. Both tasks involve calculating and assessing high-level summary statistics relative to a specific need. In DQ, the task is “Are these data good enough for my intended use?” whereas in the exploratory data analysis setting, the task is “Are these data suggesting the story (hypothesis) I am seeking to answer?” The use of specific summary statistics that align with the area of interest makes these 2 use cases appear nearly overlapping to workshop participants.
The final discussions were indeterminate in setting a clear agenda for a next cycle of DQ visualization displays and tools. A high degree of free-form user-driven interactivity but with some guidance based on user-specified DQA trigger thresholds was a common theme across stakeholders.
Discussion
Methodological Gaps
Data quality continues to be a large concern for comparative effectiveness investigators. The PCORI Methodology Committee sponsored a full-day symposium dedicated exclusively to missing data in electronic health records (https://www.pcori.org/sites/default/files/PCORI-Data-Quality-and-Missing-Data-Workgroup-Summary-121015.pdf). Five Methodology Standards (MD-1 through MD-5) focus on the source, handling, and impact of missing data and the effect on study results. Yet missing data is only 1 of many dimensions of DQ that need to be assessed. Our work fills in the much larger picture of assessing DQ in a comprehensive, standardized manner. Our 7 findings address a broad range of DQ issues—DQ terminology, representation, visualization, reporting, and barriers. Our work also has had the side effect of developing a sustainable community of like-minded informatics professionals and researchers who meet monthly to discuss new results in DQ research. Two PhD dissertations (Nicole Weiskopf, Columbia University; Steven Johnson, University of Minnesota) have emerged from this community. Integration of the categorization and recommendations from the DQC into the PCORI Methodology Standards is currently in progress.
Study Results in Context
Work products produced in aim 1 were carefully aligned to existing publications and validated using existing DQ measures. Specifically, the harmonized DQ categories were successfully aligned to 10 previously published DQ frameworks and were mapped to more than 11 000 existing DQ checks. Novel findings include the development of a CDM for DQA findings (DQA-CDM), the quantification of differences in the distribution of DQ checks across 6 national data networks, and the analysis of individual and organizational barriers to DQA. This work developed and validated a common language for communicating DQ findings; a fundamental component for efficient, accurate, and reproducible reuse of EHR data; assessing new DQ research findings against existing work, an issue that has plagued the DQ research literature and practitioners.
Update of Study Results
All materials have been made freely accessible to the public via the EDM Forum wiki or a public-facing GitHub code repository. For the published recommendations (harmonized DQ categories and DQ reporting recommendations), anonymous open comments can be added, which will notify the wiki owner and the project team.
Subsequent updates to the recommendations may be published if new best practices or additional contexts not previously considered arise. For example, 1 limitation of both sets of recommendations that appears in the published manuscripts is that the scope of recommendations is directed toward longitudinal clinical data, especially EHR data. It is not known how well these recommendations would fit other relevant sources, specifically genomic, biologic, unstructured (notes), and social media data, which are growing much more rapidly in relative size than are traditional structured clinical data. Expanding the engagement of data owners and users to include these domains is a necessary next step.
Generalizability
This work is derived from community engagement and consensus. The conclusions represent the opinions and best practices of those who participated—either in the DQC, the online wiki comments, or the anonymous online survey (DQ Barriers). While we estimate that more than 150 individuals contributed at different times for different work products, the final results represent the synthesis of input only from the participants.
We have emphasized this limitation in all our publications and have enabled online mechanisms for continued input from later participants. However, relevant communities that are not included or do not participate may have significantly different approaches to DQA.
We will continue to promote the current work products and to direct interested participants and communities to engage with online comments and participation in our monthly online community webinars, to encourage broader engagement with missing constituencies.
Subpopulation Considerations
Not applicable because this was not a CER study.
Study Limitations
One key limitation has been mentioned multiple times previously—the narrow focus on data owners and users of a limited class of electronic health data, especially EHRs and administrative data. Recommendations appropriate to this class of data owner and user may not apply to users of other types of relevant health data.
A second key limitation is the use of a community consensus methodology in real time rather than a formal approach, such as the Delphi method, for Findings 1.1 and 1.2.69 The team preferred to increase direct engagement of members in a community consensus method rather than a Delphi method believing it would enhance and facilitate building of consensus instead of the rigidity imposed by consecutive rounds of comment and review that occurs with a Delphi method. However, a community consensus approach has a potential risk of undue influence on consensus development from certain thought leaders or influential individuals. The face-to-face meeting facilitators addressed this known risk by moderating the consensus-building discussions so no individuals dominated the discussion or prevented others from sharing their views. A third potential limitation is the use of an anonymous survey for identifying DQ barriers (Finding 2.2). By not identifying and tracking who completed the survey, we cannot guarantee that individuals did not respond to the survey multiple times, although there was no evidence of multiple responses and it is highly unlikely to have occurred.
Importance/Relevance to PCOR
The objective of this work is to improve the quality, quantity, and transparency of DQA activities in observational research. The DQ harmonization work (Finding 1.1) was developed in response to the diversity of terms that prevents direct comparisons across DQ efforts. Since publication of our DQ harmonization paper, publications by multiple DQ community members have framed their work using the harmonization framework.70,71 In particular, the publications by Dziadkowiec and colleagues72 and Khare and colleagues73 use both the DQ framework (Finding 1.1) and the DQ reporting recommendations (Finding 1.2) to organize their results.
Additional work continues on improving the DQA-CDM (Finding 3.2) to eliminate limitations discovered at the DQ Code-A-Thon (Finding 3.3), with a focus on using established data standards, such as HL7 FHIR74,75 and NIH Common Data Elements.76 This work has evolved to focus on establishing a metadata standard for including DQ results as part of a machine-readable description of a data resource that could be included in automated data discovery engines such as the bioCADDIE project.77,78
Future Research
We anticipate new work will focus on implementing the multiple recommendations and best practices. A key area of new research is defining measures of impact, especially on “better science,” that can be attributed to implementing recommended DQA practices.
While the business literature has multiple case studies of the impact of poor DQ, there are no similar studies in health care. Nor are there studies that objectively quantify the costs and benefits of implementing DQA and improvement programs. Partnerships with established information quality programs, such as exists at the University of Arkansas, Little Rock, may help frame a novel study design to not only elucidate the costs but also document the benefits of improved DQ on patient care (via more accurate decision support) and on clinical research (via stronger statistical models). From a technical perspective, more work is needed to revise the CDM for DQ measures (DQA-CDM) so that DQ tools and visualizations are sharable across projects. Figure 2 illustrates this vision—where DQ tools that currently are tied to a network-specific source data model (Figure 2A) are replaced with the ability to use DQ tools by any data network irrespective of the source data model (Figure 2B). This future state would allow for DQA innovations to be shared throughout the DQ community and reduce the need for new data networks to essentially replicate DQA work already implemented by others.
Conclusions
The arrival of the era of larger volumes of electronically available data has increased the availability and reuse of EHR data. While these data have great potential for significant advancement in clinical practice and research, the quality of these data sources ultimately determines their utility. To fully understand and accurately characterize the limitations of these data sources, establishing standardized, validated methodologies for assessing and reporting DQ is crucial. And yet currently no standard practices and metrics to describe DQ exist, and, as a result, the current process is ad hoc and nontransparent to data users and data consumers. This project focused on creating an agreed-on set of definitions and recommendations to guide DQA and the presentation of results, and presenting those results in understandable visualizations. We developed the DQ terminology and recommendations with critical review online and in person from research and patient/policy community members. By aligning the DQ technical community and DQ consumers with a common terminology and common expectations for DQ reporting, this initial work provides a common landscape to understand what DQ features have been explored and are being reported. To date, we have assembled a team of leading DQA researchers who developed DQ harmonized terms and reporting recommendations that have been improved and revised through multiple rounds of critical review by research and patient/policy community members. The project also developed a CDM for storing DQ summary statistics (eg, counts of missing data by variable, average age of patients, distribution and density of recorded patient variables over time) (DQA-CDM) that are independent of the organization of a particular data set. During the last 3 months of the project, the team received stakeholder review and guidance on what features would make the prototype DQ visualizations usable for any data set and understandable for any data owner or data consumer. Recommendations regarding the visualization prototypes among researchers and stakeholders included incorporating DQ gold standards for comparison to the user's data set, enable utilizing the user's own data to develop their own relevant comparators, and making customization of the dashboard and data presentations available to fit user needs.
Definitions of Specialized Terms and Abbreviations
Specialized Terminology and Abbreviations
Term | Definition |
---|---|
AHRQ | Agency for Healthcare Research and Quality: A federal agency charged with improving the safety and quality of America's health care system. See https://www.ahrq.gov/cpi/about/profile/index.html |
CER | Comparative effectiveness research: Informs health care decisions by comparing drugs, devices, tests, or ways to deliver health care and providing evidence on the effectiveness, benefits, and harms of different treatment options |
DQ | Data quality: A marker of data fitness to serve its purpose within a given context |
DQ CDM | Data quality common data model: A general model designed to store data summary statistics that can support DQ assessments |
DQA CDM | Data quality assessment: Statistical evaluation of whether data meet the quality required to support their intended use |
EDM Forum | Evidence, Data & Methods Forum: A collaborative within AcademyHealth established to advance the national dialogue on the use of electronic health data for research and quality improvement. See https://www.academyhealth.org/about/programs/electronic-data-methods-edm-forum |
EHR | Electronic health record |
EQUATOR Network | Enhancing the QUAlity and Transparency Of health Research Network: An international initiative aimed at promoting transparent and accurate reporting of health research studies to enhance the value and reliability of medical research literature. See http://www.equator-network.org |
GROUCH | Generalized Review of OSCAR Unified Checking: A program that contains a set of DQ rules that produces a summary report for warnings of implausible and suspicious data observed from the OSCAR summary (see OSCAR below). See omop.org/GROUCH. NOTE: OMOP is no longer active and has been superseded by OHDSI. |
Mini-Sentinel | A pilot program sponsored by the US Food and Drug Administration (FDA) to inform and facilitate development of a fully operational active surveillance system for monitoring the safety of FDA-regulated medical products. See www.sentinelinitiative.org |
OHDSI | Observational Health Data Sciences and Informatics: An interdisciplinary international collaborative formed to generate evidence that promotes better health decisions and care through large-scale analytics. See http://www.ohdsi.org |
OMOP | Observational Medical Outcomes Partnership: A public–private partnership established to inform the appropriate use of observational health care databases for studying the effects of medical products. See omop.org. NOTE: OMOP is no longer active and has been superseded by OHDSI. |
OSCAR | Observational Source Characteristics Analysis Report: A program that creates structured output of descriptive statistics for all relevant tables within the OMOP CDM to allow interpretation of DQ. See omop.org/OSCAR. NOTE: OMOP is no longer active and has been superseded by OHDSI. |
PCOR | Patient-centered outcomes research |
PCORnet | A national network that collects hospital and clinic data stakeholders can use to conduct clinical research and help make informed health care decisions. See www.pcornet.org |
PICOTS | A format for formulating research questions by identifying the relevant population, intervention, comparison, outcome, time frame, and setting |
STROBE | STrengthening the Reporting of OBservational studies in Epidemiology: A set of reporting recommendations for transparent reporting of DQ in epidemiology. See https://strobe-statement.org/index.php?id=strobe-home |
References
- 1.
- Safran C, Bloomrosen M, Hammond WE, et al. Toward a national framework for the secondary use of health data: an American Medical Informatics Association white paper. J Am Med Inform Assoc. 2007;14(1):1-9. [PMC free article: PMC2329823] [PubMed: 17077452]
- 2.
- Hersh WR. Adding value to the electronic health record through secondary use of data for quality assurance, research, and surveillance. Am J Manag Care. 2007;13(6 Pt 1):277-278. [PubMed: 17567224]
- 3.
- Sandhu E, Weinstein S, McKethan A, Jain SH. Secondary uses of electronic health record data: benefits and barriers. Jt Comm J Qual Patient Saf. 2012;(38):34-40, 1. [PubMed: 22324189]
- 4.
- Weber GM. Identifying translational science within the triangle of biomedicine. J Transl Med. 2013;(11):126. [PMC free article: PMC3666890] [PubMed: 23705970]
- 5.
- Dean BB, Lam J, Natoli JL, Butler Q, Aguilar D, Nordyke RJ. Review: use of electronic medical records for health outcomes research: a literature review. Med Care Res Rev. 2009;66(6):611-638. [PubMed: 19279318]
- 6.
- Roth C, Shivade CP, Foraker RE, Embi PJ. Integrating population-and patient-level data for secondary use of electronic health records to study overweight and obesity. Stud Health Technol Inform. 2013;(192):1100. [PubMed: 23920874]
- 7.
- De Moor G, Sundgren M, Kalra D, et al. Using electronic health records for clinical research: the case of the EHR4CR project. J Biomed Inform. 2015;(53):162-173. [PubMed: 25463966]
- 8.
- Immanuel V, Johnson K, Young B, Hart G. Testimony on secondary uses of health data to the National Committee on Vital and Health Statistics. US Department of Health and Human Services; 2007.
- 9.
- Murphy SN, Weber G, Mendis M, et al. Serving the enterprise and beyond with informatics for integrating biology and the bedside (i2b2). J Am Med Inform Assoc. 2010;17(2):124-130. [PMC free article: PMC3000779] [PubMed: 20190053]
- 10.
- Chute CG, Pathak J, Savova GK, et al. The SHARPn project on secondary use of electronic medical record data: progress, plans, and possibilities. AMIA Annu Symp Proc. 2011;(2011):248-256. [PMC free article: PMC3243296] [PubMed: 22195076]
- 11.
- Holzer K, Gall W. Utilizing IHE-based electronic health record systems for secondary use. Methods Inf Med. 2011;(50):319-325. [PubMed: 21431246]
- 12.
- Johnson EK, Broder-Fingert S, Tanpowpong P, Bickel J, Lightdale JR, Nelson CP. Use of the i2b2 research query tool to conduct a matched case–control clinical research study: advantages, disadvantages and methodological considerations. BMC Med Res Methodol. 2014;(14):16. [PMC free article: PMC3909388] [PubMed: 24479726]
- 13.
- Pace WD, Cifuentes M, Valuck RJ, Staton EW, Brandt EC, West DR. An electronic practice-based network for observational comparative effectiveness research. Ann Intern Med. 2009;151(5):338-340. [PubMed: 19638402]
- 14.
- Behrman RE, Benner JS, Brown JS, McClellan M, Woodcock J, Platt R. Developing the Sentinel system—a national resource for evidence development. N Engl J Med. 2011;364(6):498-499. [PubMed: 21226658]
- 15.
- Helmer KG, Ambite JL, Ames J, et al. Enabling collaborative research using the Biomedical Informatics Research Network (BIRN). J Am Med Inform Assoc. 2011;(18):416-422. [PMC free article: PMC3128398] [PubMed: 21515543]
- 16.
- McMurry AJ, Murphy SN, MacFadden D, et al. SHRINE: enabling nationally scalable multi-site disease studies. PLoS One. 2013;8(3):e55811. doi:10.1371/journal.pone.0055811 [PMC free article: PMC3591385] [PubMed: 23533569] [CrossRef]
- 17.
- Fleurence RL, Curtis LH, Califf RM, Platt R, Selby JV, Brown JS. Launching PCORnet, a national patient-centered clinical research network. J Am Med Inform Assoc. 2014;(4):578-582. [PMC free article: PMC4078292] [PubMed: 24821743]
- 18.
- Ross TR, Ng D, Brown JS, et al. The HMO Research Network Virtual Data Warehouse: a public data model to support collaboration. EGEMS (Wash DC). 2014;2(1):2. http://doi
.org/10.13063/2327-9214.1049 [PMC free article: PMC4371424] [PubMed: 25848584] - 19.
- Hripcsak G, Duke JD, Shah NH, et al. Observational Health Data Sciences and Informatics (OHDSI): opportunities for observational researchers. Stud Health Technol Inform. 2015;(216):574-578. [PMC free article: PMC4815923] [PubMed: 26262116]
- 20.
- NIH Collaboratory Health Care Systems Research Collaboratory home page. Accessed June 8, 2016. https://www.nihcollaboratory.org/about-us/Pages/default.aspx
- 21.
- Hogan WR, Wagner MM. Accuracy of data in computer-based patient records. J Am Med Inform Assoc. 1997;4(5):342-355. [PMC free article: PMC61252] [PubMed: 9292840]
- 22.
- Aronsky D, Haug PJ. Assessing the quality of clinical data in a computer-based record for calculating the pneumonia severity index. J Am Med Inform Assoc. 2000;7(1):55-65. [PMC free article: PMC61455] [PubMed: 10641963]
- 23.
- Arts D, de Keizer N, Scheffer G-J, de Jonge E. Quality of data collected for severity of illness scores in the Dutch National Intensive Care Evaluation (NICE) registry. Intensive Care Med. 2002;28(5):656-659. [PubMed: 12029418]
- 24.
- Thiru K, Hassey A, Sullivan F. Systematic review of scope and quality of electronic patient record data in primary care. BMJ. 2003;326(7398):1070. [PMC free article: PMC155692] [PubMed: 12750210]
- 25.
- Hasan S, Padman R. Analyzing the effect of data quality on the accuracy of clinical decision support systems: a computer simulation approach. AMIA Annu Symp Proc. 2006;324-328. [PMC free article: PMC1839724] [PubMed: 17238356]
- 26.
- Cruz-Correia RJ, Rodrigues P, Freitas A, Almeida FC, Chen R, Costa-Pereira A. Data quality and integration issues in electronic health records. In: Hristidis V, ed. Information Discovery on Electronic Health Records. Chapman and Hall/CRC; 2009:55-95.
- 27.
- Botsis T, Hartvigsen G, Chen F, Weng C. Secondary use of EHR: data quality issues and informatics opportunities. AMIA Jt Summits Transl Sci Proc. 2010;(2010):1-5. [PMC free article: PMC3041534] [PubMed: 21347133]
- 28.
- Hripcsak G, Knirsch C, Zhou L, Wilcox A, Melton G. Bias associated with mining electronic health records. J Biomed Discov Collab. 2011;(6):48-52. [PMC free article: PMC3149555] [PubMed: 21647858]
- 29.
- Brown JS, Kahn M, Toh S. Data quality assessment for comparative effectiveness research in distributed data networks. Med Care. 2013;(51):S22-S29. [PMC free article: PMC4306391] [PubMed: 23793049]
- 30.
- Rusanov A, Weiskopf NG, Wang S, Weng C. Hidden in plain sight: bias towards sick patients when sampling patients with sufficient electronic health record data for research. BMC Med Inform Decis Mak. 2014;(14):51. [PMC free article: PMC4062889] [PubMed: 24916006]
- 31.
- Kahn MG, Ranade D. The impact of electronic medical records data sources on an adverse drug event quality measure. J Am Med Inform Assoc. 2010;17(2):185-191. [PMC free article: PMC3000787] [PubMed: 20190062]
- 32.
- Chan KS, Fowles JB, Weiner JP. Electronic health records and reliability and validity of quality measures: a review of the literature. Med Care Res Rev. 2010;(67):503-527. [PubMed: 20150441]
- 33.
- Parsons A, McCullough C, Wang J, Shih S. Validity of electronic health record-derived quality measurement for performance monitoring. J Am Med Inform Assoc. 2012;19(4):604-609. [PMC free article: PMC3384112] [PubMed: 22249967]
- 34.
- Brown JS, Chun A, Davidson BN, et al. Recommendations for transparent reporting of data quality assessment results for observational healthcare data. EGEMs Gener Evid Methods Improve Patient Outcomes. 2015 accepted for publication.
- 35.
- Simera I, Altman DG, Moher D, Schulz KF, Hoey J. Guidelines for reporting health research: the EQUATOR network's survey of guideline authors. PLoS Med. 2008;5(6):e139. doi:10.1371/journal.pmed.0050139 [PMC free article: PMC2443184] [PubMed: 18578566] [CrossRef]
- 36.
- The EQUATOR Network—Enhancing the QUAlity and Transparency Of Health Research website. Accessed October 21, 2013. http://www
.equator-network.org - 37.
- Kahn MG, Callahan TJ, Barnard J, et al. A harmonized data quality assessment terminology and framework for the secondary use of electronic health record data. EGEMS (Wash DC). 2016;4(1):1244. [PMC free article: PMC5051581] [PubMed: 27713905]
- 38.
- Kahn MG, Brown J, Chun A, et al. Transparent reporting of data quality in distributed data networks. EGEMs Gener Evid Methods Improve Patient Outcomes. 2015 Mar 23;3(1). http://repository
.academyhealth .org/egems/vol3/iss1/7 [PMC free article: PMC4434997] [PubMed: 25992385] - 39.
- Mini-Sentinel standard operating procedure: data quality checking and profiling. Mini-Sentinel Coordinating Center. Accessed April 1, 2013. http://www.mini-sentinel.org/work_products/About_Us/Mini-Sentinel_SOP_Data-Quality-Checking-and-Profiling.pdf
- 40.
- Observational Medical Outcomes Partnership. OSCAR–Observational Source Characteristics Analysis Report (OSCAR) design specification and feasibility assessment. 2011. Accessed April 1, 2013.
- 41.
- Observational Medical Outcomes Partnership. Generalized Review of OSCAR Unified Checking. 2011. Accessed April 1, 2013.
- 42.
- The CIHI Data Quality Framework. Canadian Institute for Health Information; 2009. https://www.cihi.ca/en/document/the-cihi-data-quality-framework-2009
- 43.
- Redman TC. Data Quality: The Field Guide. Digital Press; 2001.
- 44.
- Nahm M. Data quality in clinical research. In: Clinical Research Informatics. Springer-Verlag; 2012:175-201.
- 45.
- Maydanchik A. Data Quality Assessment. Technics Publications; 2007. xiv. Data Quality for Practitioners Series.
- 46.
- Magnusson D, Bergman LR, European Network on Longitudinal Studies on Individual Development. Data Quality in Longitudinal Research. Cambridge University Press; 1990.
- 47.
- Singh S. Evaluation of Data Quality. International Statistical Institute by Oxford University Press; 1987:618-643.
- 48.
- Sadiq S, ed. Handbook of Data Quality. Springer Berlin Heidelberg; 2013.
- 49.
- Callahan TJ, Barnard JG, Helmkamp LJ, Maertens JA, Kahn MG. Reporting data quality assessment results: identifying individual and organizational barriers and solutions. EGEMS (Wash DC). 2017;5(1):16. [PMC free article: PMC5982990] [PubMed: 29881736]
- 50.
- Graneheim UH, Lundman B. Qualitative content analysis in nursing research: concepts, procedures and measures to achieve trustworthiness. Nurse Educ Today. 2004;24(2):105-112. [PubMed: 14769454]
- 51.
- Mauthner NS, Doucet A. Reflexive accounts and accounts of reflexivity in qualitative data analysis. Sociology. 2003;37(3):413-431.
- 52.
- Sebastian-Coleman L. Measuring Data Quality for Ongoing Improvement: A Data Quality Assessment Framework. 1st ed. Morgan Kaufmann; 2013.
- 53.
- Khan M. An empirical study of barriers in implementing total quality management in service organizations in Pakistan. Asian J Bus Manag Stud. 2011;2(4):155-161.
- 54.
- Dillman DA. Mail and Internet Surveys: The Tailored Design Method—2007 Update With New Internet, Visual, and Mixed-Mode Guide. John Wiley & Sons; 2011.
- 55.
- Harris PA, Taylor R, Thielke R, Payne J, Gonzalez N, Conde JG. Research electronic data capture (REDCap)—a metadata-driven methodology and workflow process for providing translational research informatics support. J Biomed Inform. 2009;42(2):377-381. [PMC free article: PMC2700030] [PubMed: 18929686]
- 56.
- Callahan TJ, Bauck AE, Bertoch D, et al. A comparison of data quality assessment checks in six data sharing networks. EGEMS (Wash DC). 2017;5(1):8. [PMC free article: PMC5982846] [PubMed: 29881733]
- 57.
- Center for Effectiveness & Safety Research website. Accessed August 24, 2016. http://cesr.kp.org/en/
- 58.
- Ball R, Robb M, Anderson S, Dal Pan G. The FDA's sentinel initiative—a comprehensive approach to medical product surveillance. Clin Pharmacol Ther. 2016;99(3):265-268. [PubMed: 26667601]
- 59.
- Utidjian LH, Khare R, Burrows E, Schulte G. Identifying and understanding data quality issues in a pediatric distributed research network. In: 2015 AAP National Conference and Exhibition. American Academy of Pediatrics; October 24-27, 2015; Washington, DC. Accessed August 24, 2016. https://aap.confex.com/aap/2015/webprogrampress/Paper30131.html
- 60.
- Children's Hospital Association. Pediatric Health Information System website. Accessed August 24, 2016. https://www
.childrenshospitals.org/phis - 61.
- Bhattacharya S, Dunham AA, Cornish MA, et al. The Measurement to Understand Reclassification of Disease of Cabarrus/Kannapolis (MURDOCK) study community registry and biorepository. Am J Transl Res. 2012;4(4):458-470. [PMC free article: PMC3493022] [PubMed: 23145214]
- 62.
- Overhage JM, Ryan PB, Reich CG, Hartzema AG, Stang PE. Validation of a common data model for active safety surveillance research. J Am Med Inform Assoc. 2012;19(1):54-60. [PMC free article: PMC3240764] [PubMed: 22037893]
- 63.
- von Elm E, Altman DG, Egger M, Pocock SJ, Gøtzsche PC, Vandenbroucke JP. The Strengthening the Reporting of Observational Studies in Epidemiology (STROBE) statement: guidelines for reporting observational studies. J Clin Epidemiol. 2008;61(4):344-349. [PubMed: 18313558]
- 64.
- STROBE Statement: Home website. Accessed July 23, 2017. https:
//strobe-statement .org/index.php?id=strobe-home - 65.
- Mackay EA. Patients, consumers, and caregivers: the original data stewards. EGEMS (Wash DC). 2015;3(1):1173. [PMC free article: PMC4435025] [PubMed: 25992390]
- 66.
- Prodinger B, Tennant A, Stucki G, Cieza A, Üstün TB. Harmonizing routinely collected health information for strengthening quality management in health systems: requirements and practice. J Health Serv Res Policy. 2016;21(4):223-228. [PubMed: 26984788]
- 67.
- Brown JS, Kahn M, Toh S. Data quality assessment for comparative effectiveness research in distributed data networks. Med Care. 2013;51(8 Suppl 3):S22-S29. [PMC free article: PMC4306391] [PubMed: 23793049]
- 68.
- Ross TR, Ng D, Brown JS, et al. The HMO Research Network Virtual Data Warehouse: a public data model to support collaboration. EGEMS (Wash DC). 2014;2(1):1049. [PMC free article: PMC4371424] [PubMed: 25848584]
- 69.
- Linstone HA, Turoff M, eds. The Delphi Method: Techniques and Applications. Addison-Wesley Pub. Co., Advanced Book Program; 1975.
- 70.
- Estiri H, Stephens K. DQe-v: a database-agnostic framework for exploring variability in electronic health record data across time and site location. EGEMS (Wash DC). 2017;5(1):3. [PMC free article: PMC5994933] [PubMed: 29930954]
- 71.
- Zozus MN, Hammond WE, Green BB, et al. Assessing data quality for healthcare systems data used in clinical research (Version 1.0). Accessed September 13, 2014. http://sites
.duke.edu /rethinkingclinicaltrials /assessing-data-quality - 72.
- Dziadkowiec O, Callahan T, Ozkaynak M, Reeder B, Welton J. Using a data quality framework to clean data extracted from the electronic health record: a case study. EGEMS (Wash DC). 2016;4(1):1201. [PMC free article: PMC4933574] [PubMed: 27429992]
- 73.
- Khare R, Utidjian L, Ruth BJ, et al. A longitudinal analysis of data quality in a large pediatric data research network. J Am Med Inform Assoc. April 8, 2017. Accessed April 26, 2017. https://academic
.oup .com/jamia/article-lookup/doi/10 .1093/jamia/ocx033 [PMC free article: PMC6259665] [PubMed: 28398525] - 74.
- Raths D. Trend: standards development. Catching FHIR. A new HL7 draft standard may boost web services development in healthcare. Healthc Inform. 2014;31(2):13, 16. [PubMed: 24812983]
- 75.
- Health Level Seven International. HL7 launches Argonaut Project to advance FHIR interoperability standard. Health Manag Technol. 2015;36(2):26. [PubMed: 26211233]
- 76.
- National Library of Medicine. Common Data Element (CDE) resource portal. 2013. Accessed February 16, 2014. http://www
.nlm.nih.gov/cde - 77.
- BioCADDIE—biomedical and healthCAre Data Discovery and Indexing Ecosystem. Accessed October 29, 2016. https://biocaddie.org
- 78.
- Ohno-Machado L, Alter G, Fore I, Martone M, Sansone SA, Xu H. Biocaddie white paper—data discovery index. White Pap BioCADDIE. 2015. https://biocaddie.org/publications/biocaddie-white-paper
Publications
Acknowledgment
Research reported in this report was [partially] funded through a Patient-Centered Outcomes Research Institute® (PCORI®) Award (ME-1303-5581). Further information available at: https://www.pcori.org/research-results/2013/developing-standards-improving-measurement-and-reporting-data-quality-health-research
Suggested citation:
Kahn M, Ong T, Barnard J, Maertens J. (2018). Developing Standards for Improving Measurement and Reporting of Data Quality in Health Research. Patient-Centered Outcomes Research Institute (PCORI). https://doi.org/10.25302/3.2018.ME.13035581
Disclaimer
The [views, statements, opinions] presented in this report are solely the responsibility of the author(s) and do not necessarily represent the views of the Patient-Centered Outcomes Research Institute® (PCORI®), its Board of Governors or Methodology Committee.
- NLM CatalogRelated NLM Catalog Entries
- PMCPubMed Central citations
- PubMedLinks to PubMed
- Developing Standards for Improving Measurement and Reporting of Data Quality in ...Developing Standards for Improving Measurement and Reporting of Data Quality in Health Research
Your browsing activity is empty.
Activity recording is turned off.
See more...