NCBI Bookshelf. A service of the National Library of Medicine, National Institutes of Health.
National Academies of Sciences, Engineering, and Medicine; Health and Medicine Division; Board on Health Care Services; Alper J, Spicer CM, Applegate A, editors. Health Disparities in the Medical Record and Disability Determinations: Proceedings of a Workshop. Washington (DC): National Academies Press (US); 2024 Sep 20.
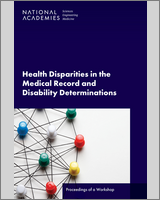
Health Disparities in the Medical Record and Disability Determinations: Proceedings of a Workshop.
Show detailsKey Messages from Individual Speakers
- With electronic health record–based (EHR) tools, good, complete, and expressive notes and structured data can improve care quality and efficiency. (Rosenbloom)
- The digital divide leads to interventions that are not effective or that do not reach all populations equally, further exacerbating health inequities. (Del Fiol)
- Policy is an important driver of data collection. Mandating that health systems capture social determinants of health will drive a high level of data completeness in the appropriate fields in the EHR. (Adler-Milstein)
- Machine learning models, and specifically deep neural network models trained using annotated text, can identify social determinants of health from free text in EHRs. (Vydiswaran)
- The gold standard for information about an individual is what the individual says about themselves. (Adler-Milstein, Kawamoto)
The second day of the workshop opened with a panel exploring issues regarding how the electronic health record (EHR) affects disability determinations. The four speakers for this panel were S. Trent Rosenbloom, vice chair for faculty affairs and professor of biomedical informatics at Vanderbilt University Medical Center and director of the MyHealth at Vanderbilt patient portal, who discussed the basics of EHR documentation; Guilherme Del Fiol, professor and vice chair for research in the University of Utah’s Department of Biomedical Informatics, who discussed the challenge of bias in EHRs and clinical documentation; Julia Adler-Milstein, professor of medicine, chief of the division of clinical informatics and digital transformation, and director of the Center for Clinical Informatics and Improvement Research at the University of California, San Francisco, who discussed how technology can pull information about social determinants of health from the EHR; and V. G. Vinod Vydiswaran, associate professor of learning health sciences at the University of Michigan Medical School and associate professor of information in the University of Michigan’s School of Information, who spoke about using narrative expressive documentation. Following the four presentations, Kensaku Kawamoto, planning committee member, professor of biomedical informatics and the associate chief medical information officer at the University of Utah, and founding director of ReImagine EHR, moderated a discussion among the panelists.
CURRENT STATE OF CLINICAL DOCUMENTATION IN THE EHR
S. Trent Rosenbloom discussed the basics of how providers produce clinical notes and why. The goal of clinical documentation, he said, is to create a record of observations, impressions, plans, and activities from clinical care, usually tied to specific, billable encounters between patients and their caregivers and clinicians or health care organizations. Clinical documentation can include narrative notes using a standard, structured format about what happened at an encounter and data points, such as laboratory test results. Using computers for clinical documentation dates back to the earliest computers based on punch cards, he added.
The most common way to document an encounter in the EHR uses templates, which are structured forms with space for the clinician to enter information. Templates, said Rosenbloom, can create massive notes that are unreadable. He noted that most patients and their caregivers are storytellers, and the role of the clinician is to capture their patients’ stories and replicate them in a clinical note in the EHR. Since documentation can be burdensome, physicians might shift the job of entering information into the EHR to a nurse, scribe, or medical student. Newer approaches to documenting an encounter include having multiple people collaboratively create a note using a wiki or other technology and having a computer record a clinician–patient encounter and using an artificial intelligence (AI) application to transcribe the recording into the EHR. Optical character recognition on handwritten clinical notes is another method for documenting an encounter in the EHR.
Rosenbloom explained that structured entry approaches make it easy to reuse information and compile information across EHRs, but they can be inefficient and inhibit capturing a complete narrative about an encounter. In contrast, entering a note directly maximizes storytelling and expressivity, but makes it difficult to reuse information.
Clinical documentation, said Rosenbloom, takes time away from interacting with patients, and often, clinicians spend time after work entering information into the EHR. Creating documentation is a burden and is an increasingly recognized contributor to clinician burnout, medical errors, hospital-acquired infections, and decreased clinician and patient satisfaction. Burden, he said, is an imbalance between what a clinician likes to do and what they have to do to get paid and ensure there is a legal record of the care provided. One issue is there is no clear standard for high-quality clinical documentation, and another is that a lack of integration into the workflow can increase documentation burden.
Regarding burden, research has found that outpatient physicians spend 16 minutes per patient interacting with the EHR, with 11 percent of that time spent after hours and on weekends. Nurses, said Rosenbloom, now spend 19 to 35 percent of their shift on documentation, up from 9 percent when medical records were kept on paper, and hospital nurses document an average of one data point every 49 to 88 seconds. While the burden is real, he noted that not all documentation is a burden. With EHR-based tools, good, complete, and expressive notes and structured data can improve care quality and efficiency, he said.
BIASES IN EHR DOCUMENTATION AND ITS EFFECT ON CLINICAL CARE
Guilherme Del Fiol said the widespread adoption of EHR systems is powering data-driven interventions to improve health care delivery, both in terms of clinical decision support and patient engagement. Clinical decision support systems are EHR tools that try to help health care professionals make better decisions and carry out those decisions more efficiently. Patient engagement tools include patient portals, along with emails and text messages that health care systems and providers send. Del Fiol said a substantial body of evidence shows that these tools help improve health care delivery (Bright et al., 2012; Chen et al., 2023; Han et al., 2019), but there is also evidence of a digital divide, at both the clinic and patient levels (Kan et al., 2024; Saeed and Masters, 2021). The digital divide leads to interventions that are not effective or that do not reach all populations equally, further exacerbating health inequities (Boyd et al., 2023a,b).
There has been near-universal adoption of EHRs, largely the result of the Affordable Care Act’s Meaningful Use incentives. However, said Del Fiol, while even low-resource settings are using EHRs, they are less likely to adopt advanced clinical decision support tools and patient engagement functions (Adler-Milstein et al., 2017; Kruse et al., 2016). Low-resource settings also lack the capacity to optimize clinical decision support tools and to establish governance over their use, both critical for optimal functioning and effectiveness (Kawamanto et al., 2018; Wright et al., 2011). These clinics can adopt these tools and use them effectively with technical assistance, such as the assistance provided by the Centers for Disease Control and Prevention’s Colorectal Cancer Prevention Program.1 Under the auspices of this program, Del Fiol’s team is working with 13 low-resource, rural federally qualified health centers (FQHCs) in Utah to help them fine-tune their EHRs so they provide reminders to clinicians that patients are due for colorectal cancer screenings and implement text messaging–based patient reminders. As a result, colorectal cancer screening rates at these FQHCs doubled after implementing these tools.
Del Fiol noted the concept of data poverty and provided a definition:
The inability for individuals, groups, and populations to benefit from digital health advances due to health data disparities, which can perpetuate or amplify existing and known health care disparities affecting marginalized and historically underserved populations. (Ibrahim et al., 2021)
The basic idea is that people who do not have data in an EHR or are underrepresented in the EHR will not benefit from data-driven interventions, which can amplify inequities. The result is two types of bias—representativeness and information presence—with significant downstream effects. Representative bias refers to there being groups disproportionately represented in EHRs, largely because they do not have access to care, so their data will not be in EHRs. For groups that have access to care, there may still be disproportionately less complete or accurate data in EHRs.
As an example, the Broadening the Reach, Impact, and Delivery of Genetic Services (BRIDGE) trial was designed to use family history to tailor prevention strategies for a variety of conditions, including cancer, where 13 percent of the U.S. population is at elevated risk of hereditary cancer (Scheuner et al., 2010). Since most people and providers do not know this, almost everyone who would benefit do not get tested for cancer. The BRIDGE trial, said Del Fiol, used a population-based algorithm to identify eligible patients. He and his collaborators scanned EHRs according to certain rule-based criteria to find eligible people who could benefit from genetic testing and notify them proactively via an automated chatbot, written by health communication experts, to provide educational information about genetic testing and offering them access to genetic testing by clicking “Yes, I would like to get tested.” Those who clicked Yes then received a saliva-collection kit at their home, which they would mail to the laboratory and receive the results.
Del Fiol and his collaborators scanned records for nearly 446,000 individuals seen at the University of Utah and New York University, identifying over 22,000 individuals, or 5 percent of the population screened, who would benefit from direct testing (Kaphingst et al., 2021). The research team recruited approximately 3,000 of these individuals and randomized them to receive standard genetic counseling or receive information from the chatbot. Some 15 percent of the people completed genetic testing, but a secondary analysis of the data found important disparities in family history documentation, or information presence bias (Bradshaw et al., 2024; Chavez-Yenter et al., 2022). “Historically marginalized groups in the trial were about half as likely to have family history documentation, and therefore, they could not meet the algorithm criteria,” said Del Fiol. “If they did not meet the algorithm criteria, they were not included in the trial and could not benefit from genetic testing.”
The secondary analysis also found representativeness bias. Even for individuals who met the algorithm criteria, those in marginalized groups were less likely to have a patient portal account. “No portal account, you cannot communicate with the health system and you are not in the trial,” said Del Fiol. Even those individuals from marginalized communities who had patient portal accounts were less likely to access their messages, and even if they answered their messages, they were less likely to use the chatbot. “It requires some digital literacy and, at the end, the downstream effect, they do not have the benefit of genetic testing,” he explained.
Digital exclusion, said Del Fiol, is a “super” social determinant of health (Sieck et al., 2021), given that people need digital technology to access health care, find resources in the community, buy food, get transportation, and obtain an education (Figure 7-1). “Being excluded from the digital environment contributes to health disparities,” he said.
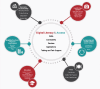
FIGURE 7-1
Digital inclusion plays a role in many social determinants of health. SOURCES: Del Fiol presentation, April 5, 2024; Sieck et al., 2021. https://doi.org/10.1038/s41746-021-00413-8. CC BY 4.0.
To reduce inequities, Del Fiol said it is important to think carefully about the design of digital interventions so they increase inclusion rather than exclusion. One step would be to ask patients when they come to the clinic if they have access to digital technology and the patient portal. For those who do not, digital navigators—with training, community health workers could serve this role—could help those individuals. Another idea would be to have proactive patient outreach and connection with services via patient portals, text messaging, and chatbots for those who are connected digitally. Today, 97 percent of people in the United States have access to phones with text-messaging capability.
CAPTURING SOCIAL DETERMINANTS WITH HEALTH INFORMATION TECHNOLOGY
Julia Adler-Milstein said EHRs have become somewhat of a Frankenstein tool, where they have been adapted to serve different purposes for different contexts. It is hard to say confidently that every EHR will have a particular type of data.
In 2014, the Institute of Medicine released two reports that pushed the need for EHRs to capture social and behavioral determinants given how important those are to understanding an individual’s health and identifying optimal treatments (Institute of Medicine, 2014a,b).2 In 2016, the 21st Century Cures Act contained a set of policies that pushed for those data to be readily exported and shared from EHRs via interoperability and data standards. The U.S. Core Data Set for Interoperability is the standardized set of health data classes and constituent data elements for nationwide, interoperable health information exchange. While this is the standard, the problem is that the data that are actually available depends on what local health systems are capturing.
A 2019 national survey of ambulatory physicians found that 76 percent were aware their EHR could record social determinants of health data, while 12 percent did not think their EHR could do that and another 12 percent were unsure (Iott et al., 2022). A subsequent 2022 survey found that 81 percent of the physicians were documenting social determinants in their clinical notes in free text form, 61 percent were also documenting social determinants in some structured data field, which could be either a checkbox or a button, and 46 percent said they were using diagnostic codes for social determinants of health (Iott et al., 2023). For family medicine physicians, 61 percent said they documented social determinants in their clinical notes, while 52 percent also documented them via structured data fields.
Adler-Milstein and her collaborators collected similar data from hospitals, finding that 83 percent were collecting data on patient health-related social needs and 54 percent were doing so routinely (Chang and Richwine, 2023). What this means is the data would be available, but in a variety of places in the EHRs.
When Adler-Milstein and her colleagues did a deep dive into their own institution’s EHR, they found that inpatient nursing questions contained the most data on social determinants of health (Iott et al., 2024), largely because California passed a regulation that nurses had to document a person’s housing status for every admission. “One key takeaway is that policy is an important driver. If you are mandated to capture social determinants of health data, you will probably see high completeness of data in that field,” said Adler-Milstein.
About half of the patient EHRs examined had social history text, and about half had social determinants data in social work notes. To determine the accuracy and completeness of these data and the extent to which the documentation represents the true prevalence of social risk, Adler-Milstein and her colleagues surveyed patients and asked them directly about their social determinants of health and compared that to what they found in EHR data (Figure 7-2). The results showed there was a vast gap between the level of social determinants and needs that patients reported and what was in the EHR.

FIGURE 7-2
The incongruence of patient-reported data and EHR data on social determinants of health. NOTES: Dark blue = survey report needed; gray = clinical note documentation during enrollment visit; light blue = both ICD-10 Z Code and note documentation during (more...)
Efforts to use geocoding and various measures such as the Social Vulnerability Index, Area Deprivation Index, and neighborhood stress score as proxies for social determinants that could supplement the EHR from a patient’s zip code found important limitations. One study of over 35,000 patients from a large network of safety net clinics found that almost 30 percent of their populations screened positive for one or more social risks, but 42 percent of the patients with at least one social risk lived in a neighborhood that was not defined as being disadvantaged (Cottrell et al., 2020). Another study of a Medicare Advantage population found the agreement between area-level and individual-level social risk ranged from 53 percent to 77 percent (Brown et al., 2023). “It is probably better to use these proxies, but it is definitely not going to solve the whole problem,” said Alder-Milstein.
Regarding the quality of social determinants of health data in EHRs, she pointed to a 2022 systematic review that looked at this very issue (Cook et al., 2021). In the 76 studies reviewed, the most common issues were completeness and plausibility—are the data values believable and accurate—for individual-level data.
In summary, the data show there is high awareness that EHRs can capture social determinants data, and the reported use is high across all potential methods of documentation. However, there are many methods used to collect these data, and only about one-third of those data are documented using structured diagnostic codes. Regarding actual use of these data, the evidence is limited. At her health system, the levels of documentation were low unless mandated, in which case it was high, or via free text, in which case the level of documentation was moderate. Completeness and plausibility shortcomings reflect on the quality of the data, with the levels of social determinants documentation dramatically underrepresenting self-reported levels and area-level proxy measures, producing noisy data.
As a final comment, Adler-Milstein said there are a great deal of data available on the social determinants of health. However, while that is a good starting point, she also said,
I do not think we can move forward confidently, especially when we are at the point of thinking about eligibility for services, to say these are going to be a robust source of data to tell us who really is or is not eligible for different types of services based on social determinants.
THE IMPORTANCE OF FREE TEXT IN EHRs AND THE ROLE OF ARTIFICIAL INTELLIGENCE
V. G. Vinod Vydiswaran explained that data in EHRs come in two forms: structured in defined fields and unstructured, which are data available in notes and radiology reports, for example. Unstructured data are not readily searchable or available for downstream decision-making tasks. He noted, too, the abundance of text data in health, whether as books and the peer-reviewed literature, paper-based medical records, or prescriptions, that may not find its way into the EHR. Medical natural language processing is an area of AI that synthesizes information from a variety of unstructured data sources to generate insights, such as whether a treatment worked or not.
Vydiswaran discussed how natural language processing can identify cohorts from the narrative data in EHRs by identifying patients who meet certain selection criteria. For example, a search of text data in an EHR might identify men who engage in low-risk alcohol use by spotting text in notes stating that a male patient lives with his wife and drinks two glasses of wine nightly. However, if that same individual were female, they would be in a high risk of alcohol use cohort because of a different defined threshold.
AI-powered models, said Vydiswaran, can identify disease better than just using International Classification of Diseases (ICD) codes. Continuing with the example above, a search of ICD codes in EHRs identified only 29 percent of patients who were high-risk alcohol users. In contrast, natural language processing, by looking for information such as how many drinks a person has or whether they have had a driving while intoxicated citation or come into the emergency department after falling in a bar, identified 87 percent of the patients with risky alcohol behavior (Vydiswaran et al., 2024).
Vydiswaran said that machine learning models, and specifically deep neural network models trained using annotated text, can identify social determinants of health from free text in EHRs (Lybarger et al., 2023a,b). Researchers have also used deep neural network models to identify inequities in telehealth use during the COVID-19 pandemic via EHR analyses of notes providers entered after successful and failed telehealth visits (Buis et al., 2023). Patients who completed telehealth visits were more likely to be younger than 65 years old, female, White, and have no significant comorbidities or disabilities than those who only canceled or missed telehealth appointments. A subsequent analysis identified those patients who had technical difficulties with their telehealth encounter. Individuals whose primary language was Spanish, along with individuals with mobility and vision disabilities, were most likely to experience technical difficulties. Other individuals who were more likely to experience technical difficulties included those who were female, over age 65, Black or African American, or American Indian or Alaska Native, and those with hearing and cognitive disabilities.
The lesson here, said Vydiswaran, is that although EHRs are faulty, incomplete, and biased, machine learning and AI-based natural language processing can pull out information useful for downstream tasks. The positive news is that text is everywhere in health care, providing a valuable source of data that is accessible with the right tools. He reiterated that AI and deep learning methods are more effective than ICD codes alone for phenotyping patient cohorts.
Q&A WITH THE PANELISTS
After summarizing his take-home messages from the presentations, Kensaku Kawamoto commented that as Adler-Milstein noted, the gold standard for self-reported information (e.g., social determinants of health data) about an individual is what the individual says about themselves. Therefore, when applying for a Social Security disability determination, such information should come directly from the patient, not the EHR.
An unidentified workshop participant remarked that the Social Vulnerability Index as a measure of social determinants includes disability status at the county level, while the Area Deprivation Index provides information at the block level but no information about disability. They then asked if pulling data from the Social Vulnerability Index when looking at rural versus urban populations risks doing harm by pulling in data from a broader group that does not get to the needed individualized decisions. Adler-Milstein responded that it is important when trying to make a disability determination to conduct deep investigative work to understand what those sources are capturing. Given there is not a data source that provides the exact information needed in this case, the best approach is to put them together and find consistencies across the data sources that would point to populations more likely to be facing challenges based on where they live. “Our best hope is that we have enough options of different data sources that if we put them together, we can feel more confident in them,” she said. The alternative, she added, is to design and validate a measure specifically fit for this purpose.
Michael V. Stanton asked the panelists to discuss how language can affect inequities. Vydiswaran answered that in his study during the COVID-19 pandemic, there was a technical issue with having three people on a telehealth visit, which were intended to be bidirectional. The solution to this problem lies in scheduling and noting in the EHR that a person has a disability or a need for a medical interpreter, for example, so when the appointment time came, that assistance would be available.
Rosenbloom said the barrier he sees with patient portal access is that they are written for people who speak English. This can create navigational challenges for individuals for whom English is not their native language, and it can make it difficult for the same individuals to access and understand educational materials available through the patient portal. Major EHR vendors have added additional language capabilities to patient portals for navigation purposes, but all information is not available in Arabic or German, for example. Beyond language issues, there are other access issues, such as the need to use one’s Social Security number to access the portal. “If our patient portal requires a Social Security number to access it, you lose a lot of Spanish speakers and you lose a lot of other language speakers who do not have a U.S. Social Security number,” said Rosenbloom. “If you require an email address to access it, you also lose a lot of people who do not use email and use WhatsApp.”
Del Fiol agreed that language is a huge factor in EHR data. His work, for example, found that having a non-English language recorded in the EHR is the strongest predictor for not having a complete family history, or any family history, recorded in the EHR, not having access to the patient portal, and not accessing the chatbot for genetic testing. However, in a study whose goal was to increase uptake of COVID-19 testing by patients, his team used bilingual text messaging to offer to mail test kits to people’s homes. The people who reviewed the grant application were skeptical that people who did not speak English would respond, but the opposite was true. People whose preferred language was Spanish had a higher response rate than those who spoke English. The key is to be intentional about making information accessible.
Elham Mahmoudi, from the University of Michigan, noted that many clinicians are not using social determinants of health information in EHRs because they do not have time. Her institution is having medical social workers look for and act on that information, though her concern is that health care systems are cutting back on their use of medical social workers. Rosenbloom agreed that time pressures play a role, but so does doctors not knowing what to do with social determinants of health information, whereas a medical social worker would.
Tara Lagu said that while asking about the social determinants of health is important, there need to be standardized questions to ask about the social determinants. Otherwise, every health system is asking different questions, making it hard to compare across systems. She has also found that the answers one gets depends on who is asking the question, making the data analysis even more challenging. Adler-Milstein added there is a tension between the call for data standards and pushback from health systems and EHR vendors about having to develop more standards. Adler-Milstein said this is a recognized problem, but she is not sure the solution will come soon.
Rupa Valdez asked the panelists if they see any changes in the data being captured and how it is being captured given that scribes are often used to record data. Rosenbloom replied that scribes are poorly studied and poorly standardized, making it difficult to generalize about what scribes are doing and translate learnings from one setting to another. Another concern with scribes is that they are a crutch that does not address the underlying problems of documentation burden and documentation quality. To him, the solution is to focus on education from the start of medical and nursing school through continuing education and having supports in practice.
Yvonne M. Perret asked if there was any research focusing on FQHCs and social determinants of health. She wants to have a tool that a community health worker could use to get information about social determinants of health and the supports needed to address them. Rosenbloom, who practices at an FQHC, said the challenge is that FQHCs have little money and cannot afford large vendor-based EHR systems, so the EHRs they have are difficult to use and cannot contribute high-quality notes or clinical documentation. These EHRs do not accommodate narrative documentation particularly well, and patients at the FQHC rarely use the patient portal. At the same time, FQHCs have certain reporting standards and therefore have methods and processes for capturing structured information.
Del Fiol said his team held focus groups with community health center clients, and the message was consistent: they are reluctant to disclose problems such as food and housing insecurity at a clinic visit. They are more likely, though, to talk to a community health worker they trust. He also noted that social determinants can change between appointments and that what is recorded in the EHR is just a snapshot of the situation at the time of an appointment. His team is trying to proactively contact patients through low-tech means such as text messaging to ask more often about social needs and to conduct a quick screening with yes-or-no questions about food and housing security, for example. A yes answer would prompt a community health worker to reach out to that individual.
Footnotes
- 1
Available at https://www
.cdc.gov/cancer/crccp/index .htm (accessed April 5, 2024). - 2
As of March 2016, the Health and Medicine Division of the National Academies of Sciences, Engineering, and Medicine continues the consensus studies and convening activities previously carried out by the Institute of Medicine (IOM). The IOM name is used to refer to reports issued prior to July 2015.
- The Health Record in Depth - Health Disparities in the Medical Record and Disabi...The Health Record in Depth - Health Disparities in the Medical Record and Disability Determinations
Your browsing activity is empty.
Activity recording is turned off.
See more...